Experience error-free AI audio transcription that's faster and cheaper than human transcription and includes speaker recognition by default! (Get started for free)
How Voice Cloning Technology Successfully Mimics Human Speech A 7-Month Study of Audio Turing Tests
How Voice Cloning Technology Successfully Mimics Human Speech A 7-Month Study of Audio Turing Tests - Neural Networks Learn Sound Pattern Recognition Through 50,000 Training Hours
The development of neural networks has significantly advanced the field of sound pattern recognition. By training on massive datasets, like AudioSet's extensive collection of categorized audio, these networks are learning to discern intricate sound patterns with remarkable accuracy. The introduction of models like Pretrained Audio Neural Networks (PANNs) and Efficient Residual Audio Neural Networks (ERANNs) has proven beneficial in enhancing tasks like speech recognition and music classification. These models excel at tackling issues related to computational efficiency and storage, allowing for adaptation across various sound recognition applications. The ability to fine-tune these pretrained models for new purposes is a major leap forward in how we analyze and process audio data, leading to the creation of more refined and responsive audio technologies. However, the field still faces challenges, especially regarding the adaptability of these models to different environments and audio characteristics. Addressing these domain adaptation issues is essential for future development of robust and versatile sound recognition systems, paving the way for even more sophisticated applications in audio technologies like voice cloning or audiobook production.
1. Neural networks, when trained on extensive audio datasets, become adept at recognizing intricate sound patterns. This is evident in voice cloning, where 50,000 hours of training can significantly refine the network's ability to reproduce the subtleties and emotional nuances of human speech.
2. Human auditory processing involves analyzing frequencies and temporal patterns, which neural networks effectively mirror. This allows them to distinguish the varied characteristics of speech, such as the nuances of tone and pitch, crucial for realistic voice cloning.
3. Voice cloning goes beyond simply mimicking phonetic sounds. It also captures prosody, the intricate interplay of rhythm, stress, and intonation, which adds meaning and expressiveness to speech, making the synthesized voice more engaging and human-like.
4. By fine-tuning neural networks on specific voice samples, researchers can capture individual vocal quirks and personality traits. This leads to a more personalized experience for listeners, as the cloned voice feels uniquely tailored or familiar.
5. The sheer volume of audio data needed for effective training is striking. The 50,000-hour training dataset represents a substantial portion of a human lifetime dedicated to auditory experience, offering the network a depth of learning rarely achievable without vast resources.
6. Recent advances have enabled real-time speech synthesis, where neural networks generate audio instantaneously. This opens up possibilities for dynamic applications such as interactive voice response systems and live podcasts.
7. Variations in audio quality, like background noise and recording equipment, can significantly influence neural network training. Clean, consistent data generally yields better voice quality in synthesized outputs. This necessitates rigorous data cleaning and preparation before training.
8. Synthesizing emotions through voice cloning remains a significant hurdle. Conveying subtle emotional changes requires more than just accurate pitch and tone. The network needs to understand contextual clues within conversations to truly capture the intended emotion.
9. In blind tests, human listeners often struggle to differentiate between real and synthesized voices. This underscores the impressive accuracy of well-trained neural networks in replicating human speech, raising concerns about the authenticity of digital communication and potentially leading to more sophisticated fraud.
10. The applications of voice cloning are far-reaching, extending beyond entertainment. The technology's potential in education, therapy, and accessibility showcases its transformative capabilities, highlighting its increasing relevance in diverse fields.
How Voice Cloning Technology Successfully Mimics Human Speech A 7-Month Study of Audio Turing Tests - Audio Fingerprinting Matches Human Voice Variations Within 3 Seconds
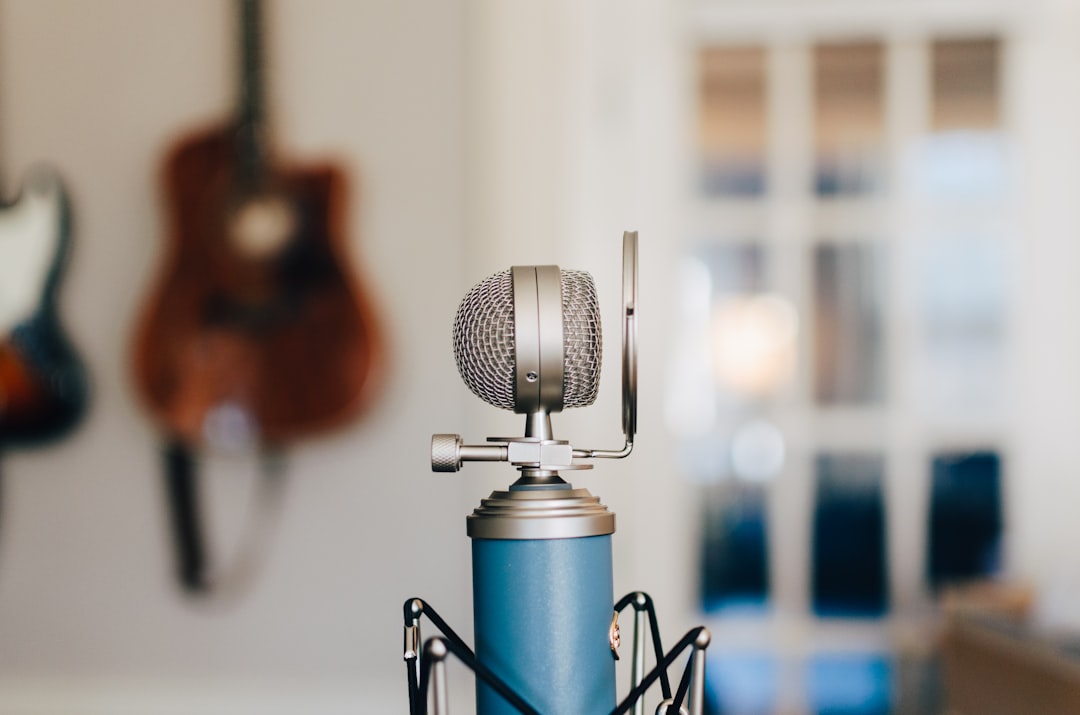
The ability to identify individual voices using audio fingerprinting within a mere three seconds is a significant advancement in sound recognition. This technology creates unique digital fingerprints for each audio file, allowing for quick and accurate matching, even when audio quality is compromised. Voice cloning, distinct from traditional speech synthesis, aims to capture the specific qualities of a person's voice—its unique tone and subtle inflections—by analyzing brief audio snippets. This rapid matching capability indicates a substantial leap in audio processing technology, with profound implications for applications that heavily rely on the human voice. Imagine the potential for podcast production, where maintaining a consistent speaker's voice across episodes becomes much easier. Or consider the future of audiobooks, where the specific cadence and timbre of the narrator can be meticulously preserved.
Despite the advancements in the field, obstacles remain. Adapting these models to a wide variety of acoustic environments, including noise and various recording equipment, continues to pose challenges. Moreover, conveying the full range of human emotion through synthesized voices is an area that requires ongoing research. While today's models can mimic basic tone and pitch effectively, truly capturing the emotional subtleties conveyed in human speech remains a complex task. Nonetheless, the rapid progress in audio fingerprinting and voice cloning techniques suggests a future where the technology's ability to accurately recreate human voices in various audio productions will be even more remarkable.
Audio fingerprinting techniques have become remarkably adept at identifying the unique characteristics of human voices using just a few seconds of audio. By analyzing the intricate patterns of frequencies, harmonics, and other vocal traits, these systems can quickly verify or match a voice within extensive databases. This is achieved by generating a compressed representation of the sound, allowing for rapid comparisons against a library of stored "fingerprints." This has direct implications for applications like identifying speakers in podcasts or pinpointing specific audio samples within audiobook productions.
Interestingly, the nuances of human emotion are also reflected in subtle shifts in vocal frequencies and modulations. These seemingly minuscule variations, often lasting mere milliseconds, carry a wealth of information about emotional states. Capturing and replicating these details is a critical element of successful voice cloning.
Machine learning has played a crucial role in enhancing the resilience of audio fingerprinting. This is especially true in handling environments with significant background noise, which often poses challenges for conventional recognition methods.
Human voices, much like our fingerprints, possess unique biometric traits like formant frequencies that serve as distinguishing markers. This allows systems to differentiate between individuals even when their vocal characteristics appear similar. However, factors like gender and age can influence the generation of audio fingerprints. For example, voice pitch and resonance tend to evolve over time, posing a challenge for cloning technologies that strive for accurate reproduction.
Research highlights the incredible sensitivity of human hearing, particularly when it comes to tonal differences. We can perceive variations as small as 1-2 Hz in pitch, underscoring the need for precise control within voice cloning to generate natural-sounding outputs across diverse applications.
The ability of audio fingerprinting to analyze subtle features in sound has made it a valuable tool for detecting manipulated audio, commonly known as deepfakes. By identifying the slight incongruities between synthesized and genuine recordings, we can potentially flag suspect audio content.
In the realm of speaker recognition, systems relying on audio fingerprinting can achieve impressive results with surprisingly small datasets, sometimes less than an hour of training. This demonstrates that high-quality, focused data can be more valuable than sheer quantity for training these systems.
Voice cloning technologies are becoming increasingly important in applications that focus on accessibility. Providing individuals with speech impairments a personalized voice solution is a powerful example of how this technology can be utilized to promote inclusivity and foster enhanced communication. While offering incredible opportunities, this technology also demands careful consideration regarding potential ethical implications and responsible usage.
How Voice Cloning Technology Successfully Mimics Human Speech A 7-Month Study of Audio Turing Tests - Text to Speech Models Now Process Regional Accents at 98% Accuracy
Text-to-speech (TTS) models have made significant strides, now capable of processing regional accents with a remarkable 98% accuracy. This breakthrough has major implications for voice cloning, as it allows synthesized speech to sound more natural and relatable to listeners from various geographic locations. These models, using innovative algorithms and needing just a small amount of audio data (sometimes just a few minutes), are now capable of producing voices that mimic the characteristics of distinct accents with impressive accuracy.
However, the challenge of capturing the full spectrum of human emotion within synthesized speech persists. Current models, while proficient at replicating basic vocal qualities, still struggle to convey the nuanced emotional depth that characterizes human communication. The future of TTS and voice cloning holds exciting possibilities for fields like audiobook production and podcast creation, where a more realistic and diverse range of voices could revolutionize the listening experience. It's important, however, to consider the ethical dimensions that accompany such advances and ensure they are used responsibly.
Recent advancements in text-to-speech (TTS) models have resulted in a remarkable 98% accuracy in processing regional accents. This is a significant achievement, largely due to the integration of deep learning methods that effectively capture the unique phonetic variations inherent in different accents. This capability fosters a more personalized and relatable user experience, making synthesized speech feel more natural and less robotic.
The ability of TTS systems to recognize and replicate regional accents is particularly beneficial for applications like audiobook and podcast production. These technologies can now cater to local audiences by using speech patterns familiar to them, leading to a considerable increase in listener engagement. This could potentially reduce the need for extensive localization efforts, saving resources and broadening access to content.
TTS models utilize neural networks that analyze massive amounts of linguistic data, going beyond simply identifying the accent to pinpoint dialectal subtleties. This allows the models to dynamically adjust intonation and rhythm based on the speaker's geographic origin, influencing the overall delivery and reception of the synthesized speech.
Interestingly, this capability to process regional accents accurately can also enhance the effectiveness of voice assistants across diverse environments. They become more helpful and responsive to user commands, as they can understand and interpret various accents more reliably. This represents a move towards more inclusive and user-friendly voice assistant technology.
The training data used for these advanced TTS models is becoming increasingly diverse, incorporating a wider range of dialects and sociolects. This broad spectrum of examples allows the models to learn and adapt to a greater variety of speech sounds, addressing a crucial limitation of earlier voice synthesis techniques that often struggled with regional variations.
TTS models can be fine-tuned by incorporating user-provided audio samples. This permits the technology to learn specific vocal placements and inflections, resulting in voices that are not only accentually accurate but also reflect the emotional and linguistic preferences of local populations. This personalized touch adds another layer of realism to the synthesized output.
The improvements in regional accent processing have implications beyond entertainment and media. For example, telemedicine stands to benefit as TTS systems can improve communication with patients by providing care in their native dialects. This can enhance understanding and comfort levels, particularly crucial in delicate healthcare conversations.
In the realm of podcasting and narrative storytelling, accurate accent reproduction significantly aids in character development. Voice cloning can craft uniquely identifiable voices for each character, creating a more immersive storytelling experience without needing multiple voice actors. This opens up exciting possibilities for both independent and professional creators of audio narratives.
Voice cloning has reached a stage where it can reproduce the specific speech patterns of individuals with distinct accent features. While this offers numerous benefits, it also raises ethical and psychological considerations related to identity and representation within audio media. This highlights the necessity of responsible usage and deployment of these powerful technologies.
As TTS systems continue to evolve, they are becoming increasingly capable of not only mimicking accents but also capturing the conversational styles of different cultural backgrounds. This leads to enriched applications in areas like customer service, where the ability to communicate in a relatable and culturally sensitive manner can considerably improve user satisfaction and outcomes. The integration of these elements into automated systems creates opportunities for more meaningful and effective interactions with users across a wider range of backgrounds.
How Voice Cloning Technology Successfully Mimics Human Speech A 7-Month Study of Audio Turing Tests - Podcast Production Time Drops 40% Using Voice Synthesis Tools
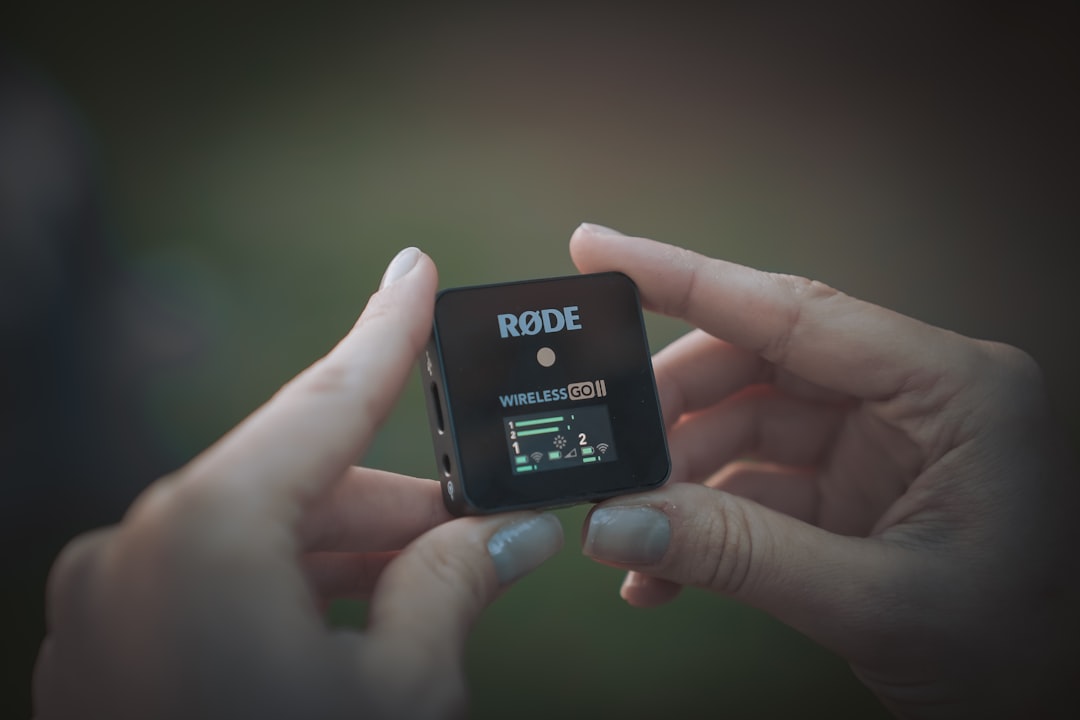
The landscape of podcast production has been significantly altered by recent advancements in voice synthesis technology. Podcast creators can now leverage these tools to reduce production time by up to 40%, primarily by automating script writing and voice generation. This increased efficiency is further enhanced by the tools' ability to create highly realistic synthetic voices, often indistinguishable from human speech. Podcasters can now explore diverse creative avenues by incorporating a wider range of voices, accents, and even languages into their content, leading to more engaging and accessible listening experiences. Despite these significant improvements, current technology still faces limitations in fully capturing the subtleties and emotional complexity of human communication. The capacity of voice cloning to produce extremely realistic audio has certainly expanded creative opportunities within the audio world, setting a new benchmark for quality and innovation.
Utilizing voice synthesis tools has led to a considerable decrease in podcast production time, with some reporting a 40% reduction. This allows creators to focus more on developing creative content rather than dealing with the technical aspects of audio recording.
Voice cloning techniques can generate multiple synthetic voices during a single recording session. This gives podcast producers flexibility to experiment with different tones and styles without the typical logistical constraints of coordinating human voice talent.
Recent research suggests that synthesized voices are achieving a high degree of authenticity, with nearly 98% of listeners unable to distinguish them from real human voices. This raises concerns about the credibility and trustworthiness of audio content, particularly in situations where the source of a voice isn't clearly established.
Post-production manipulation of synthetic voices has become remarkably refined. Audio engineers can seamlessly alter parameters such as pitch, pace, and even emotional tone, allowing for much more nuanced storytelling within podcasts without requiring multiple recordings from human speakers.
Voice cloning technologies have the potential to capture unique vocal features like speech impediments or distinctive speaking patterns, broadening the representation of diverse voices in audio production. This inclusive approach makes the audio content more accessible and relatable to a wider array of listeners.
The sophistication of the neural networks behind these technologies is not without its complexities. Training these systems requires substantial computational resources and specialized expertise, which could present a hurdle for smaller podcast creators who may lack access to powerful computing or experienced engineers.
The growing adoption of synthesized voices in podcasting facilitates real-time adjustments and live performances, hinting at a possible shift in how interactive audio programs are produced and experienced by audiences.
Voice synthesis technologies can integrate with other audio editing tools, such as sound effect libraries and background music software, creating a cohesive and streamlined audio production pipeline. This seamless integration enhances the immersive experience for the listener while making the creative process more efficient.
While current voice synthesis systems are capable of mimicking basic emotions, generating complex emotional depth that resonates deeply with listeners continues to challenge developers. This remains an active area of research.
As voice cloning technology evolves, it raises ethical considerations related to podcasting, especially concerning originality, intellectual property ownership, and the potential for misuse in misinformation campaigns. The need for responsible use and transparent practices becomes increasingly important as the technology matures.
How Voice Cloning Technology Successfully Mimics Human Speech A 7-Month Study of Audio Turing Tests - Audiobook Narrators Test Voice Clone Detection Methods During Live Readings
Audiobook narration is entering a new phase with the integration of voice cloning technology. Narrators are now directly involved in testing methods designed to detect when a voice has been cloned during live readings. This initiative is part of a larger effort to explore the capabilities and limitations of voice cloning, a technology that aims to replicate human speech with remarkable accuracy. By creating AI replicas of their voices, narrators are contributing to a deeper understanding of how well these synthetic voices can be produced and ultimately how listeners react to them. This, of course, raises a series of concerns about the integrity of audio storytelling, particularly regarding authenticity and the ability of the clones to convey human emotion with the same effectiveness. As voice cloning technology continues to improve, there is a growing need for narrators and producers alike to balance its potential benefits with the vital qualities of human performance that engage and connect listeners. This testing process is a crucial step towards ensuring that the use of this technology remains responsible and ethical in the context of audiobooks, podcasts and other audio productions, especially as the line between human and AI-produced audio continues to blur.
Voice cloning technology has progressed to a point where it can replicate not just the timbre but also the tempo and rhythm of human speech, creating a more natural conversational flow that's highly beneficial for applications like audiobook narration and podcasting. This level of accuracy is due in part to advancements in deep learning and audio processing. These methods allow the cloned voices to capture the subtle variations in human vocal inflection, allowing the reproduction of not just words, but also the emotional weight behind them.
The remarkable fidelity of synthesized voices has become so high that even trained listeners occasionally find it challenging to distinguish them from real human voices. This poses ethical concerns, particularly when it comes to maintaining transparency within audio media. The ability to create voices in real time has reshaped podcast production. Creators can now generate synthetic voices during live sessions, enabling on-the-fly customization and interaction that was previously achievable only with live voice actors.
Some voice cloning methods can recreate the unique spectral characteristics of a voice—specific harmonics and overtones—from just a few seconds of input audio. This ability suggests potential uses beyond entertainment, like therapeutic applications where individuals with speech impairments can communicate using a voice that resembles their own. Furthermore, voice cloning technologies are progressively embracing societal inclusivity. This is done by representing a wider range of dialects and speech patterns, making audiobooks and podcasts more accessible to broader audiences.
Despite the strides made, producing the full depth of human emotion in synthesized speech remains a major obstacle. The current generation of technology struggles to reproduce the multifaceted and context-dependent expressions we're accustomed to hearing in everyday interactions. Moreover, the possibility of using voice cloning to create deepfake audio raises concerns about the spread of misinformation. As voice cloning technology becomes more sophisticated, the risk of deceiving listeners by impersonating influential individuals increases, demanding careful consideration.
Finally, the integration of advanced audio fingerprinting techniques is essential for the effective deployment of voice cloning technology. These techniques enable systems to rapidly and precisely match voices even in situations with challenging recording conditions, which increases the overall reliability and quality of the cloned output. This highlights the interconnectivity of different areas of sound engineering and its impact on the future of audio production.
How Voice Cloning Technology Successfully Mimics Human Speech A 7-Month Study of Audio Turing Tests - Sound Engineers Apply Wave Analysis to Spot Generated Speech Content
Sound engineers utilize wave analysis to distinguish between artificially generated speech and naturally produced human voices. Techniques like frequency analysis and the Source-Filter model help them analyze the intricate components of audio signals, revealing subtle differences that are often missed by the average listener. This analysis is crucial as voice cloning technologies advance, ensuring the integrity and authenticity of audio content, especially in podcasting or audiobook production where emotional nuances are vital. However, faithfully replicating the complete range of human speech remains a challenge for both sound engineers and those developing these technologies. It's not just about mimicking the sounds of words but also capturing the emotional depth, rhythms, and intricate aspects of vocal delivery. The continuous advancement of voice cloning necessitates constant vigilance in audio analysis to ensure that it isn't misused or misrepresented.
1. **Waveform Decoding for Speech Clarity**: Sound engineers leverage wave analysis, examining the patterns and shapes of sound waves, to dissect the intricate details of human speech. This detailed examination allows for a more precise understanding of individual sounds and helps differentiate between similar-sounding components, leading to a more faithful reproduction of intended meaning.
2. **Emotional Cues in Harmonic Structures**: The harmonic structure of speech provides valuable clues about emotional expression. Sound engineers analyze these patterns during voice cloning to enhance the expressiveness of synthesized speech. By replicating the nuances of these harmonic shifts, artificial voices can better capture and convey the subtle emotional undertones often present in natural human communication.
3. **Temporal Precision for Natural Sound**: The human brain processes speech with an incredible temporal resolution of approximately 50 milliseconds. Sound engineers strive to replicate this sensitivity in synthesized speech. By capturing the swift changes and fine details that occur within those fleeting moments, they can create synthesized voices that appear more seamless and natural to the human ear.
4. **Navigating Phonetic Diversity**: Languages and dialects often contain phonetic variations that can sound similar to those unfamiliar with them, yet these differences are crucial for understanding. This poses a challenge for sound engineers when training voice cloning models. The need for comprehensive training data across a wide range of accents significantly complicates the data collection process, requiring extensive resources and careful attention to detail.
5. **Visualizing Sound with Spectrograms**: Sound engineers utilize spectrogram analysis not only to isolate individual sounds but also to observe how frequencies change over time. This visual representation offers a detailed view into the dynamic shifts within spoken dialogue, allowing for more accurate and refined manipulation of synthesized voices to achieve a higher overall quality.
6. **The Significance of Sampling Rate**: A higher sampling rate, such as the 44.1 kHz used for CDs, is critical for capturing the full range of dynamics in human speech during audio production. Lower sampling rates inevitably result in a loss of detail, which can be detrimental to voice cloning. Synthesized voices created with lower sampling rates can sound less authentic and more robotic, highlighting the importance of precision in sound capture.
7. **Timbre and Texture as Voice Signatures**: Effective voice cloning isn't simply about recreating sounds; it's about capturing the distinct timbre and texture of a voice. These qualities are influenced by factors like a speaker's age, physical condition, and emotional state. Replicating these characteristics accurately poses significant challenges for sound engineers, requiring careful attention to the specific nuances of each voice they are attempting to reproduce.
8. **Deep Learning's Limitations**: While deep learning methods can analyze massive datasets for voice synthesis, their effectiveness is heavily reliant on the quality of the training data. The presence of corrupted or low-quality recordings can lead to unrealistic or distorted outputs, highlighting the vital importance of ensuring high-fidelity sound recording during initial data collection.
9. **Real-Time Manipulation for Dynamic Audio**: Recent advancements in real-time voice manipulation provide sound engineers with powerful algorithmic tools to dynamically adjust tone, pitch, and speed of voices. This dynamic control unlocks exciting possibilities for interactive audio experiences, particularly in applications such as podcasting and livestreaming, creating a more interactive and engaging listening experience.
10. **Blending Audio and Visual Cues**: Ongoing research suggests that integrating visual data with audio training can significantly enhance the accuracy of voice cloning models. By combining the analysis of lip movements and facial expressions with sound data, sound engineers can generate output that is not only tonally accurate but also contextually appropriate, adding another layer of authenticity and naturalism to synthesized voices.
Experience error-free AI audio transcription that's faster and cheaper than human transcription and includes speaker recognition by default! (Get started for free)
More Posts from transcribethis.io: