Experience error-free AI audio transcription that's faster and cheaper than human transcription and includes speaker recognition by default! (Get started for free)
NVIDIA AI Microservices for Drug Discovery Integrates with AWS A Closer Look at the 2024 Collaboration
NVIDIA AI Microservices for Drug Discovery Integrates with AWS A Closer Look at the 2024 Collaboration - NVIDIA's NIM Platform Debuts on AWS Marketplace
NVIDIA's NIM platform, encompassing NVIDIA Inference Microservices, has become available on the AWS Marketplace. This move aims to simplify the deployment of high-performance AI models for various tasks. NIM is engineered to be user-friendly while still offering robust capabilities, including a selection of tools tailored to healthcare applications. It seamlessly links with popular AWS services like EC2 and SageMaker, which smooths the path for deploying AI at scale.
The platform's relevance to drug discovery is underscored by the inclusion of specialized models like MolMIM for chemistry generation and ESMFold for predicting protein structures. By integrating with AWS, NVIDIA hopes to empower healthcare and life sciences companies to navigate the hurdles inherent in drug discovery and broader digital health endeavors. This isn't an isolated initiative, as NVIDIA's broader strategy seems to be building an interconnected network of partners, having already partnered with Microsoft Azure. While it aims to accelerate the use of generative AI in healthcare, it remains to be seen how seamlessly this integration unfolds and how readily adoptable these features become within the wider AI community.
NVIDIA's NIM (NVIDIA Inference Microservices) platform has made its way onto the AWS Marketplace, becoming part of the NVIDIA AI Enterprise suite. It's essentially a collection of user-friendly tools designed for deploying AI models efficiently and securely, specifically targeting high-performance inference tasks.
One of the key aspects is its tight integration with AWS's own cloud services like EC2, EKS, and SageMaker. This integration is intended to make it easier to deploy and manage large-scale generative AI projects. A notable point is that NVIDIA has tailored certain tools within NIM for healthcare, providing access to a curated library of AI models optimized for medical applications.
Within this platform, you'll find models focused on drug discovery, including MolMIM for generating novel chemical compounds, ESMFold for protein structure prediction, and DiffDock for analyzing how drugs interact with their targets. It's interesting how this collaboration between NVIDIA and AWS is aiming to assist pharmaceutical and healthcare organizations in addressing the hurdles associated with drug discovery and related digital health initiatives.
It's worth noting this is an extension of NVIDIA's work with Microsoft Azure, suggesting they are looking to support a wider AI ecosystem rather than being tied to a single cloud platform. Furthermore, NIM connects with NVIDIA NeMo, a comprehensive platform for building custom generative AI solutions. NeMo provides users with the ability to train, customize, and manage their own models and data.
Ultimately, NIM looks to accelerate the adoption of generative AI across various industries, particularly in healthcare, by offering user-friendly deployment tools. The ongoing partnership between NVIDIA and AWS seems to have a clear focus on driving innovation within generative AI, especially as it pertains to advancements in healthcare and drug discovery research. This particular avenue could be impactful for researchers and hopefully help accelerate the discovery of new treatments.
NVIDIA AI Microservices for Drug Discovery Integrates with AWS A Closer Look at the 2024 Collaboration - Integration of BioFMs Accelerates Drug Discovery Process
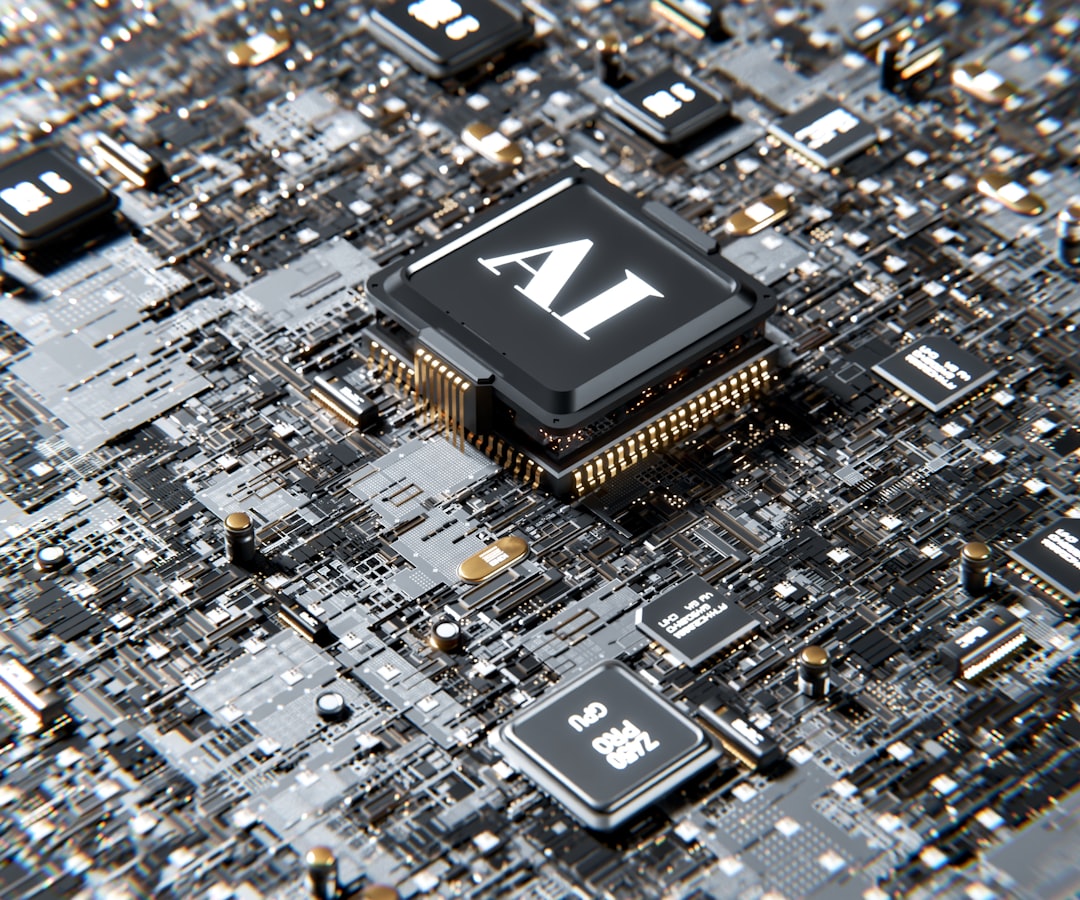
The integration of Biological Foundation Models (BioFMs) is significantly accelerating the drug discovery process. These advanced generative AI models, trained on vast biological datasets, are empowering researchers to tackle complex challenges in drug development. By leveraging BioFMs in conjunction with tools like NVIDIA's Generative Virtual Screening Blueprint, scientists can now more efficiently predict the 3D structures of protein targets and optimize the design of small molecules to interact with them. This translates to a faster and more streamlined drug discovery pipeline, particularly when it comes to handling the integration of diverse biological data, which has historically been a major hurdle.
The collaboration between NVIDIA and AWS, and other cloud platforms, showcases a broader trend towards embracing digital biology in the pharmaceutical industry. However, while these advancements hold immense promise, it's crucial to remember that their real-world impact and ability to fully translate into practical applications are still being evaluated. As AI increasingly becomes interwoven with the drug discovery process, it's vital to maintain a cautious optimism, acknowledging both the potential benefits and the limitations of these technologies as we strive for a more efficient and effective approach to therapeutic development.
The integration of Biological Foundation Models (BioFMs) is accelerating the drug discovery process in fascinating ways. These advanced AI models are specifically built to handle the complex data generated from biological experiments, seamlessly integrating it into AI workflows. This rapid integration allows researchers to extract meaningful insights more quickly, leading to faster iterations within the drug development pipeline.
One of the notable benefits is the potential to enhance the identification of drug targets. BioFMs can sift through massive datasets of genomic and proteomic information, making it easier to pinpoint potential drug targets. This is a significant improvement over traditional methods, which can be both time-consuming and resource-intensive.
Moreover, the combination of BioFMs with NVIDIA's NIM platform enables the use of cutting-edge machine learning techniques to predict molecular interactions with exceptional accuracy. This increased accuracy has the potential to dramatically reduce the time needed to discover new therapeutic options compared to traditional approaches.
The ability to scale up analyses is also a game-changer. Deploying BioFMs on AWS allows researchers to handle large-scale data analysis without the need for significant upfront investments in physical infrastructure. This is particularly beneficial as it removes a common barrier for many researchers.
Further, BioFMs provide real-time data processing, enabling immediate feedback on drug design choices. Researchers can quickly adjust their approaches based on comprehensive data analysis, rather than waiting for weeks or even months for results. This iterative approach could lead to more informed decisions and quicker optimization cycles.
Furthermore, the collaborative potential of BioFMs is impressive. They often utilize shared protocols and data formats, facilitating easier collaboration between researchers at different institutions. This multi-disciplinary approach can prove very effective for tackling complex drug discovery challenges.
The integration of BioFMs with existing laboratory information management systems (LIMS) is also made simpler using the toolkits provided within the BioFMs framework. This offers a streamlined path for laboratories to incorporate AI and leverage existing data structures for modernization.
BioFMs' predictive analytics capabilities are particularly intriguing. They can potentially anticipate potential pitfalls in the drug development process, such as negative side effects or inefficacy in specific patient groups, helping to reduce wasted time and resources associated with clinical trials that might fail.
The integration of BioFMs with NVIDIA's generative models also allows for the "design" of new molecules in a computer environment before they are synthesized in a lab (in-silico molecular design). This in-silico approach can streamline the R&D process and potentially enhance the quality of the lead compounds that move forward into clinical trials.
Ultimately, the use of BioFMs can increase cost-efficiency for drug development projects. By allowing researchers to prioritize promising research avenues, it may result in shorter project lifecycles and faster market entry for new medicines. However, as with any complex technology, it will be critical to address potential pitfalls and ensure accessibility for the broader scientific community. It remains to be seen how widely adopted these solutions become within the scientific community.
NVIDIA AI Microservices for Drug Discovery Integrates with AWS A Closer Look at the 2024 Collaboration - BioNeMo Framework Enhances Computational Drug Research
The BioNeMo framework is a notable development in computational drug research, focusing on the use of generative AI in chemistry and biology. It aims to streamline the drug discovery process by offering tools and workflows for different phases, like 3D protein modeling and lead compound optimization. Core to BioNeMo is its utilization of pre-trained large language models (LLMs) and specialized training methods, all optimized for drug discovery tasks. This platform, now available through a cloud service integrated with AWS, offers access to numerous advanced AI models and tools. While this integration enhances accessibility and scalability, there's a need to understand how seamlessly it translates into tangible results and becomes widely adopted. The future success of BioNeMo will depend on its ability to demonstrate practical benefits and overcome any barriers to implementation across the field of drug discovery. It holds much promise for a faster and more efficient drug discovery process, but it's important to maintain a realistic view of its potential limitations and hurdles.
BioNeMo, a specialized generative AI platform built for the intricacies of chemistry and biology, has emerged as a valuable tool in the quest for faster and more efficient drug discovery. It's designed to streamline the drug discovery process by offering tools that can be incorporated across multiple stages, from initial target identification to the optimization of lead compounds. This platform offers a comprehensive suite of workflows catering to tasks like 3D protein modeling and lead optimization, allowing researchers to seamlessly incorporate powerful AI models into their investigations.
NVIDIA's AI technologies are being leveraged by over a hundred companies, showcasing the growing reliance on computer-aided drug discovery and the increasing adoption of generative AI within this field. At the heart of BioNeMo lies a framework specifically crafted for computational drug research. It features pretrained large language models (LLMs), data loaders and optimized training recipes, all specifically designed to handle the unique challenges of drug discovery data. BioNeMo further simplifies things through a convenient cloud service integrated with AWS. This service provides users with access to a diverse selection of state-of-the-art language and diffusion models, all accessible through a single interface.
Furthermore, researchers can customize and refine these models within BioNeMo, using NVIDIA's infrastructure for streamlined processing and scalability. This empowers researchers with a greater degree of control and flexibility in their research. BioNeMo’s introduction represents a notable shift in how drug discovery is approached, suggesting a potential transformation within the field similar to the impact computing had on healthcare. The ability to tailor and customize AI models using BioNeMo is made easy through a user-friendly web interface or through managed APIs, allowing users to fine-tune the models for their particular needs. This is further enhanced by the fact that BioNeMo excels at supporting the development and deployment of generative AI models, placing it as a strong contender for the most effective tool within the AI-driven drug discovery space.
While potentially promising, it's important to be cautious in assessing the actual impact of BioNeMo and similar frameworks. The true effectiveness of integrating such advanced AI technologies into the complex landscape of drug discovery remains to be fully understood. Additionally, challenges like bias in training data and the interpretability of AI-generated results need careful consideration as this field evolves. Nevertheless, BioNeMo appears to be a well-designed tool that, if properly utilized, could significantly improve the drug discovery process.
NVIDIA AI Microservices for Drug Discovery Integrates with AWS A Closer Look at the 2024 Collaboration - Early Adopters Cadence and Iambic Therapeutics Lead the Way
Cadence and Iambic Therapeutics are among the first companies to embrace NVIDIA's AI microservices for drug discovery. They are part of a larger trend, with over 100 organizations now using NVIDIA's AI tools in the drug discovery space. Cadence is incorporating NVIDIA's BioNeMo microservices into its Orion platform. This integration is meant to enhance the use of AI in molecular design and drug optimization. Iambic Therapeutics is also making strides with its NeuralPLexer platform. NeuralPLexer shows a clear advantage in its ability to predict how proteins and drug-like molecules interact, outperforming other approaches in certain testing situations. The use of these AI platforms could potentially reduce the time and expense involved in drug development, particularly in areas like cancer treatment. Both Cadence and Iambic are currently working on getting their latest drug candidates into human clinical trials, suggesting a significant potential shift in the drug development process. The use of advanced AI in this manner highlights a fundamental change in how drug discovery might be approached in the future.
Within the broader landscape of AI-powered drug discovery, we're seeing a few early adopters really push the boundaries of what's possible. Companies like Cadence and Iambic Therapeutics are among over a hundred organizations adopting NVIDIA's AI tools for drug discovery. This isn't just a trend – it's a response to the immense challenges and costs associated with traditional drug development, where the average new drug takes over a decade and costs upwards of $1.2 billion to bring to market.
Cadence, for example, is incorporating NVIDIA's BioNeMo microservices into its Orion platform. The idea is to enhance the AI-guided process of designing and optimizing molecules. It's worth noting that this move is partly due to the expanded toolkit that NVIDIA's BioNeMo has rolled out, giving drug developers access to a more refined set of models. It remains to be seen how seamlessly these integrations actually work in real-world drug development projects.
Iambic Therapeutics, on the other hand, has been utilizing AI for a while now, particularly for cancer research. Their AI platform, NeuralPLexer, has shown remarkable promise, outperforming others in benchmark studies for predicting how proteins and drug molecules interact. They've just raised a significant sum of money ($100 million), aiming to accelerate two lead cancer drug programs into early-stage clinical trials. There is optimism that their AI-driven approach has helped speed up the drug discovery process, especially in oncology. It'll be interesting to see how quickly they can bring new therapies to patients. They're planning to bring a third undisclosed oncology program into trials soon after those two programs advance.
This collaboration between Iambic and NVIDIA is a specific example of how AI is aiming to turbocharge the drug discovery process, particularly in the complicated world of cancer therapeutics. While still in early days, we're beginning to see some potentially impactful real-world examples of the integration of these advanced AI models. It's crucial to maintain a healthy degree of skepticism, however. The practical applications of these powerful AI tools are still emerging, and while promising, their impact remains to be fully determined. As researchers, it's exciting to observe how they’re being leveraged and to consider the potential they hold to revolutionize healthcare, but the path forward will likely involve both breakthroughs and challenges.
NVIDIA AI Microservices for Drug Discovery Integrates with AWS A Closer Look at the 2024 Collaboration - AWS-NVIDIA Partnership Simplifies AI Integration in Biopharma
The collaboration between AWS and NVIDIA in 2024 is aiming to make it easier for the biopharmaceutical industry to incorporate AI into their operations, especially in drug discovery. They're utilizing advanced AI models, called Biological Foundation Models (BioFMs), trained on massive amounts of biological data to accelerate the drug development pipeline. NVIDIA's BioNeMo platform, designed specifically for generative AI in biology and chemistry, is now accessible through AWS, giving researchers more tools to work with. This partnership also provides access to over 25 newly developed AI microservices available on the AWS Marketplace, enabling a wider range of tasks in drug development. Although this collaboration seems to have strong potential to revolutionize drug discovery, the degree to which these tools become fully integrated and produce real-world impact is still uncertain. There is a need for researchers to approach these advancements with a balanced perspective, recognizing both the potential benefits and the unknown challenges that come with deploying AI on a large scale in healthcare.
The collaboration between AWS and NVIDIA is enabling Biological Foundation Models (BioFMs) to access flexible computing resources, which is essential in biopharma where computational needs can vary drastically depending on the project. This means researchers can scale up or down their computing power as needed, making it more efficient to handle the diverse demands of drug discovery.
NVIDIA's generative AI, now conveniently accessible through AWS, can potentially improve the accuracy of predicting how molecules interact. Traditionally, this has been a challenging task due to the intricate nature of biological systems, and AI models may offer more reliable predictions.
The introduction of over 25 microservices focused on healthcare, especially in drug discovery, could accelerate the pace of innovation. These microservices include models with specialized applications, suggesting they might streamline the preclinical phase of drug development by offering more precise predictions and reducing the amount of time spent on early-stage research.
Through BioNeMo's cloud service integrated with AWS, pre-trained AI models are now more readily available to researchers. This can open up advanced computational techniques to researchers in institutions that might not have had access to such powerful resources previously, democratizing, in a way, access to cutting-edge technologies.
Companies like Cadence are seamlessly integrating BioNeMo into existing platforms like Orion, showing the potential for these advanced AI tools to complement, not replace, existing infrastructure. This could be a key factor in making adoption of AI more palatable and less disruptive to established research workflows.
The ability of BioFMs to forecast outcomes can significantly impact clinical trial design. By identifying potentially unproductive trials before they start, researchers could prevent wasted time and resources, leading to a faster and more efficient development process.
Tools like DiffDock, which analyzes drug interactions, could increase the precision in selecting drug candidates for further development. This can help reduce the reliance on trial-and-error methods, which can be very time consuming and expensive in the drug discovery process.
Early adoption of NVIDIA's microservices, as seen with Iambic Therapeutics, is demonstrating tangible results in shortening drug development timelines, especially in the field of oncology. This validates the potential of these advanced technologies to have a real-world impact on drug development.
The AWS-NVIDIA partnership is shifting the emphasis towards cloud-based, scalable solutions for drug discovery. This approach aligns with current industry trends towards making faster, data-driven decisions, which could accelerate the overall pace of innovation.
This collaboration brings together NVIDIA's expertise in AI and machine learning with AWS's cloud infrastructure. This could lead to a future where biopharmaceutical companies can more easily collaborate and leverage shared computational resources to collectively develop and implement new solutions. It's an exciting time to be in this field, though we must remain cognizant of potential challenges and the need for independent, unbiased assessment of these technologies as they are put into practice.
Experience error-free AI audio transcription that's faster and cheaper than human transcription and includes speaker recognition by default! (Get started for free)
More Posts from transcribethis.io: