Experience error-free AI audio transcription that's faster and cheaper than human transcription and includes speaker recognition by default! (Get started for free)
How AI Legalese Decoder Transforms EPS Report Analysis A Technical Deep-Dive
How AI Legalese Decoder Transforms EPS Report Analysis A Technical Deep-Dive - Neural Networks Break Down SEC Filing Data Into Plain English Text
Artificial neural networks are proving to be powerful tools for demystifying the dense language found in SEC filings. These networks act as translators, taking the complex legalese often found in these documents and rendering it into plain English. The AI Legalese Decoder, a prime example, utilizes sophisticated natural language processing (NLP) to break down these complex documents. This allows individuals, even without a legal background, to grasp the essence of these important disclosures.
The Decoder's approach involves a combination of machine learning and analyzing vast amounts of data. Not only does this simplify the complex financial and legal terms used, but it also helps users gain a better understanding of the overall context. The ultimate goal is to make this vital financial information more accessible to everyone. As these AI-driven tools develop further, they have the potential to illuminate a space that has traditionally been shrouded in dense, hard-to-understand language. This, in turn, creates more transparent pathways for individuals to access and comprehend the crucial information contained within SEC disclosures and corporate filings.
1. Neural networks are being used to dissect the often convoluted language of SEC filings, converting the dense legal jargon into easily understandable English. This allows a broader audience, including those without a legal background, to grasp the crucial information within these documents.
2. The process utilizes natural language processing (NLP) models, specifically trained to decipher intricate legal syntax and terminology. It represents a significant step forward in the capabilities of machine learning, pushing the boundaries of what AI can accomplish with language.
3. These neural networks are often trained on extensive historical SEC filings, with human legal experts providing annotations to guide the learning process. By analyzing this vast dataset, the networks learn contextual nuances and improve their ability to accurately interpret legalese over time.
4. Assessing the performance of these networks involves using metrics like BLEU scores. These scores compare the AI-generated plain English output to human-produced translations of the same legal text, providing an objective measure of how well the network performs.
5. While these neural networks are sophisticated, they're not infallible. They can struggle with the subtleties of legal language, occasionally simplifying things to the point that key details are lost. This can create a risk of misinterpretation, hindering a full understanding of the original document.
6. The flexibility of neural networks means they can be specialized for specific sectors. This allows them to adapt their translations to industry-specific terminology and common trends, leading to more accurate and nuanced results within a given context.
7. One of the key benefits is the potential to reduce the time investment analysts spend on interpreting filings. This streamlines processes that often get bogged down by dense, complex language and can potentially free up analysts for more insightful work.
8. The introduction of neural networks for legal document interpretation brings up important questions regarding the legal responsibilities associated with relying on automated systems. We need to carefully consider issues of accountability and ensuring the accuracy of the output for legal or financial decision-making.
9. Organizations using these AI tools frequently experience increased compliance, as the clearer, simplified language facilitates a better understanding of regulations among employees. This, in turn, contributes to better adherence to the rules and guidelines.
10. The integration of neural networks into financial analysis demonstrates the ever-increasing relationship between technology and finance. It challenges traditional analysis methods and suggests a shift towards new, more AI-driven approaches in the way financial information is processed and understood, with potential implications for investment strategies.
How AI Legalese Decoder Transforms EPS Report Analysis A Technical Deep-Dive - Machine Learning Models Used For Financial Risk Assessment Detection
Machine learning models are becoming increasingly important in financial risk assessment, moving beyond traditional methods like statistical analysis and manual reviews. These models allow financial institutions to analyze enormous amounts of data, leading to more accurate predictions of credit risk. Furthermore, machine learning has found a key role in compliance and risk departments, specifically when it comes to recognizing fraud, money laundering, and other financial irregularities. There's been a surge in attention from both academics and financial businesses around integrating AI and machine learning within finance, highlighting their adaptability in addressing different kinds of financial risks. While offering improvements to efficiency in risk management, these tools also offer a deeper look at larger risks within the financial systems. However, the reliance on automated systems raises questions about responsibility and the risk of misinterpreting data, emphasizing the need for careful control when using machine learning in financial analysis.
Machine learning models are becoming increasingly common in financial risk assessment, often replacing traditional statistical methods and manual reviews. These models leverage the ability to sift through massive datasets, helping financial organizations predict credit risk more accurately. There's a clear trend of both industry and researchers focusing on AI and machine learning within finance, particularly when it comes to compliance and detecting things like fraud or money laundering. Some industry experts predict AI and machine learning could bring a substantial boost to the banking sector by improving risk management and decision-making. Research on the topic shows a significant rise in publications related to machine learning and credit risk, highlighting its increasing importance in the field. AI and machine learning are changing how we approach and control financial risk, making various risk management processes more efficient. We're seeing researchers create models using large datasets not only to improve decision-making, but also to tailor services to individual customer needs. The use of machine learning has expanded beyond just credit risk into broader financial risk management, showing its adaptability across different financial settings. It's becoming clear that machine learning is essential for tackling systemic risks present in the financial markets, enabling a more robust analytical approach to financial analysis.
While powerful, there are some nuances to consider. The reliance on historical financial data for training can lead to biases embedded within the models, requiring ongoing evaluation and adjustments. Machine learning, however, can pick up subtle patterns in transactions that might evade human analysts, making it valuable for fraud detection. An intriguing aspect of these models is their ability to adapt to new data and changing market conditions, which can be helpful in volatile times. Assessing how well they're performing relies on metrics like the AUC-ROC, which measure the balance between correctly identifying risks and avoiding false alarms. There is evidence suggesting that certain machine learning models are outperforming traditional methods like logistic regression in predicting risks, potentially suggesting a shift in how risk is analyzed. Techniques like feature engineering allow models to create unique indicators of financial distress, such as leveraging sentiment analysis from news to potentially predict stock performance and risks. Handling the large datasets often involved in finance may require dimensionality reduction methods like PCA to improve performance and interpretability. It's crucial to remember these models are not perfect and their effectiveness can lessen in unfamiliar or unprecedented situations, highlighting the need for ongoing human oversight. There's growing recognition from regulators about the role of machine learning in risk detection, leading to new guidelines for ensuring these automated systems are transparent and accountable. This move towards greater regulation suggests a shift towards a more structured environment in the financial sector.
How AI Legalese Decoder Transforms EPS Report Analysis A Technical Deep-Dive - Natural Language Processing Extracts Key Performance Metrics From 10Q Reports
Natural language processing (NLP) has emerged as a valuable tool for extracting crucial performance metrics from 10Q reports, enhancing the way we understand financial disclosures. This technology lets analysts navigate complex language with greater accuracy, unearthing important details that might otherwise be missed. By automating the process of pulling out key data, NLP models not only reduce the time spent on manual analysis but also minimize errors that could arise from human interpretation. The journey isn't without challenges though. Maintaining the accuracy of the data extracted and handling any potential biases within the models are ongoing areas of focus within the field. The inclusion of NLP in financial reporting marks a shift in how we access and utilize vital data in the world of finance.
Natural language processing (NLP) models have shown promise in automatically extracting key financial metrics, like earnings per share or revenue, straight from 10Q reports. Essentially, these models turn the complex text of these financial reports into structured data, making it easier to analyze.
These models often use techniques like identifying specific entities (named entity recognition) to pinpoint and categorize important numbers within the text. This rapid extraction process helps analysts quickly identify key financial details for informed decisions.
Recent developments in NLP have allowed for the analysis of sentiment in 10Q reports. Essentially, these models can try to gauge whether management's outlook is optimistic or pessimistic. This provides insights into how a company's sentiment might influence its stock price.
Evaluating the performance of these NLP extractions requires both the usual accuracy metrics and more specialized benchmarks relevant to the domain of finance. This is to ensure that the data extracted follows the specific standards and expectations of financial reporting.
Combining advanced NLP methods with machine learning lets these models better understand contextual relationships within the text. This is especially important for accurately interpreting complex financial disclosures where the meaning of a phrase might hinge on the specific words used or the broader context.
While these NLP models are impressive, they still sometimes struggle with ambiguous language. This means there might be a need for human review to ensure that the subtle nuances of specific financial numbers are interpreted correctly. This raises concerns about whether fully automated analyses are reliable enough on their own.
Using these tools can significantly shorten the time it takes to analyze reports traditionally. It’s possible to reduce the analysis cycle from days to a matter of hours. This shift allows financial analysts to focus more on strategic decisions and less on manual data collection.
NLP-driven financial analysis also has the potential to enhance regulatory compliance. These tools can be used to systematically identify and extract compliance-related language in 10Q reports, which could be valuable for internal audit processes within organizations.
Because the language used in financial reporting is constantly changing, NLP systems need ongoing updates and retraining using the most recent filings. This highlights the need for organizations to maintain up-to-date, high-quality datasets for optimal model performance.
Relying on NLP to extract these key performance indicators creates vulnerabilities. These models are sensitive to changes in regulatory language or reporting formats. Therefore, the algorithms need constant adjustments to ensure their effectiveness.
How AI Legalese Decoder Transforms EPS Report Analysis A Technical Deep-Dive - Real Time Data Pipeline Architecture Behind The EPS Analysis System
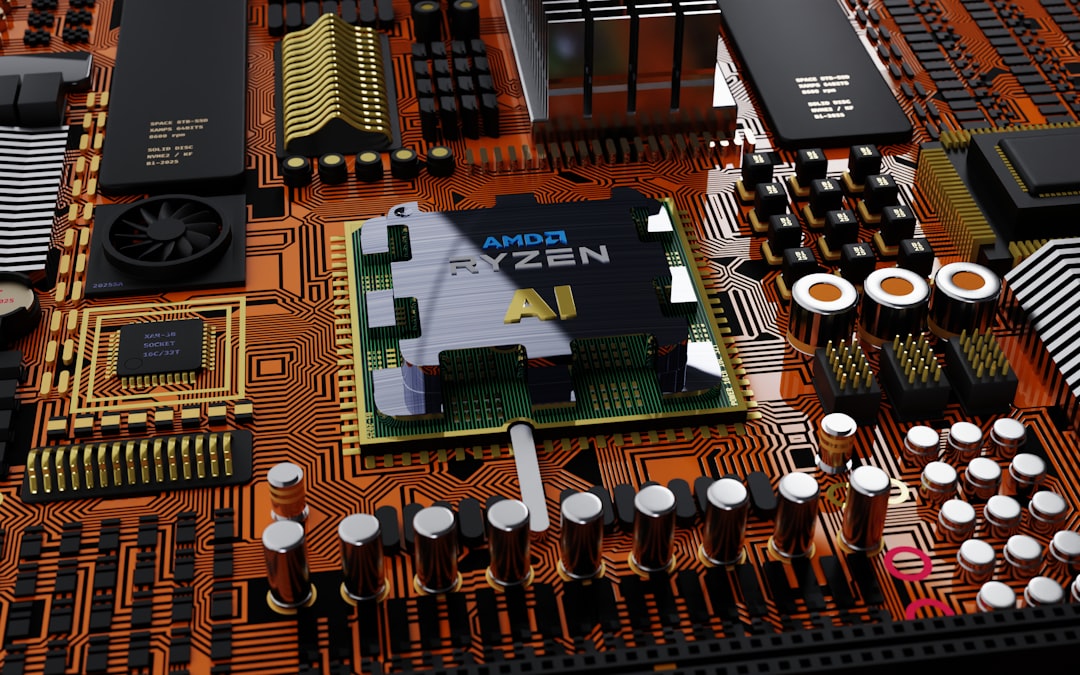
The EPS Analysis System hinges on a real-time data pipeline architecture, which is essential for rapid processing and analysis of earnings per share (EPS) reports. This approach avoids the traditional Extract, Transform, Load (ETL) process, allowing for the immediate ingestion and processing of new data, which is vital for quick responses to market changes and crucial decision-making. The system's foundation is built on technologies like Apache Kafka, for efficiently gathering incoming data streams, and Apache Flink, to quickly process and analyze that information.
The demand for near-instant access to insights is increasing in the financial world, making real-time data pipelines more critical than ever. Building these pipelines isn't simple, demanding careful attention to both data intake and real-time modifications and checks to maintain accuracy. This move towards real-time data analysis highlights the shift within finance towards a more dynamic, adaptable approach to processing financial information, pushing for systems that can scale and respond to the constant influx of data in today's markets. The need for efficient and responsive infrastructure that can manage this data deluge is undeniable.
The EPS Analysis System relies on a real-time data pipeline architecture to quickly process and analyze the flood of EPS reports. This approach is increasingly important in a world where vast amounts of financial data are constantly being generated. Zero ETL (Extract, Transform, Load) is a modern data handling approach that's becoming more prevalent, and it's a significant factor in how this system functions. The pipeline design incorporates the concept of real-time data ingestion, where new data is immediately captured and transmitted as soon as it's available from its source.
A crucial part of building any real-time data analysis pipeline is selecting the right components. In this case, the developers use Apache Kafka for managing data ingestion and Apache Flink to handle the processing and analysis of that data. This real-time approach allows for immediate access to the data, which is vital for AI applications, particularly when training models to get the most accurate and relevant insights. We are talking about real-time analytics here, a process that includes collecting, analyzing, and applying data as it happens, so quick decisions can be made.
The structure of these real-time data pipelines is a critical aspect of creating software that can handle large amounts of information intelligently and with a degree of autonomy. Building this kind of system, however, is a significant engineering challenge. It's not just a matter of ingesting data—you also need to handle data transformation and validation in real-time, which adds to the complexity. The demand for real-time analytics is growing, which means organizations need to build more robust and reliable data pipeline architectures to meet this expanding need.
Data collection is the first step, and it involves pulling data from a variety of sources, such as databases, APIs, and even social media. While the concept of gathering this data may seem simple, it's not without its own unique complexities. The nature of the information and the sheer volume of the data are among the significant challenges to keep in mind. When you have a highly dynamic environment, dealing with the inconsistencies and potential issues in the data coming from multiple sources is a continuous task. We see a growing reliance on AI techniques to handle the challenges of processing and integrating the data into a streamlined format that can then be used for analysis.
How AI Legalese Decoder Transforms EPS Report Analysis A Technical Deep-Dive - Automated Compliance Check Features Track Regulatory Statement Changes
Automated compliance check features are a game-changer in regulatory compliance. These features, often powered by AI, automatically monitor regulatory statements for any changes. This means businesses can stay on top of evolving regulations without the need for constant manual checks. Programs like Lextronai provide real-time tracking of changes, allowing compliance teams to shift their focus from laborious manual tracking to more strategic tasks. This boost in efficiency not only streamlines compliance processes but also helps companies preemptively address compliance issues, ensuring a swift and effective response to regulatory changes. It's important to note, though, that this increased automation brings with it the need for greater transparency and scrutiny. Companies must ensure these AI systems are accurate and reliable to ensure they are accurately reflecting changes in a constantly shifting regulatory landscape, and that there are mechanisms for human oversight when needed.
Automated compliance check features offer a way to continuously track changes in regulatory statements, providing real-time updates. This is crucial for businesses that need to be on top of new compliance requirements and proactively manage risks. It's like having a dedicated watchdog for regulatory changes, reducing the time and effort spent on manually keeping up with legal updates.
Often, these features rely on machine learning models. They can sift through vast collections of legal and regulatory texts to identify changes, minimizing the risk of missing updates. It's a valuable way to ensure continuous compliance by automatically flagging anything new or modified.
It's worth noting that regulatory language is often in a state of flux, making traditional static compliance checks easily out of date. Automated systems are vital for adapting to this dynamic environment. It's like having a flexible response system that allows organizations to adjust their approach to compliance as needed.
The accuracy of automated checks can be further refined by using contextual models. Instead of just spotting a change, they analyze how the modification might impact existing regulations, helping to ensure a consistent and well-aligned compliance strategy. It's a more nuanced approach to compliance beyond simply flagging differences.
Real-time compliance tracking can leverage NLP to extract key changes and alert the appropriate people within an organization. This quick dissemination of relevant information ensures everyone stays in the loop without unnecessary delays or miscommunications. It's essentially a targeted information flow system for regulatory changes.
However, there's a risk that automated systems might misinterpret subtle nuances within regulatory changes. This underlines the importance of human oversight to ensure the accuracy of the interpretations and to validate that they align with legal expectations. A balance between automation and human expertise seems crucial here.
One of the most appealing aspects of automated compliance features is their scalability. For companies operating across borders, it becomes much easier to manage the complexities of multiple jurisdictions without stretching human resources to the breaking point. This is especially important for large multinational businesses.
Automated compliance checks can also be a gateway to using NLP to analyze the general sentiment regarding regulatory changes within the broader market. This can offer a better understanding of how stakeholders may react to new policies and help refine compliance strategies accordingly. It's like having a sentiment gauge for the legal and regulatory world.
While the capabilities of these features are substantial, there's always the risk that they might not keep pace with a continuously shifting regulatory landscape. This highlights the importance of regularly fine-tuning and updating these systems. It's a reminder that these tools, while powerful, aren't a set-it-and-forget-it solution.
Finally, as more organizations rely on these automated features, there's a growing need for clear governance models to ensure that there's a chain of accountability when it comes to decisions regarding compliance that are driven by these technologies. In essence, we need a way to clearly define who is ultimately responsible when these systems make compliance choices.
How AI Legalese Decoder Transforms EPS Report Analysis A Technical Deep-Dive - The Role Of Large Language Models In Financial Document Classification
Large language models (LLMs) are becoming increasingly important for classifying financial documents, especially those dealing with complex regulations. Their strength lies in their ability to handle huge amounts of data and grasp the context within that data, making them well-suited to decipher the intricate language often found in financial documents. These models, particularly advanced ones like GPT-4, have shown promise in interpreting complex financial regulations and extracting key information, including mathematical calculations, from reports and statements. This has led to better insights from earnings per share reports and similar documents.
However, this reliance on LLMs to classify documents comes with its own set of challenges. There's a risk that these models, despite their power, may falter in scenarios they haven't encountered before. This can lead to inaccurate classifications if not carefully monitored. It's crucial that, as financial institutions continue to integrate these models into their operations, they maintain a careful balance between the efficiency these models bring and the need for human oversight to ensure accuracy, especially when dealing with the nuanced language and terminology found in finance. This careful monitoring will be key to preventing misinterpretations that could have serious implications in this highly regulated field.
Large language models (LLMs) have shown a remarkable ability to understand the context and structure of language, which makes them suitable for sorting through and classifying financial documents. We've seen some impressive results, with LLMs achieving high accuracy rates, especially when they're trained using datasets specifically tailored for the financial industry. This helps them grasp the subtle differences in legal and financial terminology.
One of the most practical advantages of using LLMs is the potential for cost savings. They can automate the laborious task of reviewing a large volume of paperwork, potentially replacing much of the work that would traditionally be done manually. This can translate into significant efficiency gains for financial organizations.
Interestingly, LLMs are also able to understand the nuances of language, recognizing synonyms and variations in wording. This is crucial for understanding financial regulations, which often use very specific and complex language that can be difficult for humans to consistently interpret.
We can also tailor LLMs for specific tasks, like detecting fraud or assessing risk. This specialized training often results in better performance compared to general-purpose language models. For companies operating internationally, this feature is valuable since many LLMs are capable of working across multiple languages, which simplifies compliance with varying global regulations.
However, despite the strong performance of LLMs, there can be challenges in interpreting how they arrive at their classifications. This can be problematic when it comes to compliance and needing to justify the decisions of an automated system. This area is something researchers are actively trying to improve.
Additionally, like most AI models, there is a growing concern about potential bias within LLMs. If they are trained on historical data, which often contains inherent biases, then there is the risk that these biases can be reflected in their classifications, which could lead to errors or unfair results.
A positive development is the emergence of transfer learning in the field. LLMs can now be trained with smaller datasets and still maintain high levels of accuracy. This makes it easier for organizations to implement and customize LLM-based systems for document processing.
Furthermore, LLMs can quickly adapt to changes in regulations due to their ability to be retrained with new data. This offers a huge advantage over traditional methods, allowing firms to be more responsive to changes in regulatory environments.
It's also important to consider the impact of LLMs on the workforce. As these models become more sophisticated, they may change the types of skills needed in the financial industry. There will be a need for individuals who can understand and interpret the output from these AI systems and help ensure compliance with legal requirements.
The use of LLMs in classifying financial documents represents a major shift in how we analyze and understand financial information. While there are important considerations regarding bias and interpretability, their potential to increase efficiency and accuracy makes them an important tool in the future of finance.
Experience error-free AI audio transcription that's faster and cheaper than human transcription and includes speaker recognition by default! (Get started for free)
More Posts from transcribethis.io: