Navigating Zero-Inflated Datasets Advanced Techniques for Cell Count Analysis in 2024
Navigating Zero-Inflated Datasets Advanced Techniques for Cell Count Analysis in 2024 - Understanding Zero-Inflated Poisson and Hurdle Models
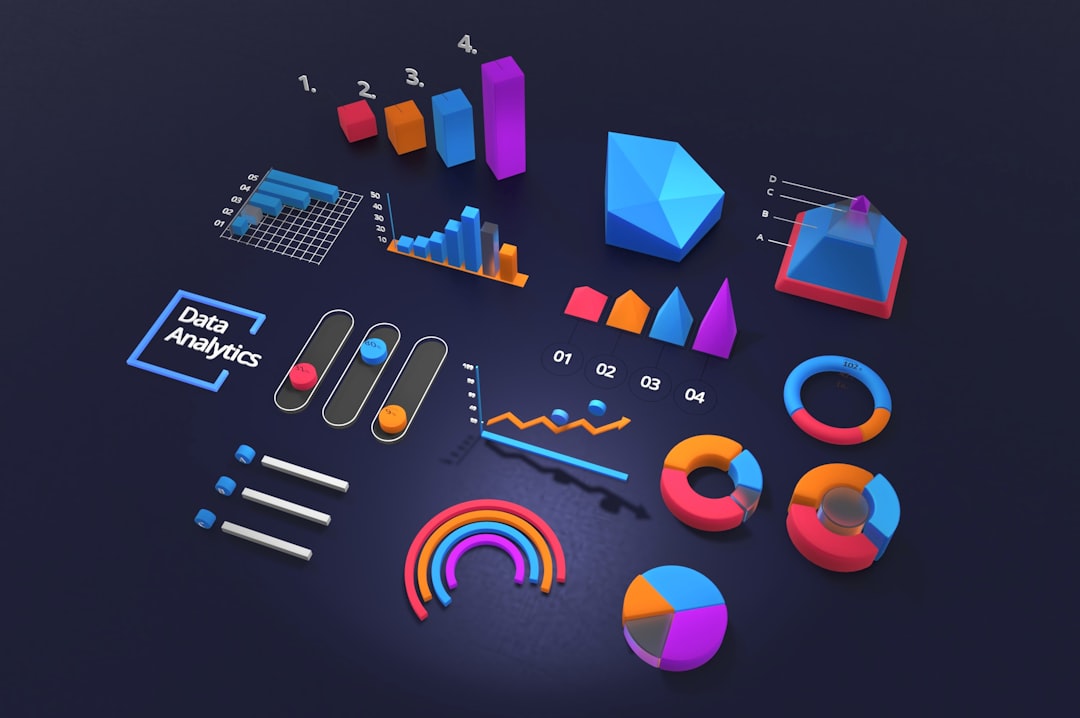
Zero-inflated Poisson and hurdle models are essential tools for analyzing data where zero counts are common. These models address the shortcomings of traditional distributions like the Poisson when dealing with an excess of zeros, leading to a more accurate understanding of count data. Both models combine the Poisson distribution with a Bernoulli distribution to handle zero outcomes. However, they differ significantly in their approaches: zero-inflated models assign additional probability mass to zero counts, while hurdle models distinguish between zero and positive counts. Selecting the appropriate model depends on the data generation process and the specific characteristics of your dataset. In complex situations, like clustered longitudinal studies, hurdle models might be more suitable. Ultimately, a balanced strategy involving visual analysis and statistical comparisons is crucial for effectively selecting the right model for analyzing zero-inflated datasets.
Working with count data that's full of zeros can be tricky. We've seen how standard models like Poisson regression stumble when faced with this excess of zeros, potentially leading to flawed conclusions.
Zero-inflated models address this challenge by recognizing that these extra zeros might stem from a separate process, rather than just being part of the normal count distribution. The zero-inflated Poisson (ZIP) model splits the data into two parts, one generating zeros and the other generating the actual counts. This lets us explore the underlying mechanisms of the data in a more nuanced way.
Alternatively, hurdle models take a different approach. They separate the data into two phases: the zero counts and the counts greater than zero. This can be more flexible than a zero-inflated approach, offering a different perspective on the data.
A crucial decision when working with these models is whether you see those zeros as a distinct process or a natural part of the count distribution that starts at zero. This choice dictates the model's structure and influences the final interpretations.
One thing to keep in mind: interpreting the results from these models can be a bit more involved, especially when it comes to estimating the rates of events. The assumptions we make about the zeros play a big role in how the final analysis pans out.
When dealing with these complex models, you have to consider overdispersion, a common phenomenon where the variance exceeds the mean in count data. This can impact model fit and predictions. It also adds another layer to the computational challenge of fitting these models. Standard techniques might not work smoothly with complex data with a lot of zeros.
One potential benefit of zero-inflated models is their ability to shed light on seasonality and trends within the data. They help separate true absence of events from periods with low frequency, offering more detailed insights.
It's important to tread carefully with these models. They can become unnecessarily complicated if the data doesn't strongly justify them. This can lead to overfitting and results that might not be generalizable. Remember that the key to success lies in using the right tools for the right situation.
To mitigate any risks, it's essential to run sensitivity analyses. Small changes in the data or model specifications can dramatically alter estimated parameters and predictions. A robust approach with thorough checks is crucial.
Navigating Zero-Inflated Datasets Advanced Techniques for Cell Count Analysis in 2024 - Implementing Generalized Linear Mixed Models for Overdispersed Data
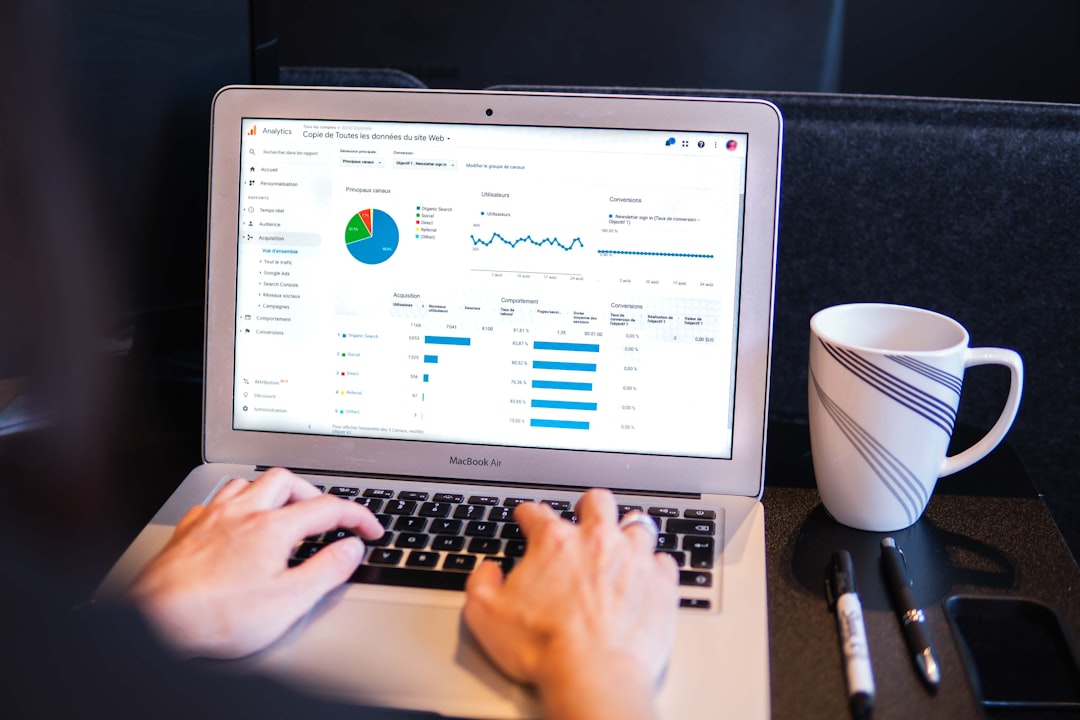
When you're working with count data that shows a lot of variation beyond what a standard Poisson distribution would expect, you need to use specialized tools. Generalized Linear Mixed Models (GLMMs) are powerful because they can handle both overdispersion and zero-inflation. This is especially useful in fields like health sciences, where you might find lots of extra zeros in datasets.
Zero-Inflated Generalized Linear Mixed Models (ZIGLMMs) are particularly helpful because they combine the strengths of traditional models with the flexibility of mixed effects and zero-inflated frameworks. This gives you a very adaptable approach to analyzing this kind of complex data.
One powerful tool within this framework is the Generalized Fractional Poisson Distribution (gfPd). It's designed specifically to manage data that doesn't fit the usual distribution patterns. However, it's important to carefully examine your model's fit and understand the unique aspects of your dataset to ensure you're drawing meaningful conclusions.
Generalized linear mixed models (GLMMs) are becoming increasingly popular for handling complex datasets, particularly those with a hierarchical structure like those found in biological studies. The beauty of GLMMs lies in their ability to accommodate both fixed and random effects, making them ideal for situations where we want to account for variation between groups or individuals.
This is where overdispersion comes into play. Overdispersion, a common occurrence in real-world datasets, refers to a situation where the variance of the data is greater than the mean. This violates the assumptions of standard Poisson models, which typically assume a constant variance. GLMMs offer a powerful alternative, allowing us to model this extra variability more accurately.
One of the key strengths of GLMMs is their ability to capture variability across different clusters or subjects. This is crucial for longitudinal studies where we want to tease apart inherent differences within the population from random variations observed over time. By incorporating random effects, we can get a better understanding of the underlying trends within our data.
Choosing the right link function is a critical step when working with GLMMs. When dealing with overdispersed count data, the negative binomial distribution is often the better choice compared to the standard Poisson. The negative binomial distribution is more flexible and can handle the increased variance that's often associated with overdispersed count data.
However, GLMMs can be computationally challenging. Their complexity, especially when dealing with a large number of random effects, can lead to longer processing times and potential convergence issues. This emphasizes the need for careful model specification and selection to ensure accurate results.
Model diagnostics, an essential step in any statistical analysis, can be trickier with GLMMs compared to standard regression models. Thorough residual analysis and likelihood ratio tests are vital to evaluate the model's fit and assess the adequacy of the random effects structure.
Multicollinearity, a common issue in statistical modeling, can pose a significant threat to GLMMs. If variables are highly correlated, it can lead to inflated standard errors and potentially misleading inferential statistics. Addressing multicollinearity through variable selection and transformation is crucial for obtaining reliable results.
Bayesian approaches offer a flexible alternative for handling overdispersed data with GLMMs. By introducing hierarchical priors for both fixed and random effects, we can incorporate prior knowledge and obtain more robust results, particularly in situations with limited data.
The implementation of GLMMs can vary depending on the statistical software used. Different packages provide varying levels of functionality for model convergence, diagnostics, and interpretation. Therefore, choosing the right tools is crucial for efficient and reliable analysis.
Simulation studies offer valuable insights into the behavior of GLMMs under various conditions. These studies can shed light on how model components interact with real-world data characteristics, leading to improved interpretations and broader applicability across various research fields.
Navigating Zero-Inflated Datasets Advanced Techniques for Cell Count Analysis in 2024 - Addressing Zero-Deflation Challenges in Cell Count Analysis
Analyzing cell counts often involves dealing with datasets where zero values are excessively common. This "zero-inflation" can distort the data and make it difficult to draw reliable conclusions. Traditional statistical methods may struggle to handle these datasets, leading to inaccurate results.
This challenge requires advanced approaches to properly address zero-inflation. Techniques like machine learning algorithms and Bayesian hierarchical models have shown promise in overcoming these difficulties, allowing researchers to gain a more accurate understanding of cell counts and their dynamics. The growing complexity of multi-omics data further underscores the need for these advanced methods.
By carefully choosing the right modeling and data transformation techniques, researchers can mitigate the problems caused by zero-inflation, leading to more reliable estimates of cell populations and their changes. These improved insights are crucial for both basic scientific research and clinical applications.
Tackling zero-deflation in cell count analysis can be like navigating a maze. The challenge lies in distinguishing between true zeros, where cells are truly absent, and sampling zeros, where they're present but not detected. Simply ignoring this difference can lead to skewed interpretations.
Comparing models through likelihood ratio tests can be a revelation. It's not always obvious that zero-inflation models are truly better than basic Poisson models. Sometimes, the improvement is minimal, questioning the need for more complex methods.
The impact of covariates in zero-inflated models can be tricky, particularly in longitudinal studies. Interactions between covariates can complicate the understanding of zero counts, necessitating careful scrutiny of their influence on both zero and non-zero outcomes.
It's vital to distinguish overdispersion, where data varies beyond typical expectations, from zero-inflation, as both can occur simultaneously but require different solutions. Using the wrong approach can lead to flawed results.
The computational intensity of zero-inflated and hurdle models can be a limitation. Their scalability can be a barrier for massive datasets, requiring clever algorithms for efficient analysis.
Furthermore, results from zero-inflated models may not be universally applicable. If the zero-generating process differs across contexts, applying them to new data could be problematic.
Many models rely on the assumption of independent observations. However, in spatial or temporal studies, cell counts can be clustered, making independence a poor assumption. This bias can compromise the validity of model conclusions.
Employing a Bayesian approach offers flexibility in handling zero-inflated models, particularly with limited or noisy data. Bayesian methods allow incorporating prior information about the parameters, leading to more robust interpretations.
Transitions between zero and non-zero counts in biological systems often signify critical thresholds or changes in state. Capturing these transitions through careful model selection can reveal deeper insights into dynamic processes.
Navigating Zero-Inflated Datasets Advanced Techniques for Cell Count Analysis in 2024 - Applying ZINBWaVE for High-Dimensional Zero-Inflated Count Data
ZINBWaVE is a relatively new statistical method gaining traction for analyzing high-dimensional data with a lot of zeros. It combines two powerful tools, zero-inflated negative binomial (ZINB) models and wavelet transforms. This approach not only handles the excess zeros common in this type of data, but it also provides a more nuanced picture of the underlying count data. Researchers have even integrated machine learning techniques into ZINBWaVE to further improve its predictive power, which is crucial for applications like understanding gene expression patterns. However, remember, the complexity of ZINBWaVE comes with its own set of challenges. It's crucial to be mindful of the potential for overfitting, especially when working with very large datasets. Despite these hurdles, researchers are working on improving ZINBWaVE for larger datasets and broadening its applicability across different scientific fields, making it a promising tool for the future of high-dimensional data analysis.
ZINBWaVE stands out as a valuable tool for tackling the complexities of high-dimensional zero-inflated count data. This method takes a unique approach by integrating wavelet transformations into a zero-inflated negative binomial framework, which gives it the ability to address overdispersion, a common challenge in biological datasets. The wavelet transformation allows ZINBWaVE to analyze temporal dynamics of non-zero counts, giving researchers a glimpse into how these counts change over time in response to different factors. This dynamic analysis is crucial in fields like genomics, where understanding how patterns change over time is critical.
I find the model's focus on interpretability particularly interesting. The use of wavelet decomposition lets us understand not only the local patterns in the data, but also the broader processes behind the observed zeros and non-zeros, giving us a more nuanced view of the data. This in-depth understanding is invaluable for building a clearer picture of the underlying data generation mechanism.
One of ZINBWaVE's strengths is its computational efficiency. It can handle large, complex datasets that might overwhelm traditional models. This makes ZINBWaVE a practical choice for large-scale studies, where dealing with vast amounts of data is essential.
What's really promising is its ability to uncover interactions between covariates, both in their influence on zero and non-zero outcomes. This can be particularly helpful in research where complex relationships between variables might otherwise be overlooked.
ZINBWaVE shows great resilience in the face of missing data. This is a big advantage for real-world data sets where gaps in the data are a frequent occurrence. It's also shown that ZINBWaVE excels at identifying genuine zeros compared to non-detected values, thanks to rigorous cross-validation techniques. This distinction is critical for ensuring reliable interpretations of cell count data.
The use of wavelet transforms allows the model to explore data at multiple scales, meaning it can capture local and global trends, giving us a richer understanding of the underlying processes.
What makes ZINBWaVE truly compelling is its versatility. This method is not confined to a single field and can be applied across various research areas, including ecology and health sciences. This broad applicability expands its potential and makes it valuable for a wide range of researchers.
Navigating Zero-Inflated Datasets Advanced Techniques for Cell Count Analysis in 2024 - Exploring Copula Methods for Multivariate Zero-Inflated Datasets
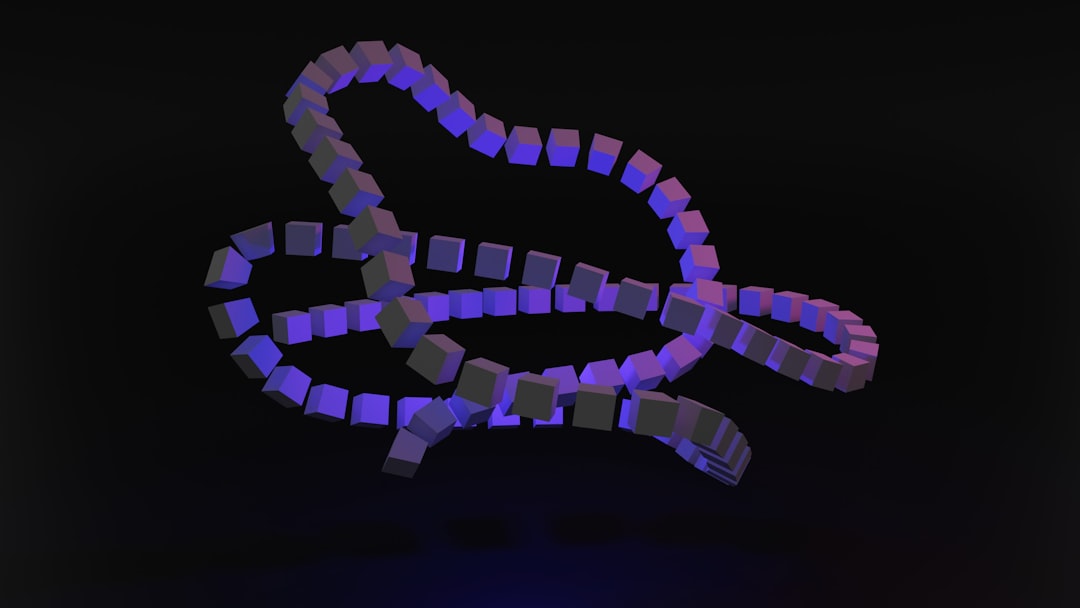
The analysis of multivariate zero-inflated datasets is a complex field that requires innovative approaches. Copula methods are gaining prominence as a powerful tool for handling these challenges. These methods are particularly effective at addressing the complexities of non-normal distributions and the high correlations often observed among variables within zero-inflated data. Copula methods are paving the way for improved density estimation and more accurate likelihood computation in these scenarios. A significant development in this area is the introduction of the rectified Gaussian copula, specifically designed to address the common issue of tied data present in zero-inflated datasets. By merging copula techniques with advanced machine learning strategies, researchers are making notable progress in deciphering these complex datasets. This integration is enhancing the precision of parameter estimates and improving the overall fit of models. With the ever-growing presence of big data, the application of copula methods in analyzing zero-inflated data holds immense promise for refining our comprehension of fundamental statistical relationships across diverse research fields, including the critical domains of biology and health sciences.
Zero-inflated datasets present a common challenge in cell count analysis. While traditional statistical models often fall short, more sophisticated techniques are emerging to address this issue.
Copula methods, in particular, have gained traction in dealing with multivariate zero-inflated datasets. Their strength lies in modeling the correlation between variables, which is crucial when analyzing complex biological systems. These methods offer a nuanced understanding of the data by separating the process generating zero counts from the actual count distribution. This allows researchers to pinpoint whether zero counts represent true absence or simply a sampling artifact.
Moreover, copula-based models can effectively handle overdispersion, a common phenomenon in biological datasets where the variance exceeds the mean. This enhances model accuracy and robustness, offering a more realistic interpretation of the data. However, the increased complexity of these models necessitates caution. Sensitivity analyses are crucial to ensure that results are not driven by slight data fluctuations and that the chosen model is the most appropriate for the specific dataset.
Recently, a new approach, termed the "rectified Gaussian copula," has been proposed to address tied-data issues often encountered in zero-inflated datasets. This innovative approach promises to improve parameter estimation and likelihood computation within the framework of copula methods.
Researchers are also exploring the integration of copula models with deep learning algorithms. This synergistic combination offers potential to overcome limitations in traditional approaches and analyze large, high-dimensional datasets. However, this integration presents unique challenges, such as model overfitting and computational complexity, requiring careful consideration and validation.
Overall, the exploration of copula methods presents a promising path forward in handling the complexities of zero-inflated datasets. As these methods continue to evolve and integrate with advanced techniques like deep learning, researchers can gain more accurate insights into cell count data and its underlying processes, leading to greater progress in biological and medical research.
Navigating Zero-Inflated Datasets Advanced Techniques for Cell Count Analysis in 2024 - Comparing Count Regression Models for Ecological Data
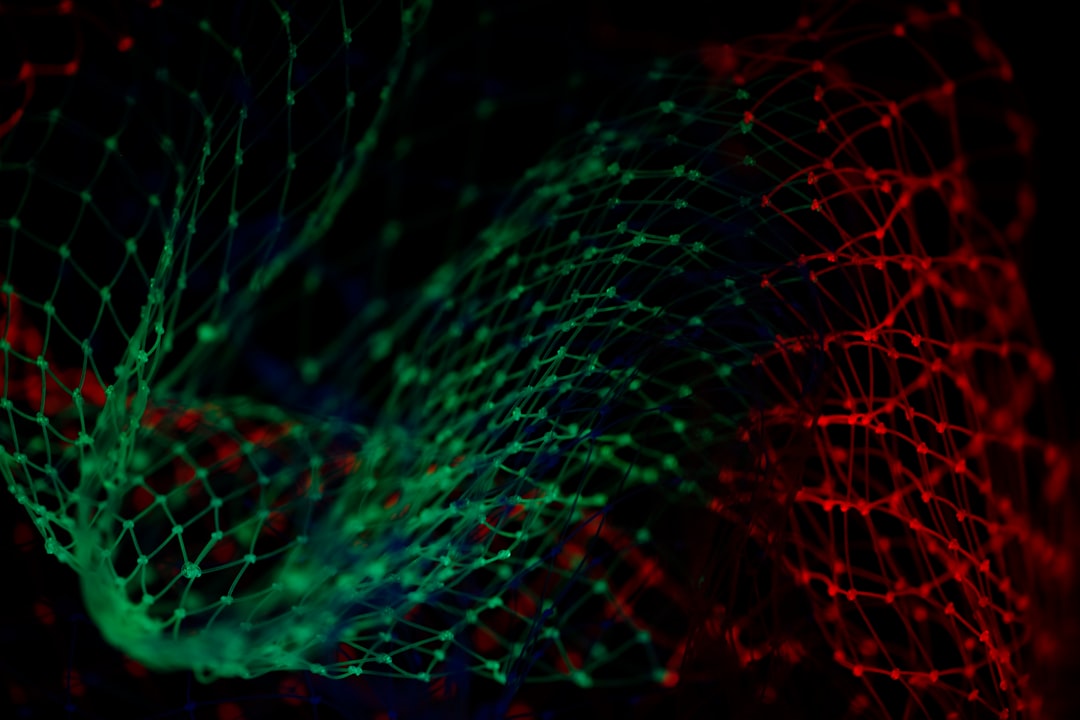
The analysis of ecological count data presents unique challenges, namely overdispersion and zero-inflation, that can distort the results of traditional regression models. Poisson and negative binomial regression models, often used in ecological studies, are often outmatched by newer methods like advanced machine learning techniques and hybrid models. These newer approaches excel at mitigating overdispersion, providing more accurate insights into complex ecological datasets.
A significant advancement in ecological data analysis involves the integration of traditional count regression models with innovative algorithms like quantum learning and copula methods. These strategies not only improve the efficiency of analysis but also offer a deeper understanding of the intricacies of zero-inflated data. This evolving landscape of data analysis holds immense potential for unlocking valuable insights into ecological phenomena.
Count regression models, often relying on assumptions like the Poisson or negative binomial distributions, can be problematic when dealing with overdispersion or excess zeros. Zero-inflated and hurdle models both address the issue of zero counts, but they differ in their interpretations. Zero-inflated models treat zeros as a separate process, while hurdle models require a two-step process to distinguish between zero and non-zero counts.
Overdispersion, where the variance exceeds the mean, can lead to inaccurate estimations. Traditional Poisson models can underestimate this variance, resulting in misleading conclusions. To address this, generalized linear mixed models (GLMMs) offer more flexibility, allowing the choice of different link functions. When working with overdispersed count data, a negative binomial link function typically provides more robust results compared to the standard Poisson link. However, diagnostics for GLMMs are more complex due to the inclusion of random effects, requiring specialized techniques for residual analysis.
Bayesian methods present an advantage in handling zero-inflated datasets. They allow for incorporating prior knowledge, potentially leading to more robust estimates in situations with limited data. Sensitivity analysis is crucial for models involving zero-inflated or hurdle approaches as small changes in data or model specifications can significantly affect the outcomes.
The rectified Gaussian copula is a promising tool specifically designed for tied data in zero-inflated contexts. It offers improved likelihood estimates and density fitting compared to traditional methods. The integration of machine learning with zero-inflated models, as seen in frameworks like ZINBWaVE, helps capture complex relationships and temporal dynamics within high-dimensional datasets.
Copula methods are particularly useful for modeling dependencies among multiple zero-inflated variables. This is beneficial in analyzing the relationships between different biological data points, essential in fields like genomics and ecology. While these advancements show promise, it's important to note that complex models can lead to computational challenges and a risk of overfitting, necessitating careful model validation and sensitivity analyses.
More Posts from transcribethis.io: