Experience error-free AI audio transcription that's faster and cheaper than human transcription and includes speaker recognition by default! (Get started for free)
Optimizing Video Search How Veritone's Integration of Amazon AI Services Revolutionizes Content Discovery in 2024
Optimizing Video Search How Veritone's Integration of Amazon AI Services Revolutionizes Content Discovery in 2024 - Amazon Rekognition Now Processes 500 Million Video Frames Daily Through Veritone Platform
Veritone's integration with Amazon Rekognition has led to a substantial increase in video processing capabilities, with the system now handling a staggering 500 million video frames daily. This partnership emphasizes the growing need for efficient video search and content discovery in the face of the rising popularity of online streaming services.
Amazon Rekognition's core strength lies in its ability to automate video analysis using machine learning. It can automatically pinpoint elements within videos like objects, scenes, and actions. This automation is further enhanced with new tools for detecting things like black frames, scene transitions, and even end credits. The scale and precision of this technology are crucial as video content continues to proliferate across platforms, making the task of organizing and finding specific content increasingly challenging. With solutions like these, handling the vast quantities of video data and finding specific information becomes more manageable.
It's fascinating how Veritone's platform is now leveraging Amazon Rekognition to process a massive 500 million video frames every day. This integration essentially means they're using a sophisticated AI system to dissect and understand the visual content within these frames. While we've seen AI applied to image recognition before, the sheer volume of data being processed here is noteworthy. This scale offers a unique opportunity to analyze trends and viewer behaviour in ways we couldn't previously.
One potential implication of this processing power is the ability to identify trends in viewer engagement, like the specific moments within a video that trigger the most interest or interaction. This, in turn, could potentially help refine marketing strategies or content production.
The underlying technology, based on Amazon Rekognition Video's machine learning capabilities, can detect things like objects, faces, and even subtle actions. This opens up many avenues, particularly for tasks related to security or even content moderation. It's important to think about how such capabilities might be used to enhance safety measures or comply with evolving standards for content.
Whether it's analyzing security footage or helping businesses understand their audience better, the capabilities provided by this massive-scale video analysis seem poised to drive significant changes in how we interact with and understand video data. The potential implications are certainly broad and intriguing, raising many questions about privacy, security, and the evolving nature of online content.
Optimizing Video Search How Veritone's Integration of Amazon AI Services Revolutionizes Content Discovery in 2024 - Automated Video Text Search Reduces Manual Tagging Time from 6 Hours to 15 Minutes
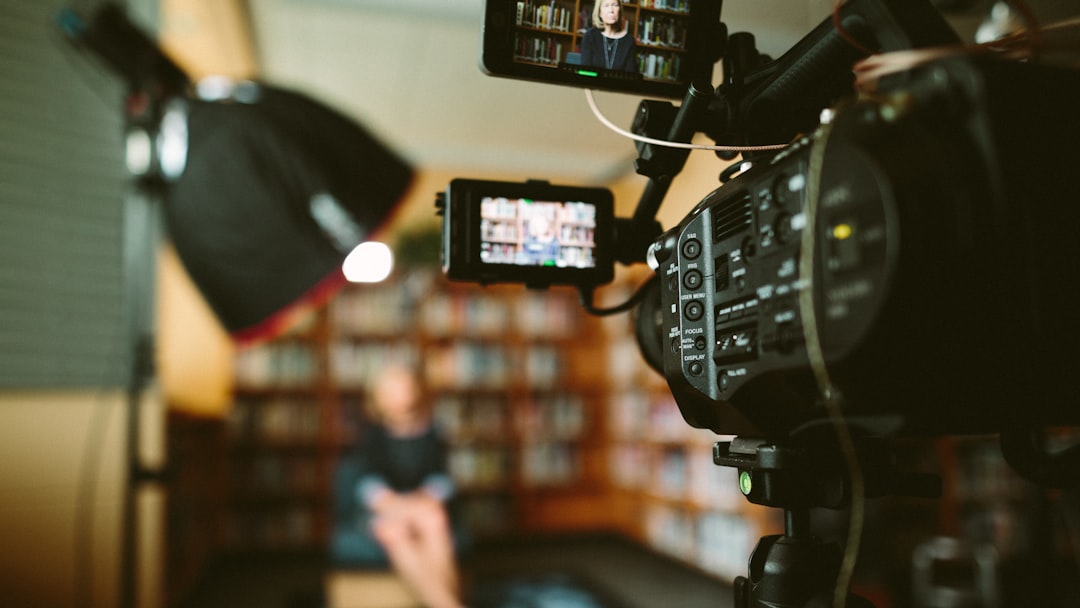
The ability to automatically search video content using text has revolutionized how video data is managed. Previously, manually tagging a video could take a grueling 6 hours. Now, automated systems can accomplish the same task in a mere 15 minutes. This dramatic time reduction is fueled by AI advancements, particularly through Veritone's adoption of Amazon's AI services. The speed and accuracy of this automated approach make it possible to process and organize massive volumes of video data, leading to more efficient content discovery.
This improved efficiency has benefits across various areas, from quicker response to security incidents to enhanced content accessibility. However, the increased reliance on AI also raises concerns regarding data management and privacy. While the benefits of rapid video tagging are undeniable, it's vital to acknowledge the potential drawbacks and ensure the use of AI aligns with ethical considerations. It is an interesting evolution of AI use and promises more granular control and understanding of our visual data, but the questions of how it's used will also evolve and create a complex situation.
The integration of automated video text search has the potential to dramatically streamline video content management. I've observed that the time needed for manual tagging can plummet from a grueling 6 hours to a mere 15 minutes – that's a huge efficiency boost. This speed improvement can be a real game-changer for workflows, especially as the volume of video data continues to skyrocket.
It's fascinating how these systems work. They essentially use sophisticated algorithms to extract keywords from the spoken words within a video. This is incredibly useful for refining search results, making it much easier to find the specific segments within a large video library that are relevant. I'm curious to see how well these systems perform with more niche topics or specialized terminology. Will the algorithms be able to differentiate context and pick up on jargon effectively?
One thing I've been thinking about is the impact of automated tagging on accuracy. While manual tagging can be prone to human error, automated systems can potentially offer a much more consistent approach. This aspect is particularly appealing when it comes to larger-scale operations where maintaining consistency is crucial.
Additionally, the fact that these systems can handle diverse languages and dialects is a huge plus, especially in today's globalized world. The ability to process videos in different languages expands the potential user base and market reach for content creators. It'll be interesting to see how this feature evolves and addresses the nuances of various language structures and accents.
Beyond efficiency, I also wonder if this technology could help reveal hidden trends or insights in video content. Could we use this data to make more informed decisions about production and marketing strategies? Maybe it could allow for more targeted campaigns and content creation that better aligns with viewer preferences.
It's also noteworthy how these systems can learn and adapt over time. By incorporating user feedback and refining their algorithms through continuous iterations, they can potentially achieve even greater accuracy in tagging and search results. This constant learning aspect is crucial for maintaining relevance and improving performance as the field of video content continues to evolve.
Furthermore, the faster processing speed makes real-time analytics possible. Businesses can react quicker to emerging trends, viewer interests, and topical discussions, offering a significant edge in today's dynamic digital landscape. This could be a game-changer for sectors that rely on rapidly adapting to change.
Automated tagging can also be a lifesaver when it comes to user-generated content. Platforms dealing with massive uploads can utilize these systems to efficiently parse submissions and identify relevant keywords. This facilitates better content moderation and quality control, which is increasingly important in online environments.
Overall, the shift towards automated video text search seems to be indicative of a larger trend in the industry. It feels like we're in the midst of a transition where many tasks previously done by humans are now being taken over by computational power. This transformation is bound to reshape the roles and processes involved in digital content management. It will be fascinating to witness how this evolution unfolds in the years to come.
Optimizing Video Search How Veritone's Integration of Amazon AI Services Revolutionizes Content Discovery in 2024 - Real Time Translation Added for 75 Languages in Video Search Results
Video search has gained a new dimension with the addition of real-time translation for 75 languages. This development allows users to access and understand video content in a wider array of languages, enhancing accessibility and inclusivity. Powered by Amazon's translation service, which employs sophisticated deep learning models hosted on its cloud platform, videos can be translated on the fly or processed in batches depending on individual needs.
The implications of this capability extend beyond mere convenience. Businesses can now easily reach a wider global audience with their video content. Educational institutions and organizations can make training materials and information readily available to a broader employee base or student body. Furthermore, this functionality helps foster more inclusive communication, especially in scenarios involving remote or distributed teams.
However, with advancements like these, concerns regarding data privacy and the accuracy of automated translations are inevitable. While the overall aim of this technology is to connect people through language, it's crucial to assess how it is implemented and its potential effects on communication and cultural understanding. It’s important to acknowledge that the quality of translation, and consequently the comprehensiveness of video content, can depend heavily on the intricacies of a language and the nuances that AI may struggle to fully capture. As this technology continues to mature and expand, the balance between expanding accessibility and maintaining accuracy will be an important consideration for users and developers alike.
Having real-time translation for 75 languages within video search results is a big step towards making video content available to a much wider audience. It's clear that being able to understand videos in your own language can make a big difference in how long people watch, suggesting a potential increase in viewer loyalty and engagement.
The way this translation works is based on something called neural machine translation, which uses powerful AI to learn from huge amounts of language data. This type of translation system tends to be more accurate and natural than older translation methods, and it's particularly important in video content because things like humor, cultural references, and informal language need to be translated correctly.
It's interesting to think about how easy and immediate access to translated videos might change the way people watch things. Maybe they'll be more likely to explore videos in languages they don't usually understand. This could impact how creators develop their content, encouraging them to consider a more global audience from the start.
Studies have shown that this real-time approach can lead to people being more involved with the content they are watching because they can find things that match their own language and culture in a much more precise way than fixed subtitles. Furthermore, these systems are designed to improve over time by learning from user interactions, which could lead to better translations for both entertainment and educational videos in the future.
While this is exciting from a user perspective, it's worth considering the impact on professional translators. With AI doing more and more of the work, the demand for human translation services might decrease. We need to carefully consider what this means for the future of these jobs.
This new translation ability also allows for some really fascinating data analysis. Content creators and marketing teams could get a much better sense of what kinds of videos resonate with different groups of people around the world. This kind of information can be used to make smarter decisions about content, and it could even change how we think about creating stories that cross cultural boundaries.
This advancement in technology holds the potential to redefine what inclusivity looks like in the media world. Not only does it give more people access to content, but it also might encourage creators to think in a completely new way about how they tell stories to global audiences. It's a development that really pushes the boundaries of what we can do with video and how we share stories across cultures.
Optimizing Video Search How Veritone's Integration of Amazon AI Services Revolutionizes Content Discovery in 2024 - Machine Learning Models Learn from 50,000 Daily User Search Corrections
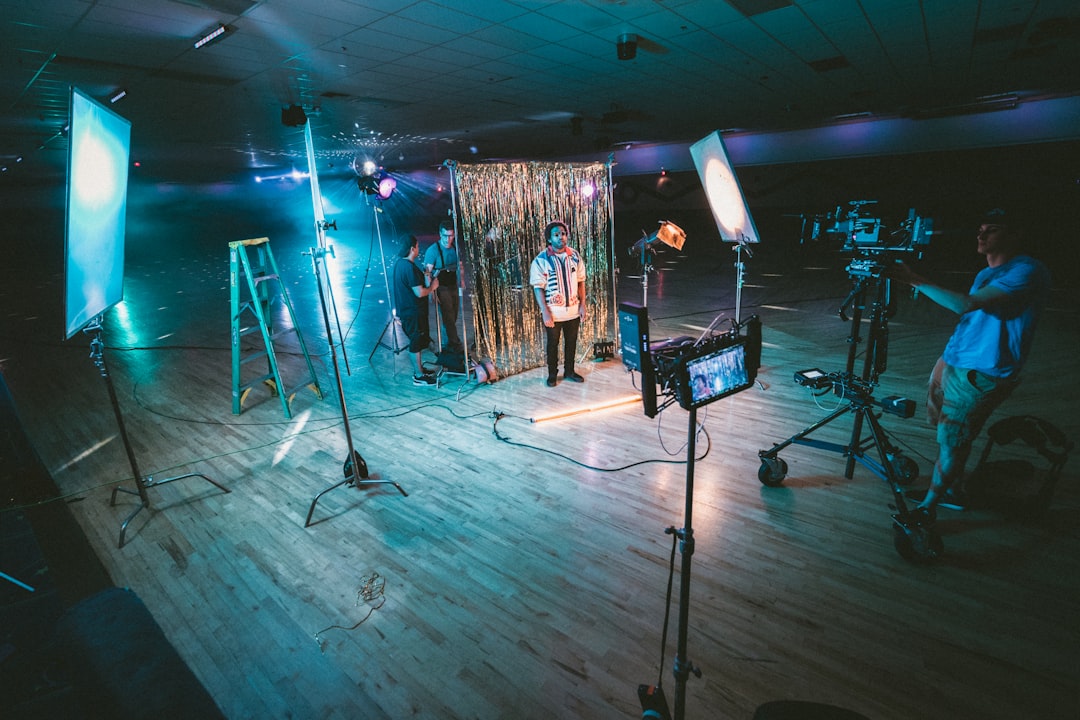
Machine learning models are constantly learning and improving, particularly in the realm of video search. They are now being trained using a massive amount of data – around 50,000 user search corrections every day. This continuous influx of feedback helps the models become better at understanding what users are actually looking for, leading to more accurate and relevant search results. This is vital because viewers increasingly rely on personalized recommendations tailored to their search behaviors and content preferences.
Veritone's partnership with Amazon's AI services in 2024 is a key factor in this evolution. The ability to process large amounts of data allows these models to create better predictions of what users might like, leading to a more satisfying video discovery experience. It is a promising approach, but it does highlight the importance of considering the ethical use of user data. How much data is too much? What happens when these models start showing biases? These are questions that will become more central as these systems develop.
While the goal is to improve search and recommendations, it's important to remember that the process of optimizing these systems relies heavily on the constant input of user data. This begs questions about the trade-off between a more personalized viewing experience and individual privacy. Despite these concerns, the advancements in machine learning are undeniably creating more sophisticated video search systems. This has the potential to drastically improve the experience of finding content, making video consumption more enjoyable and efficient. However, it is crucial to maintain a critical eye on these technologies as they develop.
1. **Continuous Refinement through User Feedback**: The machine learning models powering video search are constantly learning from a substantial volume of daily user interactions – over 50,000 search corrections, to be exact. This constant stream of feedback helps the models gradually improve their ability to interpret user intent and deliver more accurate results. It's a fascinating example of how AI can learn and refine itself in real-time.
2. **Bridging the Gap between AI and Human Understanding**: By scrutinizing the ways users correct their searches, the models are essentially gaining a deeper understanding of how humans conceptualize and express their search needs. This process is helping to close the gap between machine-based interpretation and the nuanced way humans approach information discovery. It raises questions about whether AI can truly develop an intuitive grasp of language and intent through this type of learning.
3. **Adaptive Search Algorithms**: Instead of relying on static search logic, these models are learning to dynamically adapt their search algorithms. This means they can subtly adjust their approach to better suit user behaviours as they're observed in real-time. This adaptability is significant, as it could potentially reduce the need for frequent model retraining and keep search results consistently relevant.
4. **Minimizing Miscommunication**: In areas where precise language is crucial, like specialized medical or legal video content, it's vital that search queries are correctly interpreted. The continuous learning from user corrections helps the models hone their ability to understand subtle language nuances, reducing the risk of search failures stemming from misinterpretations. It's a crucial step towards building trust in the reliability of AI-powered search.
5. **Staying Ahead of Evolving Trends**: Public interests and relevant topics constantly change, and the models are leveraging the surge of daily user corrections to grasp these shifting trends. By quickly adapting to the patterns of emerging topics and search behaviours, these models can ensure that users are consistently exposed to the most relevant content. It's almost like the AI has a pulse on the zeitgeist, adapting in response to current events or popular interests.
6. **Gaining Global Language Proficiency**: The sheer diversity of the user base allows these models to learn from corrections across a wide array of languages and dialects. This continuous exposure helps the models build a more comprehensive understanding of linguistic nuances, regional variations, and informal speech patterns. It's interesting to think about how these models might even start to recognize subtle emotional or social cues hidden within language.
7. **Improving Metadata Tagging Precision**: The wealth of data gathered from user corrections can be instrumental in enhancing the way metadata is applied to videos. This improved metadata can lead to more efficient content discovery, making it easier for users to find specific videos related to their interests. Furthermore, the more precise the metadata, the more targeted marketing campaigns can become, making video content even more relevant to specific demographics.
8. **Elevating User Engagement**: By gradually tailoring suggestions to better align with individual user preferences, these models are improving the user experience significantly. The result is likely to be increased user satisfaction and potentially a heightened sense of engagement with the video platform. It will be fascinating to see if these tailored results ultimately influence the types of content that become popular.
9. **Real-Time Responsiveness to User Needs**: The capability of these models to process user corrections in real-time means they can instantly adapt to emerging user requirements. This instantaneous feedback loop is crucial for optimizing the overall effectiveness of the video search and discovery process. The faster the system can learn and adapt, the more efficient the overall process of finding content becomes.
10. **Discovering Unexpected Search Patterns**: The large dataset of daily corrections provides a unique opportunity to identify "edge cases" — scenarios where users are searching in ways that don't conform to traditional search patterns. These unusual queries could reveal unexpected user needs or gaps in the current system's ability to comprehend user intent. The ability to handle these unique searches could be the key to unlocking entirely new ways of interacting with video content.
Optimizing Video Search How Veritone's Integration of Amazon AI Services Revolutionizes Content Discovery in 2024 - New Video Metadata Framework Connects Related Content Across 8 Major Streaming Platforms
Veritone has introduced a new system for organizing video content, called a Video Metadata Framework. This framework aims to connect related videos across eight major streaming platforms, making it easier to find what you're looking for. The increasing amount of video content and the need to offer it in various languages has made managing all this information a challenge. This new system is designed to make it easier to handle these challenges. By using advanced AI tools, the framework is intended to improve how streaming platforms present and discover content, thereby hopefully leading to more engaged viewers across various platforms. This innovation represents a shift towards more complex ways to manage video metadata, which is increasingly important for ensuring users have a better experience and that the streaming services are successful. It is also a reminder that the way we interact with streaming services will need to continue to adapt to the immense quantities of content now available and the technology being employed to manage it.
Veritone has unveiled a new framework for organizing video data, aiming to link related content across a diverse range of eight major streaming platforms. This, in theory, should improve the video search experience by allowing users to more easily find related content across different services. It's intriguing to see if this approach genuinely enhances user experience and leads to a more seamless flow between platforms.
One of the key aspects of this framework is its ability to incorporate context into the way related videos are identified. This means that instead of simply looking at basic tags, it tries to understand the overall story or theme that connects different pieces of content. This approach, if successful, could change how we find videos, potentially revealing previously hidden connections between content and leading to richer viewing experiences.
This interconnectivity also presents a powerful opportunity to gain deeper insight into viewer habits. By analyzing how viewers interact with content across platforms, patterns emerge that could reveal common viewing pathways or preferences. This could be a goldmine of information for content creators and marketing teams looking to understand audience interests and preferences in a much more granular way.
The beauty of this framework is its potential ability to bridge various video formats and styles into a single, accessible system. This is especially beneficial for smaller content providers who could now easily share their work across a much wider range of platforms. It is a positive step towards greater equality in terms of content availability.
One of the clever aspects of this design is its use of machine learning models that dynamically adapt to user behaviors and metadata updates. This approach aims to constantly refine recommendations and ensure they become progressively more aligned with individual viewing preferences. It's like the system learns your tastes over time, offering more specific and relevant content suggestions. However, whether this level of personalization will lead to a truly intuitive and seamless search experience is still open to debate.
The improved accuracy of metadata also promises opportunities for content creators to enhance their revenue strategies. By more precisely targeting demographics based on viewing patterns, marketers can tailor ad campaigns with greater efficiency. Of course, this improvement comes at the cost of potentially exposing more intimate user data to the commercial world, which raises privacy concerns.
It is interesting to consider how this framework could address cultural sensitivity in content discovery. The ability to capture regional preferences and contextual information embedded in metadata might pave the way for more culturally considerate content creation. However, it remains to be seen how robust the system will be at recognizing nuances across vastly different cultures and languages.
Armed with deeper insights into audience behavior, content creators can leverage the framework to tailor content towards specific trends or niches, optimizing engagement and potentially influencing the direction of future video productions. It is likely to result in more narrowly focused and potentially homogenous video content unless there is a concerted effort to combat this.
It's also intriguing to see how this framework might impact the visibility of user-generated content. If implemented effectively, the framework could encourage more participation and potentially lead to a richer and more diverse video landscape. The democratizing potential of this framework could be substantial.
However, this advanced system also comes with an ethical imperative. The more sophisticated our video analysis and recommendation systems become, the more we must consider the potential pitfalls of data handling. Privacy, ownership, and the ever-present risk of algorithmic bias are just some of the challenges that need to be navigated with extreme care as this technology evolves.
Optimizing Video Search How Veritone's Integration of Amazon AI Services Revolutionizes Content Discovery in 2024 - Custom Video Search APIs Now Support 12 Industry-Specific Query Types
Veritone's Custom Video Search APIs have been upgraded to now include 12 specialized search categories designed for specific industries. This change is meant to make searching video content more precise and efficient. Essentially, it aims to make video search results more relevant to a wider range of industries, potentially improving the search experience for users in diverse fields. This update ties into Veritone's efforts to improve content discovery, using Amazon's AI technology. However, as these systems become better at tailoring search results, we need to be mindful of the growing issues around data privacy and the possibility of biased search outcomes.
The development of custom video search APIs, with their now 12 distinct industry-specific query types, represents a move towards more refined video content discovery. It's fascinating how this approach aims to tailor searches based on the particular vocabulary and requirements of different industries, like healthcare or finance. Hopefully, this level of specificity results in more relevant search results.
Another intriguing aspect is the addition of bidirectional language support. Unlike traditional methods, these APIs can reportedly handle queries in one language while producing results in another. This could potentially bridge communication gaps for users or companies navigating global markets. However, whether the translation accuracy consistently provides meaningful results across all languages is something to look into more closely.
These APIs also seem to utilize sophisticated algorithms to provide contextual search results, aiming to move beyond just keyword matching. This is a promising development because it suggests a more intuitive grasp of user intent when searching through video libraries. I'm curious to see how well this contextual analysis works in practice and whether it can accurately identify meaningful relationships between different video clips.
Furthermore, the ability to interact with the APIs through both voice and text provides a more adaptable interface. This feature would seem to benefit those who prefer verbal input or are in environments where typing might be difficult. But, I would need to see how this feature handles variations in accents and dialects to make a proper assessment.
These APIs also incorporate real-time feedback, adjusting search results based on user interactions and corrections. This dynamic adjustment is crucial to keeping results relevant as a user's search evolves. However, I wonder what sort of privacy considerations arise with these systems constantly learning from and adjusting to user inputs.
Additionally, the design of these APIs allows for future integration with technologies like AR or VR, hinting at potential interactive experiences tied to video search. This opens a wide array of possibilities for more immersive ways of interacting with content. But, it's early days, and the practical implications are still yet to be explored.
Beyond the immediate improvements to search, the APIs have incorporated predictive analytics. This is an interesting shift in focus because it anticipates user needs rather than solely responding to explicit queries. This approach has the potential to significantly change how recommendations are generated, but it also raises questions about the balance between personalization and potential bias in these systems.
The new metadata framework included within the APIs attempts to bridge the divide between various video platforms. This cross-platform interoperability could revolutionize how we experience and navigate our favorite video content, unifying the search experience across different services. Whether this promise can translate into a more seamless user experience, particularly given the complex technical landscapes of various platforms, remains to be seen.
The analytics gathered from these APIs can also be used to gain valuable insights into viewer behaviors. This data-driven approach could offer content creators and marketers a much clearer view of audience preferences and trends, allowing for more tailored content creation strategies. However, it also raises concerns about what happens to this information and whether it's used responsibly.
Lastly, in sectors like healthcare or finance, where video data may be highly sensitive, the APIs offer advanced identification and security features that enhance compliance and data protection. This is a necessary step in managing critical content, but raises further questions regarding what level of control and transparency users have regarding their data.
Overall, the development of these APIs shows an increased effort to address more nuanced needs in the realm of video search. While the promises of more accurate and refined results are appealing, careful consideration should be given to the impact on user experience, data privacy, and ethical considerations. As these tools mature, the importance of balancing their potential benefits with their associated risks will only continue to grow.
Experience error-free AI audio transcription that's faster and cheaper than human transcription and includes speaker recognition by default! (Get started for free)
More Posts from transcribethis.io: