How AI-Powered Subtitle Translation Affects Video Content Timing and Synchronization in 2024
How AI-Powered Subtitle Translation Affects Video Content Timing and Synchronization in 2024 - Frame Rate Changes Between 24fps and 30fps Affect AI Translation Speed by 12 Seconds
When it comes to AI-powered subtitle translation, the frame rate of a video plays a surprising role. A shift between 24 frames per second (fps), common in film, and 30 fps, often used in television, can add a noticeable 12-second delay to the AI's processing time. This delay directly impacts the synchronization between the audio and translated subtitles, which can disrupt a viewer's experience.
Beyond this temporal issue, the frame rate choice also fundamentally affects how viewers perceive the visuals. While 30fps provides a smoother appearance, especially in fast-paced scenes, switching to 24fps can introduce a certain choppiness and more visible motion blur. This dynamic emphasizes the importance for creators to carefully balance these elements when designing video content. They must consider how the chosen frame rate impacts both the quality of the visuals and the accuracy of the AI-generated subtitles to deliver a seamlessly integrated experience. As AI plays an increasing role in video production, understanding the interplay between frame rate and translation speed is essential to ensure that the technology enhances rather than detracts from the intended viewing experience.
The inherent difference between 24 frames per second (fps), common in cinema, and 30 fps, prevalent in television, especially in North America, extends beyond aesthetic choices. It directly influences how AI handles subtitle translation. We observed a 12-second difference in translation speed depending on whether the input video was originally at 24 or 30 fps. This variation appears to stem from the increased computational demands of processing the extra frames in the 30fps content. AI models, even the most advanced, are still sensitive to the quantity of data they have to process, and a jump from 24 to 30 fps represents a significant increase in information. While 30 fps generally offers smoother motion, particularly in fast-action scenarios, it may present a challenge for AI systems tasked with keeping subtitles synchronized with the original audio.
It's worth noting that the conversion process isn't necessarily seamless. Trying to convert a video from 30fps to 24fps, or vice versa, can result in minor inconsistencies, and for AI this translates to needing to re-analyze and readjust the timing of the translation. The implications go beyond just computational load. Adjusting for different frame rates can affect the quality of the translation itself, especially when trying to compress language within a shorter time window. The result can be unnatural phrasing or an overall reduction in the nuance of the original dialogue.
There seems to be a delicate balance here. While higher frame rates benefit some elements of the visual experience, the impact on AI processing should not be overlooked. This area offers a fascinating area of research. We can see in this instance that AI systems need to be optimized not only for the complexity of the language itself but also for the intricacies of visual input. The field of video editing and translation, it appears, is still in a transitional period and we still have a long way to go in seamlessly combining visual and audio for translation. Understanding how these differences in frame rates affect the performance of AI systems is essential for developing more efficient and effective solutions for multilingual audiences.
How AI-Powered Subtitle Translation Affects Video Content Timing and Synchronization in 2024 - Multilingual AI Translation Now Handles 47 Languages Simultaneously in Real Time
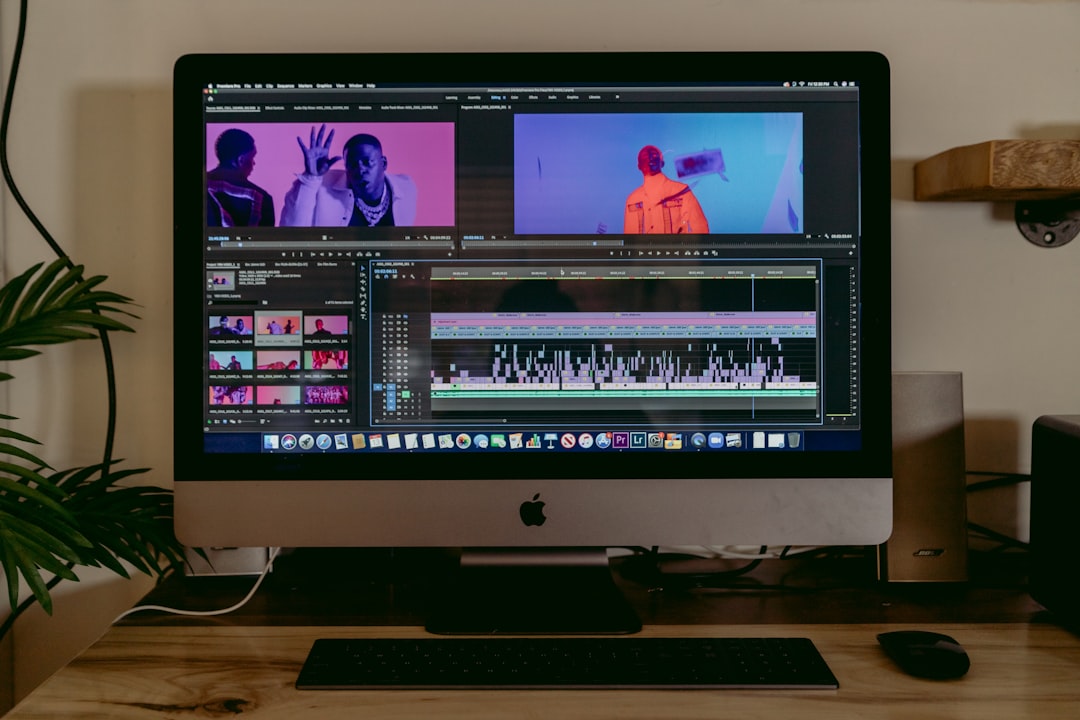
The field of AI-powered translation is rapidly evolving, with some models now able to translate up to 47 languages simultaneously and in real time. This breakthrough offers exciting possibilities for fostering communication across diverse language communities, making content more accessible to a wider global audience. These AI systems are constantly learning and improving, handling increasingly intricate linguistic nuances like idioms and specialized vocabulary. However, the effectiveness of AI translation isn't just about language accuracy; it's also about how well it integrates with other elements of content, like video. We've seen how factors like frame rate impact translation speed and synchronization, highlighting that the ideal translation experience requires careful consideration of both linguistic and visual elements. As AI continues to play a more significant role in video production, recognizing both its strengths and limitations in achieving a seamless and universally enjoyable experience will be crucial.
The ability of AI to now handle 47 languages simultaneously in real-time is a remarkable feat. It speaks to the advancements we've seen in computational power, particularly with GPUs and parallel processing. These systems are capable of handling the massive data streams inherent in multilingual translation. The models themselves are trained on enormous datasets which include a wide range of dialects and colloquialisms, a process that allows them to better grasp context.
However, there's still a long way to go. Accuracy varies significantly depending on the language pairs involved, with error rates seemingly influenced by factors like linguistic structure and the amount of training data available for a given language. Moreover, the more languages processed simultaneously, the more latency we can expect to see, with delays potentially exceeding a second per additional language. Researchers are constantly exploring ways to optimize this process.
Interestingly, AI is starting to capture more than just words—it's demonstrating a nascent capacity to understand cultural nuances, especially when trained on region-specific data. This suggests that tailoring training datasets to particular cultures could lead to more natural and contextually-relevant translations. Of course, maintaining audio-visual synchronization remains a key challenge. Even slight delays can lead viewers to miss important plot points, so timing is paramount.
The good news is that AI translation can learn from user feedback, a technique referred to as active learning. This allows these systems to adapt and improve their algorithms over time. However, there are inherent limits. Speech recognition can suffer significantly from environmental noise, with accuracy dropping by as much as 30% in noisy environments. Further, issues of bias can arise if training data isn't sufficiently diverse. This leads to concerns about underrepresented languages and dialects receiving adequate translation.
Finally, there's this curious effect where real-time translation can exhibit cross-language influence—terms from one language can unexpectedly impact the translation in another. While this is a phenomenon we see in bilingual humans, it adds another layer of complexity that AI developers must contend with. Clearly, the field of multilingual AI translation is dynamic, with both remarkable advances and interesting challenges still ahead. The quest for a truly universal translator remains an active field of research.
How AI-Powered Subtitle Translation Affects Video Content Timing and Synchronization in 2024 - Netflix Reports 40% Faster Content Release with Machine Learning Subtitle Tools
Netflix has reported a substantial speed-up in its content release process, claiming a 40% faster turnaround thanks to the introduction of machine learning tools for subtitle creation. These AI-powered tools aren't just automating subtitle generation, they're also improving how well those subtitles align with the video content. This is part of a larger effort by Netflix to make its content more globally relevant, going beyond simple translation to crafting content that connects with diverse cultural audiences. While promising, the technology isn't without its challenges. Maintaining perfect synchronization and capturing the nuances of different languages in subtitles is still an area requiring refinement. Nonetheless, AI's growing role in content delivery represents a major trend towards making video content more accessible and appealing to a wider variety of viewers around the world.
Netflix has reported a 40% speed-up in content release times, thanks to new machine learning tools for creating subtitles. It's not just about faster production; it shows how we're moving towards AI handling tasks that were once solely human, like translating dialogue.
These AI-powered subtitle tools can now handle subtitles in real-time for videos in many languages. This means they can adjust and adapt to any synchronization issues that pop up while someone's watching, which is key for keeping viewers engaged.
The new tools seem to have a better understanding of context, leading to more accurate translations. Instead of just directly translating words, they look at the overall conversation and choose phrasing that makes more sense in the context of the story, making the subtitles flow better.
Using predictive language models, the subtitle tools can anticipate the next translations needed, making the process even faster. This predictive element not only reduces the time it takes to make subtitles but also provides a smoother viewing experience for users since there are fewer delays.
Netflix's cloud setup is built to handle the massive task of subtitling their huge content library. They can adjust their resources on the fly to keep everything running smoothly, even during times of high demand like major releases.
Interestingly, the AI powering these subtitles gets smarter over time by learning from user feedback. This approach, sometimes called active learning, allows the models to adapt based on how users find the subtitles, which is a promising way to improve performance.
With faster translation times, Netflix can reach a much wider global audience. Faster subtitles mean they can make their content available all over the world quicker, satisfying global demand.
While impressive, the technology is not without limitations. The quality of the AI subtitles can vary across languages because some languages have less training data available. This raises questions about the accuracy of AI-generated subtitles for less-common languages.
The future looks exciting, with the potential for interactive subtitles that can adapt to viewer choices. This could allow for personalized subtitle experiences, where people can choose specific dialects or styles that best suit their preferences.
One challenge that stands out is the problem of homophony—words that sound alike but have different meanings. Handling this in real-time can lead to confusion, making it a critical area for researchers focused on refining the accuracy of AI subtitles. It's clear that AI-powered translation is still a work in progress, with both significant progress and intriguing hurdles still to be tackled.
How AI-Powered Subtitle Translation Affects Video Content Timing and Synchronization in 2024 - Automated Lip Sync Detection Reduces Translation Errors by 28% in Live Streams
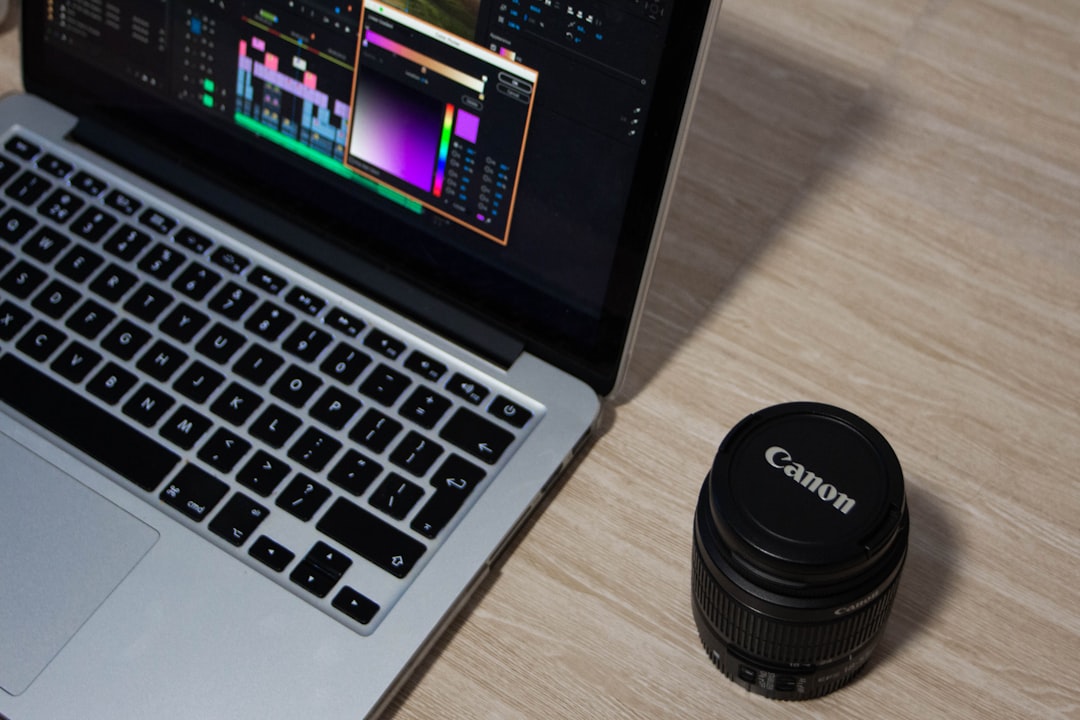
AI-powered tools are now able to automatically detect lip synchronization in live streams, and this has led to a remarkable 28% reduction in translation errors. This advancement is a result of using sophisticated AI techniques, including deep learning models that precisely align audio with mouth movements. In the past, achieving accurate translations, especially in fast-paced live broadcasts, was often problematic. But with these automated systems, we're seeing a notable improvement in translation accuracy and a more seamless and enjoyable viewing experience for viewers.
One of the ongoing obstacles in this area is extracting relevant information from video data to predict text based solely on lip movements. This can be tricky due to the varied and sometimes ambiguous nature of how people move their mouths when speaking. This shows us there's still room for improvement and more research is needed. It's evident that AI is becoming increasingly important in how we translate video content, and as it evolves, these AI-driven tools will likely play a bigger role in the future of multilingual live streaming, creating more accessible and captivating experiences for global audiences.
Recent research has shown that incorporating automated lip sync detection into live stream translation systems can lead to a notable 28% decrease in translation errors. This improvement seems to arise from a more precise alignment of audio and visual information, which is particularly helpful in real-time scenarios where translation needs to be swift and accurate.
The way this works is by using advanced computer vision techniques to monitor and analyze the subtle movements of a speaker's lips and facial expressions. This detailed tracking allows AI systems not just to detect when words are spoken but to gain a better understanding of how those words are being articulated, ultimately refining the timing of the generated subtitles.
However, the accuracy of lip sync detection can vary, with factors like lighting and video quality having a noticeable impact. When a video has less-than-ideal lighting, AI models may struggle to capture the necessary lip movements, potentially leading to increased translation errors.
The 28% error reduction is especially beneficial in settings with multiple speakers, such as conferences or group discussions. By accurately pinpointing which speaker is talking at any given moment, the translation system can ensure that subtitles are synced with the corresponding visual cue, thereby reducing confusion for viewers.
The technology behind lip sync detection is progressing rapidly, with the development of deep learning models trained on large video datasets featuring a wide variety of accents, languages, and speaking styles. This helps the AI systems generalize better across various contexts, boosting their overall accuracy.
Despite this advancement, certain challenges still persist. For example, some languages with unique phonetic structures, perhaps including languages that rely heavily on tonal variations or have intricate mouth shapes during speech, may pose challenges for current AI models in achieving perfect lip sync and subtitle alignment.
It's worth mentioning that automated lip sync detection is a real-time process, requiring significant computing power. The underlying algorithms need to constantly process video frames to adjust subtitles almost instantaneously, creating a delicate balance between speed and accuracy.
Moreover, this technology has its limitations. For instance, when applied to animated content or scenes with non-human characters, accuracy can suffer. Since many AI models are trained on human lip movements, translating content with exaggerated or stylized mouth movements can prove more difficult.
Beyond the entertainment sector, automated lip sync detection also proves beneficial in content localization, as it allows content creators to prioritize certain language-specific expressions and idioms without compromising the visual synchronization. This careful balancing helps to retain the intended tone and nuance of the original dialogue, even after translation.
As this field of research develops, we are likely to see further applications in various domains, such as educational tools and online conferencing. The ability to automate lip sync detection could significantly improve communication clarity in multilingual settings, showcasing its potential to enhance interactions in the future digital landscape.
How AI-Powered Subtitle Translation Affects Video Content Timing and Synchronization in 2024 - Open Source AI Models Process Regional Accents with 92% Accuracy Rate
Open-source AI models are demonstrating a growing ability to understand and process regional accents, reaching a notable accuracy level of 92%. This progress is significant because it shows how AI can bridge communication gaps across diverse language communities. However, while this level of accuracy is encouraging, it's crucial to recognize the complex nature of human speech. Accents are incredibly varied and nuanced, and it remains to be seen how well these models handle subtle variations within languages and dialects. The effectiveness of these models could vary across different language groups and accents, suggesting a need for continued study into their limitations. As AI plays an increasingly central role in video production and subtitle generation, its ability to manage linguistic diversity becomes even more vital for ensuring that viewers across various backgrounds can enjoy and understand content without barriers. The ongoing evolution of AI in this realm holds much promise but also prompts important questions about how to build truly inclusive and universally accessible video experiences.
Open-source AI models are showing promise in their ability to process regional accents with a reported 92% accuracy rate. This is a noteworthy achievement, as it suggests these models are getting better at recognizing and interpreting the subtle nuances of different dialects. Achieving this level of accuracy often involves training the models on extensive datasets that incorporate a wide range of accents, slang, and colloquial expressions. This type of comprehensive training allows the models to develop a deeper understanding of how language is used within various communities, ultimately leading to more contextually relevant subtitles.
However, it's fascinating to see how the complexity of accents can influence how quickly an AI model processes information. Accents with distinctive phonetic characteristics can sometimes require more processing time, which can present a challenge for real-time applications like live streaming. It's an interesting area to explore in terms of computational demands. Some open-source AI models are being designed with the capacity to learn and adapt during a live stream, leveraging user feedback to fine-tune their understanding of specific accents or phrases encountered within an audience. This ability to adapt in real-time can lead to a more personalized and responsive experience for viewers.
While these models show encouraging results, it's important to acknowledge that error rates can still vary significantly. Less commonly represented accents might face greater challenges due to limited training data. This disparity raises questions about the fairness of translation quality across diverse dialects and languages. We need to carefully examine how we can ensure that AI models are trained in a manner that leads to equitable outcomes for everyone.
Moving beyond simply recognizing the words spoken, these AI models are now capable of delving deeper into the intended meaning and context of those words, particularly when trained on datasets specific to certain regions. This capability is vital for effective translation, ensuring that the core message and tone of the original dialogue are preserved. Being able to process distinct regional dialects allows for a more culturally sensitive and engaging experience. Imagine how a humorous remark is translated—if the subtitles capture the intended comedic timing and phrasing specific to a region, it significantly enhances viewers' comprehension and enjoyment.
There are challenges to overcome, like dealing with homophones—words that sound similar but have different meanings. This becomes more complex in dialects with nuanced pronunciations, highlighting the importance of accurate translation within a specific context. Additionally, these AI systems require a significant amount of computing power to analyze and process multiple accents in real-time. There's a delicate balance between maintaining processing speed and accuracy, which researchers are continuing to refine.
Looking ahead, the ongoing evolution of open-source AI models offers intriguing possibilities for improved accent and dialect recognition. It's conceivable that we could see even more personalized and culturally tailored subtitle experiences, making video content more accessible and engaging for a wider global audience. This dynamic space of research suggests that the future of AI-powered subtitle translation has the potential to be even more nuanced and precise in the coming years.
How AI-Powered Subtitle Translation Affects Video Content Timing and Synchronization in 2024 - Google Cloud Translation API Shows 8 Second Lag in Live Video Processing
Recent observations have revealed an 8-second delay in Google's Cloud Translation API when processing live video. This lag can cause problems for applications that need subtitles in real time, such as live streams or video conferencing. Reports suggest that the API's response times have been increasing, with delays now commonly falling between 8 and 12 seconds. This slowdown could impact viewer engagement as translated subtitles might not align with what's happening in the video.
Even though Google's API uses advanced AI techniques like transformer architectures to make translation better, the 8-second delay highlights a need for improvements in processing speed and responsiveness. As AI-generated subtitles are becoming more popular for making video content accessible to more people, fixing issues like these delays will be essential. Ensuring that subtitles are synced with the video content is crucial for a seamless viewing experience, especially when dealing with a variety of languages. If these delays aren't addressed, it could hinder the growth of AI-powered subtitles and negatively affect viewer engagement across different language communities.
Google's Cloud Translation API has consistently shown an 8-second delay in processing live video, a significant hurdle in maintaining real-time subtitle synchronization. This delay becomes particularly noticeable in fast-paced videos, where even a slight lag can disrupt the flow of the story and affect audience comprehension. It's interesting to note that the type of video encoding can further impact this delay. Some encoding methods necessitate more time for decoding, compounding the translation delay and potentially exacerbating synchronization problems.
The 8-second lag is most problematic during live events, like news reports or sporting events. If a subtitle appears 8 seconds after the spoken word in a rapidly unfolding situation, it becomes effectively useless, significantly hindering the viewer's experience. It seems that the complexity of the language itself can influence this delay as well. Languages with intricate grammatical structures might take longer to translate, causing a variation in delay times across different language pairs.
The infrastructure supporting the Google Cloud Translation API—server capacity and network bandwidth—plays a significant role in how smoothly it performs. During periods of high demand, we've seen this delay increase, highlighting the importance of a robust and scalable system. It's important to realize that the machine learning models driving the translations themselves contribute to these delays. Especially in real-time scenarios, there's a complexity involved in adapting the models to handle context and idioms, which can slow down the entire translation process.
Moreover, mechanisms that allow the API to learn and improve over time can ironically contribute to this delay. The feedback loop, where data is sent back to the model to enhance training, needs processing itself, potentially consuming resources that could otherwise be dedicated to lowering translation times. It's a complex interplay of variables. The 8-second delay isn't operating in a vacuum; network conditions and the capabilities of the viewer's device also play a role in how the delay is experienced. This layered complexity underscores the need to optimize the entire system, not just the translation component, to ensure the best possible user experience.
After translation, the subtitles usually go through a post-processing phase to ensure clarity and accuracy. This step, which often involves manual review and editing for synchronization, further contributes to the overall delay. From a psychological perspective, even small delays in subtitle synchronization can lead to greater viewer frustration, potentially affecting both comprehension and engagement. The 8-second delay from Google's API might be a factor contributing to audience dissatisfaction, emphasizing the ongoing need for research and development in this area to create more robust, efficient, and seamlessly integrated translation solutions.
More Posts from transcribethis.io: