HuggingGPT Bridging AI Modalities for Complex Task Resolution in 2024
HuggingGPT Bridging AI Modalities for Complex Task Resolution in 2024 - HuggingGPT's Integration of Multiple AI Models for Complex Problem Solving
HuggingGPT's novel approach lies in its ability to orchestrate various AI models, including powerful language models, to tackle intricate problems that span across different areas and data types. It intelligently chooses the most appropriate AI tools based on user requests and the nature of the task, fostering seamless collaboration between specialized models to produce more detailed and impactful results. Experiments across a range of tasks, including language processing, image understanding, and audio analysis, have highlighted HuggingGPT's capacity to tackle these diverse challenges efficiently. Its emphasis on bridging different AI domains suggests a potential pathway towards achieving artificial general intelligence, a goal that remains elusive for individual AI models working in isolation. This interconnected design not only amplifies HuggingGPT's capability but also encourages wider participation and collaboration among researchers within the broader AI field. While it presents exciting potential, it's crucial to also consider the complexities of managing and coordinating such diverse sets of AI tools.
HuggingGPT is built upon the idea of combining different AI models, like those found on the Hugging Face hub, to tackle complex problems that go beyond what a single model could manage. Its core function is to understand a user's request and then choose the most suitable models based on their descriptions within the Hugging Face ecosystem. This approach enables it to effectively bridge various AI areas, such as language, vision, and speech, making it more adaptable to the wide range of tasks people might need.
The ability to orchestrate multiple models to work together means HuggingGPT can deliver results in a range of formats, including text, images, or even audio, providing a comprehensive view of the solution. It’s like having a team of specialized AI experts, each focusing on their area of strength, to collaboratively tackle a problem.
One interesting characteristic is its capacity to seamlessly switch between these AI models, adapting to the demands of the task at hand. This suggests that it's able to assess the situation and determine the most appropriate model in real-time, which could be quite helpful in dynamic and uncertain environments.
While it's impressive that HuggingGPT can integrate so many different AI models, it's important to consider its limitations. Currently, it seems to struggle with issues that require deep understanding of common sense and human experience. This suggests there's still work to be done in this area to enhance its accuracy and adaptability.
The architecture is constructed in a way that makes it easy to include new models as they are developed in the AI community. This ensures that HuggingGPT can remain updated with the latest advancements and stay relevant in the evolving field of AI. However, utilizing numerous models does lead to increased computational needs, which is something to keep in mind.
The researchers have tried to ensure that the models are trained using a varied set of data sources to reduce biases. It's a crucial effort, though the success of this approach is still something that requires careful observation, and it highlights the broader challenges of constructing reliable and equitable AI systems. Overall, HuggingGPT presents a compelling step forward in applying collaborative AI for tackling more complex problems. It’s an exciting development, and the prospect of seeing what it can accomplish in the future is promising.
HuggingGPT Bridging AI Modalities for Complex Task Resolution in 2024 - Task Planning and Model Selection Using Large Language Models
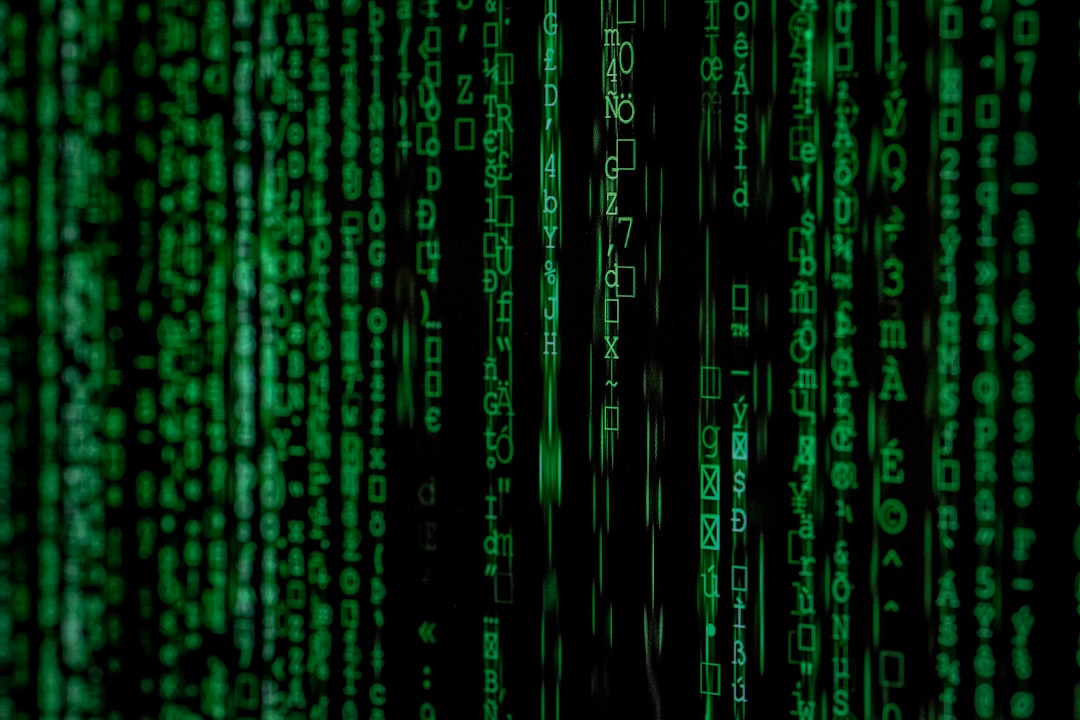
HuggingGPT's approach to task planning and model selection relies heavily on large language models to effectively break down complex tasks and allocate them to the most appropriate AI tools. This process involves carefully selecting the ideal model for each subtask, essentially treating model selection as a decision-making process where the most fitting model, based on its specific abilities, is chosen. By integrating a variety of AI domains—including language, image recognition, and audio processing—HuggingGPT improves the efficiency with which these tasks are completed. This ability to integrate different AI types positions HuggingGPT as a key player in the ongoing effort to build artificial general intelligence. While it has the potential to excel in coordinating a wide range of AI models, it also faces challenges. One area of concern is its ability to fully grasp common sense and human experiences, and it also requires substantial computing resources. The continued advancement of HuggingGPT's framework suggests its strong potential while highlighting the important aspects of ethical considerations for progress within AI.
Large language models (LLMs) are proving to be surprisingly adept at planning tasks and selecting the right AI model for the job. They can analyze complex requests and intelligently choose from a wide range of models, like those found on the Hugging Face hub. This dynamic model selection process seems to be key to HuggingGPT's effectiveness.
HuggingGPT's design promotes a real-time assessment of task requirements, allowing it to seamlessly switch between different models based on the current context. This adaptability appears to be a major contributor to both the speed and relevance of task solutions.
Research suggests that using a variety of AI models together not only handles complexity better but also leads to more reliable results. The strengths of one model can effectively balance out the weaknesses of others, resulting in a more robust outcome.
HuggingGPT uses an approach that combines outputs from different models. This ensemble method can produce more balanced and well-rounded solutions, but it also increases the computational load. It's a trade-off worth considering.
Even with its strengths, HuggingGPT still faces challenges in reasoning using common sense. This suggests that simply selecting models based on statistical correlations isn't enough for deep, nuanced understanding.
When quick decisions are needed, HuggingGPT's ability to shift between different AI modalities becomes especially useful. Its versatility makes it well-suited for unpredictable environments or situations requiring immediate adjustments.
The model selection within HuggingGPT uses a scoring system that factors in things like past model performance and the specific type of task. It's a clever way to optimize for the best model based on experience, rather than just trying different options randomly.
As HuggingGPT continues to integrate new AI models as they're developed, it raises questions about how we ensure reliability and oversight. New models will need careful evaluation to make sure they don't disrupt the overall consistency and trustworthiness of the system.
The collaborative design of HuggingGPT amplifies the abilities of individual models, but it also brings about operational challenges. One key challenge is the need for a system-wide understanding of the results in order to create a coherent final solution.
Researchers are working to reduce biases in these models by using diverse training data, but the complex nuances of human experience that impact model selection and task planning remain an area requiring significant improvement. It highlights that there's still much to learn and improve in these types of systems.
HuggingGPT Bridging AI Modalities for Complex Task Resolution in 2024 - Bridging Visual, Auditory, and Textual AI Domains in 2024
The landscape of artificial intelligence in 2024 is witnessing a growing emphasis on bridging the gap between visual, auditory, and textual domains. While strides in generative AI have led to increasingly realistic audio and visual outputs, the ability to seamlessly integrate these diverse modalities for deeper understanding remains a challenge. One notable hurdle involves the extraction and harmonization of semantic information from different data types, like translating the meaning of visual or auditory cues into a text-based understanding. Although promising work, like models that link specific sounds with their textual counterparts, has emerged, we're still facing limitations in achieving comprehensive, nuanced semantic interpretations across all modalities. Moreover, these efforts are often inspired by the way humans perceive and process multiple sensory inputs simultaneously. This drive to create a more human-like experience within AI is key, as it pushes us toward a more immersive and intuitive interaction with technology. While the aim of creating AI that seamlessly combines diverse sensory inputs is compelling, it's also clear that building a truly cohesive multimodal system involves overcoming substantial technical and conceptual barriers. The future of multimodal AI depends on successfully navigating these difficulties to unlock more engaging and useful applications.
Here are ten points about the exciting developments in merging visual, auditory, and textual AI domains, especially within the context of HuggingGPT in 2024:
1. **Multimodal AI's Growing Importance**: We're seeing that AI systems that blend visual, audio, and text data are exceeding the performance of single-modality systems, particularly in complex situations like analyzing video content or generating engaging stories. This suggests that combining data types can unlock new levels of understanding.
2. **The Dynamic Nature of Model Switching**: HuggingGPT’s clever ability to switch between different AI models as the task changes is a fascinating example of AI mimicking how humans adapt in different situations. It's crucial for efficiency in dynamic, unpredictable environments.
3. **Data Type Synergy**: Integrating data from images, sounds, and text is proving to be beneficial. For example, we're seeing how combining visual descriptions with audio clues can improve automated video captioning. It shows how different data types can enhance one another's value.
4. **Cross-Modal Attention for Better Comprehension**: Recent research highlights AI models that learn to pay attention to relevant parts of different data types at the same time. This "cross-modal attention" approach is improving how AI systems retrieve and understand information.
5. **Improved User Interactions Through Multimodality**: Research indicates that AI systems which smoothly combine different data types make it easier for people to interact with them. Reducing the mental burden on users can lead to faster learning and more intuitive responses, which is particularly valuable in education.
6. **The Risk of Bias Magnification**: While combining multiple data types is powerful, it also increases the risk of amplifying existing biases found within individual AI models. We need to be careful about this and proactively look for ways to reduce bias in training data to ensure fair and accurate outputs.
7. **Blending Model Outputs for Stronger Solutions**: HuggingGPT uses a method where it blends the results from multiple models. This technique doesn't just improve accuracy but also helps to make the overall solution more robust. It's a bit like having a backup system in place.
8. **The Ongoing Challenge of Common Sense**: Despite the impressive progress, AI still struggles with tasks that require common sense reasoning when dealing with multiple data types. This tells us that simply merging data doesn't automatically lead to deep, human-like understanding.
9. **The Increasing Complexity of Model Coordination**: As tasks get more difficult, the process of effectively coordinating models that deal with audio, visuals, and text becomes a major challenge. This complexity can significantly increase the computational resources needed, highlighting the balance between capability and efficiency.
10. **The Ethical Landscape of Multimodal AI**: The rise of multimodal AI like HuggingGPT brings about serious ethical questions about responsibility and decision-making, especially as these systems are becoming more prevalent in sensitive areas like healthcare and autonomous vehicles. It’s a crucial aspect that needs constant attention.
These observations highlight the incredible evolution of AI—it's not just a matter of adding more features, but rather about creating systems that work together in powerful ways, fundamentally changing how we approach complex problems.
HuggingGPT Bridging AI Modalities for Complex Task Resolution in 2024 - Enhancing AI Flexibility Through Continuous Expert Model Integration
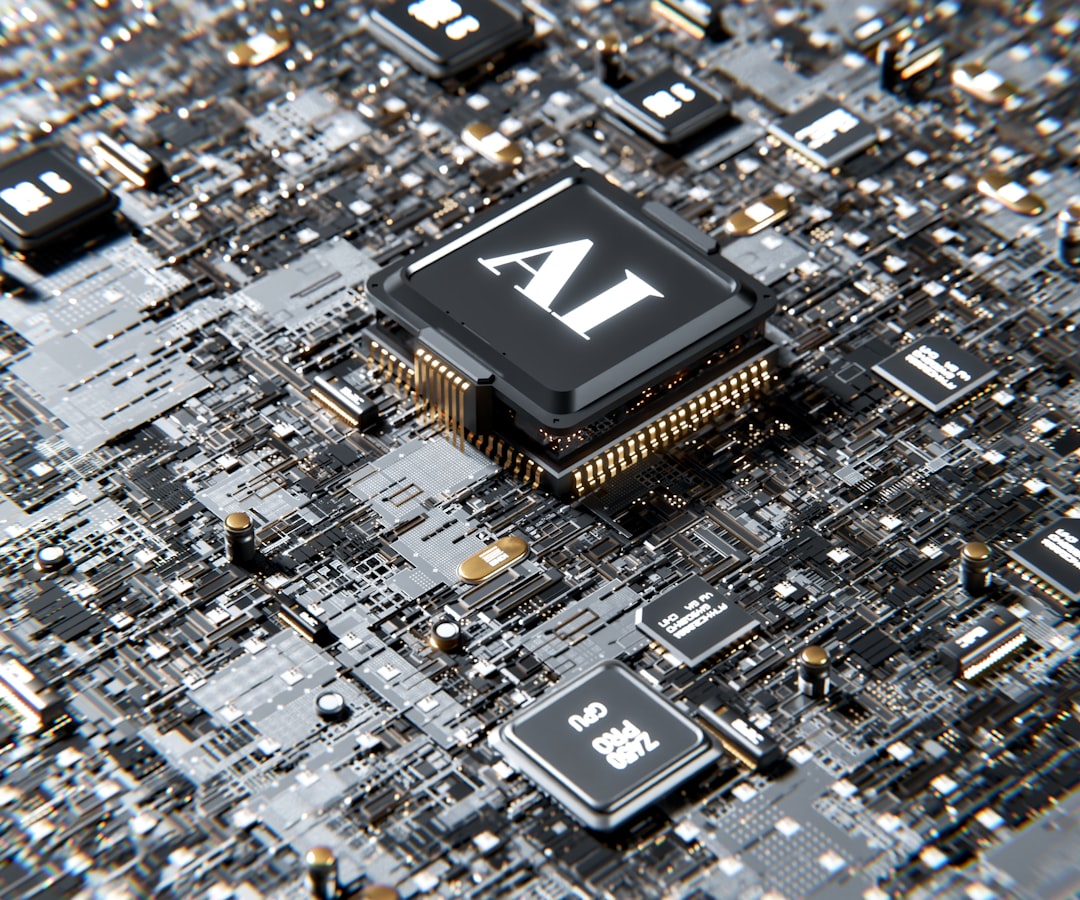
The ability to seamlessly integrate a range of specialized AI models is crucial for enhancing the adaptability and problem-solving capabilities of systems like HuggingGPT. This approach, which relies on the diverse collection of models within the Hugging Face ecosystem, allows AI to tackle complex tasks across various data types and domains. The real-time selection and utilization of the most suitable models for each task enhances flexibility, allowing the system to dynamically adjust to changing needs. This continuous integration of expert models provides users with a more responsive and versatile AI experience. While the benefits of such dynamic integration are undeniable, challenges remain. Coordinating the interactions of multiple models can be intricate, requiring careful management to maintain consistent and reliable outputs. Furthermore, the computational resources needed to support this flexibility can be considerable. Consequently, striking a balance between enhanced capabilities and the operational complexity associated with a vast and constantly evolving model network is a key concern in the future development of HuggingGPT.
Integrating diverse AI models into HuggingGPT offers a path towards greater flexibility and adaptability in tackling a wider range of tasks. This continuous incorporation of specialized models allows HuggingGPT to readily adopt improvements and updates, making it more responsive to the evolving landscape of AI capabilities. The ability to dynamically switch between these models is quite impressive and mirrors the way humans adapt to different situations. It's like having a team of expert AI specialists available on demand to deal with specific parts of a problem. This dynamic approach helps optimize task completion and highlights the potential for a collaborative AI ecosystem.
However, this flexibility comes at a cost. The computational requirements to manage a variety of models can be substantial, prompting questions about balancing complexity and practical usage. Further, while these models work remarkably well in many situations, they still struggle with tasks that require deep, nuanced understanding of the world like we have as humans. It's a fascinating challenge to see if we can improve AI's grasp of human experiences and common sense within this architecture. Another significant challenge is reducing biases. While including diverse models helps to some degree, we have to be vigilant in monitoring the training data of individual models so we don't accidentally introduce biases or magnify existing ones.
HuggingGPT uses smart techniques to choose the best model for each sub-task. It considers things like the model's past success on related tasks to make an educated decision. This approach is a big reason why it is able to perform so well. The merging of results across multiple models brings its own set of problems, though. It's not always easy to blend the meanings extracted from text, images, and sounds into one consistent message. Moreover, there's a risk that it could get too specialized in the kind of data it's been trained on and lose the ability to generalize well to different situations (overfitting).
This concept of constantly adding new models and capabilities opens the door to many important research questions. How can we ensure that adding new models doesn't make the system unstable? How can we effectively combine these models without dramatically increasing the computational requirements? And of course, ethical considerations are always crucial: How do we develop a collaborative AI system that promotes fairness and aligns with human values? The future of HuggingGPT and its ability to leverage a broad range of expert models holds exciting potential, but it will also require meticulous attention to detail and continuous innovation to address these growing complexities.
HuggingGPT Bridging AI Modalities for Complex Task Resolution in 2024 - Autonomous Management of Diverse AI Challenges
HuggingGPT's capacity for "Autonomous Management of Diverse AI Challenges" is a significant step forward in 2024. It aims to simplify complex problem-solving by seamlessly integrating multiple AI models, each specializing in different aspects of a task. This self-managing approach ideally reduces the need for human intervention in the process, allowing HuggingGPT to tackle challenges autonomously. The ability to dynamically select the most fitting model for a given sub-task is a key feature of this approach, but also introduces new hurdles. Concerns about the system's operational complexity and computational demands naturally arise as we rely on a growing number of specialized models. It's also vital to ensure the system doesn't inadvertently amplify existing biases within individual models. Furthermore, fostering an ability to understand human-like reasoning and common sense within this autonomous management structure is still a challenge. While HuggingGPT's capacity for autonomous management represents significant progress, it's crucial to continually evaluate its reliability, fairness, and overall capability to ensure its positive contribution to AI advancements. Its ongoing capacity for learning is key for this autonomous management to continue to address new challenges and stay relevant in the future.
HuggingGPT's ambition to autonomously handle diverse AI challenges through model integration presents a fascinating yet complex landscape. Managing the interplay of numerous models within the system introduces significant architectural challenges. Keeping the output consistent and reliable becomes harder with each new addition, which necessitates careful consideration of how to orchestrate their interactions effectively.
While it's great that HuggingGPT can dynamically choose the right AI tool for the task at hand, efficiently assessing model performance in real-time is tricky. It needs sophisticated algorithms to evaluate each model's strengths and weaknesses in various conditions. This real-time assessment process is key to truly achieving the desired flexibility.
The ability to use numerous AI models gives HuggingGPT its adaptability, but it also means it needs a lot of computational resources. This creates a trade-off: how much flexibility do we want, and how much computing power are we willing to dedicate to it? It's a balancing act that will influence how useful and widespread HuggingGPT becomes.
Despite the integration of various data types, a persistent challenge is achieving a level of cross-modal understanding comparable to human perception. HuggingGPT often struggles to grasp nuanced meaning and context when combining text, images, and sound, which can lead to problems when trying to reason across different data modalities.
The effort to reduce bias through diverse training datasets is commendable. However, managing the risk of inadvertently magnifying existing biases becomes more difficult with each integrated model. We need careful monitoring and evaluation to prevent this from becoming a significant problem, especially as these models are used in more and more critical applications.
Continuous updates from the Hugging Face ecosystem provide benefits, but they also introduce the risk of destabilizing the overall performance of HuggingGPT. The system needs safeguards against incompatibility between models. It's like building a structure where each component needs to be perfectly compatible for stability.
Common sense reasoning remains a major gap in current AI models, including those within HuggingGPT. It's not enough to just merge information from multiple sources—we still need to figure out how to imbue these models with a deeper understanding of the world and contextual human experiences.
HuggingGPT's model selection relies on a scoring system based on past performance, but the wide variability in task requirements makes this process imperfect. This can lead to suboptimal performance in certain scenarios, especially those requiring rapid or adaptable responses.
Combining information from various AI modalities into a coherent message presents its own set of difficulties. Harmonizing interpretations across text, images, and audio can be difficult and sometimes result in diluted or inconsistent conclusions. It’s like trying to weave together narratives told in different languages—the meaning can be lost in translation.
The rise of multimodal AI systems like HuggingGPT brings up important ethical questions around transparency, accountability, and fairness. As these systems become more integrated into our lives, especially in sensitive areas, we need to pay more attention to ensuring they are used responsibly and in ways that align with our values. These are vital considerations that we can't ignore as the technology continues to evolve.
HuggingGPT Bridging AI Modalities for Complex Task Resolution in 2024 - Impact on Future AI Applications and Research Directions
HuggingGPT's emergence significantly impacts the future landscape of AI applications and research. It embodies a pivotal shift towards building more comprehensive multimodal AI systems capable of solving multifaceted problems. By effectively integrating a wide array of AI models, HuggingGPT demonstrates the promise of collaborative AI, potentially enabling solutions across language, vision, and audio domains, and pushing the field closer to the goal of artificial general intelligence.
However, this novel approach isn't without challenges. Successfully integrating and coordinating the interactions of diverse AI models is a complex undertaking. Managing the associated computational resources is another key concern. Furthermore, ensuring that ethical considerations are paramount throughout the development process is crucial.
Moving forward, critical questions surrounding AI development arise from HuggingGPT's progress. Can AI systems gain a deeper understanding of common sense and human experience? How can we mitigate the potential for bias amplification within these collaborative AI systems? And how do we strike a balance between the system's desired flexibility and the need for reliable and trustworthy outputs? These questions and many others highlight that the future direction of AI will necessitate continued innovation and careful consideration of the complexities inherent in advanced AI integration. HuggingGPT's influence extends beyond problem-solving; it underscores the need for a sustained focus on navigating the intricate landscape of collaborative and increasingly sophisticated AI systems.
HuggingGPT's capacity to dynamically switch between diverse AI models significantly accelerates problem-solving, particularly in situations demanding immediate action. This ability to adapt on the fly mirrors human adaptability, which could be crucial in applications like real-time decision-making or interactive environments.
However, despite its multimodal integration, a notable challenge remains: bridging the gap in contextual understanding that humans effortlessly achieve. Integrating text, audio, and visual information doesn't automatically lead to comprehensive grasp of the meaning behind these inputs. This emphasizes the complexity of developing AI that leverages multiple data types without losing the subtle nuances that give them context.
Another concern is the potential magnification of biases. While researchers strive to mitigate biases through diverse training data, combining a multitude of models could unintentionally amplify existing biases. This issue demands continuous attention, especially as HuggingGPT is applied to more critical applications.
Furthermore, managing computational resources becomes more challenging as more AI models are incorporated. Striking a balance between enhanced capabilities and operational efficiency is critical for HuggingGPT's future development. A related concern is the possibility of overfitting, where models become too specialized in their training data, leading to potentially poor performance in unfamiliar scenarios.
The sophisticated scoring system HuggingGPT employs to choose the best model for a task is a clever approach, but it can sometimes lead to suboptimal results. The inherent variability of tasks, particularly those requiring rapid adjustments, can hinder its ability to consistently select the ideal model.
The system's intricate design, with its interwoven network of models, brings about new engineering hurdles. Adding new models requires careful consideration of their potential impact on the system's stability. Maintaining seamless integration while preventing performance degradation from new additions is a complex task.
Consistently synthesizing diverse interpretations from text, audio, and images into a unified and clear output presents a significant hurdle. A lack of alignment can dilute the meaning, jeopardizing the reliability of the generated solutions.
As with any powerful AI system, HuggingGPT raises ethical concerns, especially in its applications within sensitive domains like healthcare or autonomous systems. Defining clear standards for accountability and decision-making in such contexts is crucial.
Despite HuggingGPT's impressive capabilities, the ongoing struggle with common-sense reasoning serves as a reminder of the limitations of current AI. This area remains a key focus for future research aimed at emulating human-like cognitive processes.
Ultimately, HuggingGPT stands as a pivotal step in advancing AI's capacity to tackle increasingly complex problems. But, as this innovative system continues to evolve, researchers need to carefully address the emerging challenges related to bias, computational resources, model integration, and ethical considerations to fully realize its transformative potential.
More Posts from transcribethis.io: