Experience error-free AI audio transcription that's faster and cheaper than human transcription and includes speaker recognition by default! (Get started for free)
MIT and Google's HealthLLM A Deep Dive into Wearable Sensor Data Analysis for Health Predictions
MIT and Google's HealthLLM A Deep Dive into Wearable Sensor Data Analysis for Health Predictions - MIT Google Partnership Creates Novel Health Data Framework for Wearables
The MIT and Google partnership has produced HealthLLM, a new framework that uses large language models for health predictions based on wearable sensor data. It uses data from many devices, plus public health datasets, to assess how well the models perform on new health-related tasks. Testing on eight top LLMs, including GPT-4, shows these models can handle complex health information, including personal details and past health. The framework's performance is on par with specifically-designed methods, suggesting AI can be useful in monitoring health. This work points to a possible future of improved health prediction and management by analyzing data from wearable devices.
MIT and Google have established a novel health data framework. This setup brings together data from a variety of wearable sensors, creating a central hub for analysis. Machine learning algorithms within this framework process extensive health data in real time, potentially leading to quicker health predictions and personalized interventions.
The framework has been engineered to analyze things like heart rate variability and sleep, aiming to provide tailored insights helpful for managing long-term health issues. Critically, it uses federated learning, enabling the models to improve while maintaining user privacy by keeping sensitive data on individual devices. It’s intended to help health tech companies collaborate on shared insights, all the while adhering to privacy regulations.
Early results seem to indicate that this new framework is more accurate with health predictions than older systems, likely because of its ability to look at complex relationships between multiple data streams from different wearables. Moreover, this isn't just for general health metrics; it can also integrate data from specialized wearables, like those tracking glucose or cardiovascular function, further individualizing results. The design is modular, making it adaptable to new sensors and technology without a complete system rebuild.
This work is generating critical discussion around the ethics of data use in health tech, highlighting the need for transparency and informed consent in decentralized data collection. Future development is focused on advanced predictive analytics. The goal appears to be to transform how healthcare professionals make decisions using real-time, wearable data.
MIT and Google's HealthLLM A Deep Dive into Wearable Sensor Data Analysis for Health Predictions - Real Time Analysis of Sleep and Heart Rate Data Shows Promise in Health Monitoring
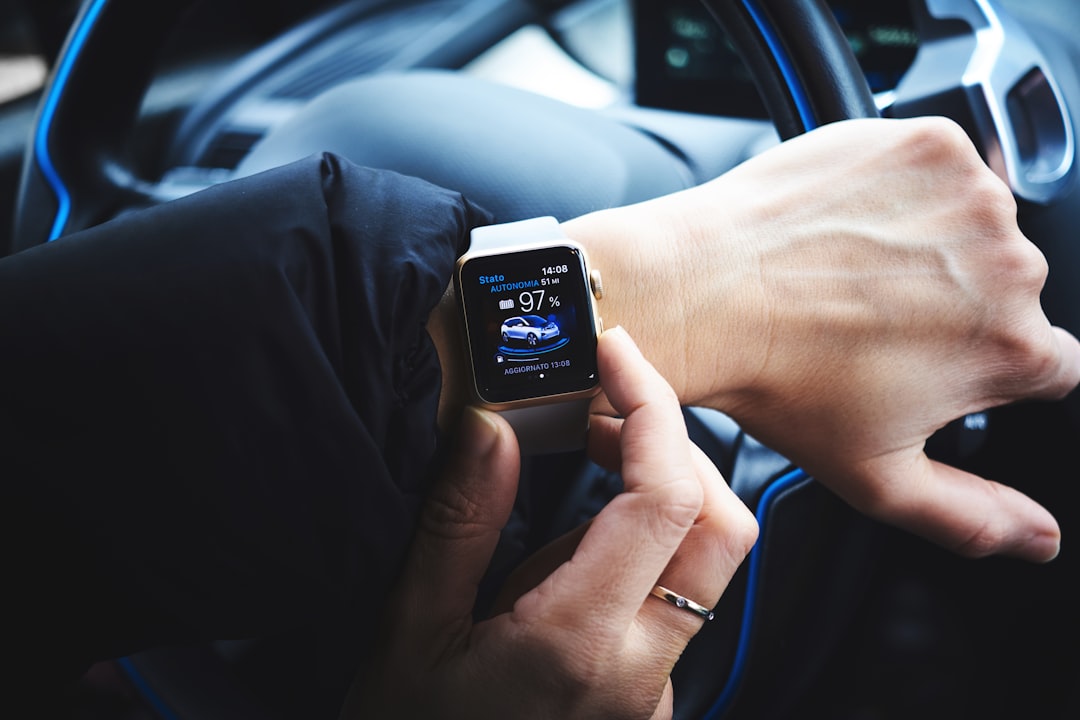
The continuous monitoring of sleep and heart rate data is gaining traction as a valuable method for health tracking. Wearable devices now allow the constant collection of key health metrics, giving insight into a person's health status without disrupting their daily routine. Advanced data analysis techniques can identify deviations in sleep patterns and heart rate, which may indicate developing sleep problems. These technologies show potential for more targeted health interventions, tailoring recommendations to a person's unique needs through continuous, precise physiological information. Nevertheless, increased use of these systems poses vital considerations regarding data protection and the ethical use of private health data.
Analyzing sleep and heart rate data in real time shows promise in picking up deviations from normal patterns that could signal underlying health problems. Things like irregular heartbeats or sleep issues might be spotted much sooner than using traditional methods. Beyond just physical health, research indicates that heart rate variability, when combined with sleep information, could offer a glimpse into our emotional states. This brings a more complete picture of someone's overall well-being.
By pairing sleep analysis with heart rate monitoring, we could learn more about how sleep and heart health relate. It’s possible that disrupted sleep cycles may strongly increase the risk for heart conditions. Current studies are now exploring if real-time sleep analysis might even hint at things like anxiety and depression, by looking at physical signs before any mental or emotional symptoms might become apparent.
The ongoing stream of data from wearables can help machine learning models tailor themselves to the person, refining their predictions over time. It can then generate personalized suggestions. This approach differs significantly from standard methods that tend to only focus on one source of information at a time. By handling data from many different wearables simultaneously, we could be better able to see a much more complete snapshot of overall health.
By using federated learning to train models with user data, the system can balance development with user privacy, keeping personal data secure. Studies also suggest that people using their own data are seeing improvements, supporting the idea that wearables can help you be proactive rather than reactive when it comes to your own health. Plus, heart rate data from sleep strongly correlates with sleep quality. This gives us an objective measure beyond just how someone describes their own sleep experience. This kind of predictive power opens avenues for things like real-time alerts about irregularities, like abnormal heart rates, and could actually trigger timely medical care.
MIT and Google's HealthLLM A Deep Dive into Wearable Sensor Data Analysis for Health Predictions - Machine Learning Models Process Data from Four Major Health Databases
Machine learning models are actively analyzing data sourced from four substantial health databases. This activity is geared towards improving clinical decision-making tools and refining personalized healthcare strategies. The models use both electronic health records (EHR) and data captured by wearable sensors. The objective is to discover patterns and insights that could notably support health monitoring and preventative actions. Even though these machine learning models offer great possibilities, they are still facing implementation hurdles and serious ethical issues regarding data handling. Current research efforts are directed towards enhancing these machine learning applications' reliability and security, especially when it comes to ensuring fairness in healthcare access and outcomes. As machine learning operations (MLOps) in healthcare gradually matures, we can expect to witness more practical uses of these technologies, which may lead to more reliable health predictions and better results for patients.
Machine learning models powering this kind of health analysis are fueled by data from four major health databases. Each brings something distinct to the table. Some include genomic information, while others capture data on lifestyle habits. This variety adds depth and perspective to the analysis. By incorporating data from different sources, the AI's insights can be adapted to a range of different personal circumstances. The core idea is to spot complex patterns that might not be apparent with traditional health monitoring. This could be especially beneficial for managing long term health issues which often take a long time to develop. Data gets processed over time which might lead to earlier intervention. The use of federated learning is interesting, since it allows the system to learn from varied data sources without ever handling sensitive health records directly. This maintains individual privacy and improves accuracy by drawing from the rich diversity of the datasets. New sensor tech could be brought in easily because the whole thing is designed to be modular. This means it won’t be obsolete when a new device is launched. The goal of using machine learning here is to detect subtle shifts in health metrics which could give warning signs of things such as heart problems or sleep problems. The system would adapt to change in real time as conditions vary. Research suggests that combining sleep data and heart rate data reveals information about both physical and psychological well being. An analysis of data in the aggregate gives scientists a view of population health trends. This is important since we could detect shifts in health across a whole group and adapt interventions to public health needs. Alerts for things like unusual heart rates or irregular sleep could change emergency medical response. In the long run, these AI powered real time alerts could potentially get users to seek medical attention faster, before things become an emergency.
MIT and Google's HealthLLM A Deep Dive into Wearable Sensor Data Analysis for Health Predictions - Consumer Health Tracking Expands Beyond Step Counting to Mental Wellness
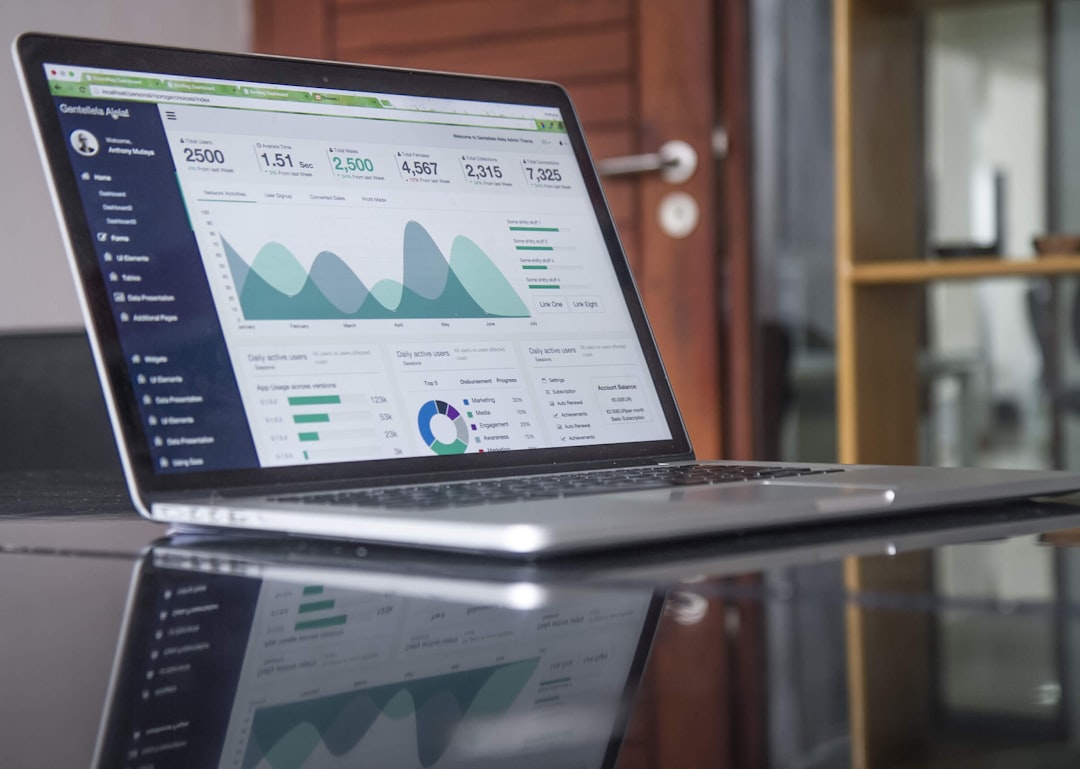
Consumer health tracking is moving beyond simple step counts to include mental wellness and a more complete view of health. This change is being driven by projects like the MIT and Google's HealthLLM, which use data from wearable sensors to try to make better health predictions. These technologies aim to spot and help with both physical and mental health by looking at various health data together. There's a growing understanding that these two are connected. Models like HealthAlpaca are showing good results in mental health analysis, pointing to the need for tech solutions for the increase in mental health concerns. While younger people are adopting this more thorough tracking, there are still questions about how the data is being used and if mood-tracking tech is actually helpful.
Consumer health tracking is now moving past basic step counting, incorporating metrics linked to mental wellness, to better understand overall health. There's a growing acknowledgment of how closely mental and physical health intertwine.
Research suggests heart rate variability (HRV) could be a key emotional indicator. Linking HRV and sleep data from wearables could allow predictive algorithms to spot mental health issues, like anxiety or depression, even before the person experiences obvious signs.
Machine learning is also starting to look at qualitative information, such as moods and stress, in addition to numbers, moving toward more tailored insights that match people’s individual experiences rather than general averages.
These health tracking tools may soon become predictive frameworks, where early signals from wearables allow doctors to act sooner for both physical and mental health emergencies, possibly shifting standard treatment methods.
The blending of machine learning and consumer health data raises tricky ethical problems around sensitive mental health data. How to keep things transparent, and allow the person to have full control of their information is hotly discussed by tech developers and government.
Studies reveal predictive models are much more accurate when many sources of information are examined together with mental wellness indicators, which helps understand links between stress, sleep, and irregular heart rates.
It seems constant monitoring with wearables can help users understand more about their own health. By giving tailored insights on mental wellness, people can identify shifts in their state and do something about it.
Using federated learning is helpful in mental health applications since it allows models to learn from diverse data without needing to access personal data directly. This may reduce potential security problems with sensitive data.
Future mental wellness tracking tools might go beyond simple data collection by adding things like live cognitive checks or mood tracking. These may actively involve users in the process of monitoring their mental state.
As different fields start to mix when it comes to health tech – with wearable data informing psychological research – it could lead to new methods for handling mental health. It might very well redefine how we assess mental health in the future.
MIT and Google's HealthLLM A Deep Dive into Wearable Sensor Data Analysis for Health Predictions - Wearable Sensors Bridge Gap Between Daily Activities and Medical Insights
Wearable sensors are now increasingly connecting our everyday routines with medical understanding, offering continuous health monitoring through advanced tech. These gadgets collect and send physiological data, allowing doctors to identify health patterns and potential problems as they unfold. New developments, like biochemical sensors that check saliva and complete systems that look at both mood and stress, show the potential of wearables to go past just physical health and help with mental wellness, too. Despite their promise, challenges are still present. Notably, incorporating the huge amounts of data generated into healthcare systems and respecting user privacy remains an issue. As wearable capabilities evolve, they have the potential to reshape how we manage and predict our health. However, clear guidelines must go hand in hand with progress in order to protect user data and encourage trust.
Wearable sensors are showing promise in forecasting things like heart problems and sleep issues, potentially enabling interventions sooner, and therefore helping long term well being. It is also becoming clear that heart rate fluctuations (HRV) might give us insights into stress and emotional state, linking physical readings to psychological well being. By mixing different types of wearable data—like glucose levels and sleep patterns—machine learning might uncover complex links between many health measures, leading to more complex and subtle insights. Data handling techniques are now including federated learning, which may help improve accuracy of models without actually directly accessing data on personal devices and therefore may protect privacy, at least on paper.
An emerging area is analyzing more subjective data, like how someone reports feeling alongside sensor readings, maybe creating more personalized health checks. Machine learning may identify health trends that could reshape public health programs through looking at real time insights and allow for targeted treatments based on these patterns. The flexibility of the system at MIT and Google lets them plug in new tech as it comes out, which might make health tracking more useful without the whole system needing a big upgrade every time something new is released. It's also getting more and more obvious that bad sleep might cause heart problems, which suggests tracking sleep patterns using wearables might help stop these types of issues from forming. The data gleaned from wearable devices might not only shape decisions about your individual health, but it might also impact health policy, which would bring personalized health solutions to the public. Researchers are also examining how adding mood tracking features can enhance existing physical health monitoring to better acknowledge the link between mental and physical health in our prediction models.
MIT and Google's HealthLLM A Deep Dive into Wearable Sensor Data Analysis for Health Predictions - Research Results Show Accuracy Gains Over Traditional Health Prediction Methods
Research findings indicate that the HealthLLM, a project of MIT and Google, shows better accuracy than older methods in predicting health issues. By analyzing data from wearable sensors, this model uses complex machine learning to understand how different health signs interact. This approach has resulted in more precise forecasts compared to how electronic health records are usually processed. This new system is proving its ability to predict things like infections and the need for medical device changes, with a fairly high degree of accuracy. It also allows for real-time data processing. This system shows potential for future health management, but questions of ethical data usage remain crucial as development continues.
Studies indicate that employing large language models (LLMs), like the HealthLLM developed through the MIT and Google collaboration, show improved precision when it comes to forecasting health outcomes when compared to older health prediction methods. The efficacy of LLMs in this arena seems closely linked to their capacity to understand data that is both specialized and non-linguistic in nature, such as personal details along with data from wearable sensors, rather than just text. This analysis includes wearable sensor information, which research suggests might be more accurate at forecasting health outcomes than standard electronic health record methods. It appears that HealthLLM uses a very simple system that quickly summarizes wearable sensor information and puts it into a user-friendly format. This appears to be crucial for making these types of data more understandable to both humans and AI systems. The research further confirms that things like personal history and enhancements to models do alter how precise they can be when it comes to these types of tasks. The system’s capabilities for predicting treatment results are now being directly tested against advanced deep learning models, including some that are built to account for uncertain and missing data, such as recurrent marginal structural networks (rMSN) and counterfactual recurrent neural networks (CRN).
The studies showed a measure of accuracy at .73 when it comes to predicting infections and a slightly higher rate of .78 for forecasts related to needing medical devices explanted. These figures suggest a solid predictive ability in these LLM models. Researchers suggest that machine learning is suited for dealing with a large number of public health outcomes. This seems to indicate that it might be more applicable in scenarios where structured medical records don’t exist. The research in question seems to be a part of Project ALPHA, which is collaborating with Informa Pharma Intelligence to use the largest data set to date to analyze clinical trial results. A key trend appears to be the focus on health prediction that goes beyond regular medical records. This includes personality information, which seems to show a change toward comprehensive risk assessment in health modeling. It raises questions about how models might try to infer personality and the validity of such an inference in a predictive system.
Experience error-free AI audio transcription that's faster and cheaper than human transcription and includes speaker recognition by default! (Get started for free)
More Posts from transcribethis.io: