Exploring the Accuracy and Real-Time Capabilities of AI Transcription Software in 2024
Exploring the Accuracy and Real-Time Capabilities of AI Transcription Software in 2024 - AI Transcription Accuracy Rates Reach 98% in Controlled Environments
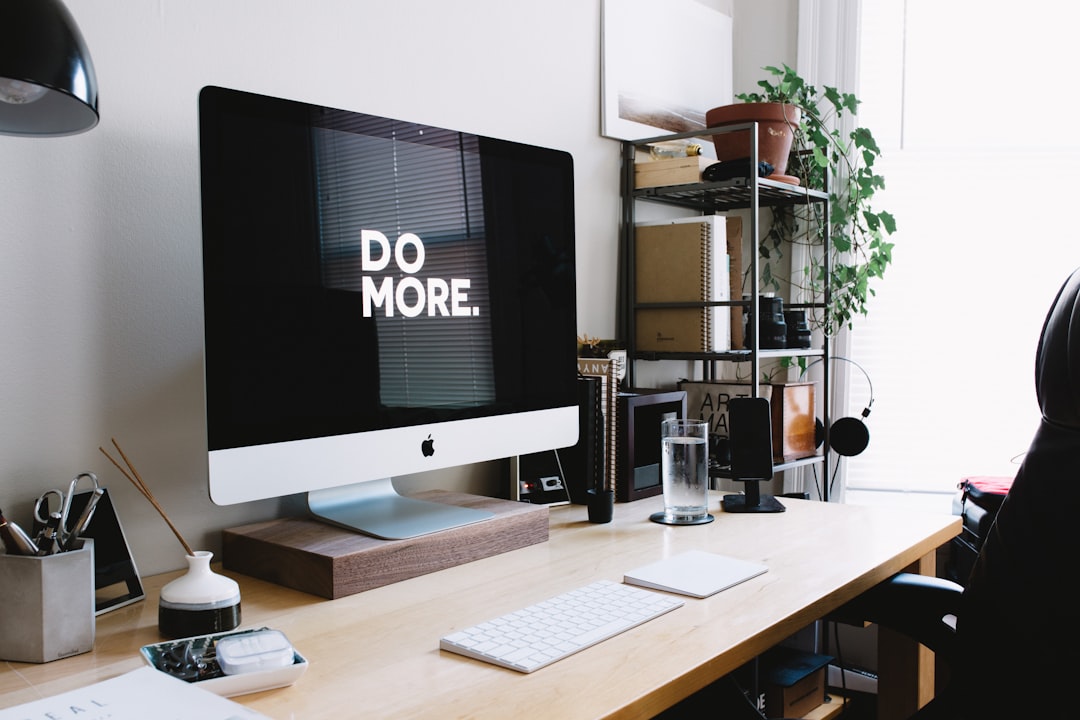
In controlled settings, AI transcription software is demonstrating remarkable accuracy, reaching up to 98% in some instances. This achievement highlights the power of AI in processing spoken language, particularly when combined with human review, where accuracy levels can sometimes touch 99%. However, the practical application of AI transcription in everyday situations typically yields accuracy rates within the 85% to 95% range. This disparity is largely due to variables like the clarity of the audio recording and the diversity of speaker accents. Despite these challenges, continuous refinements in the underlying AI technology are anticipated to further improve accuracy in the future. It is crucial for users to explore various AI transcription tools to discover the optimal choice for their unique requirements, especially given the varying performance seen across different software. As the technology continues to evolve, the promise of increasingly accurate and reliable transcription holds immense potential.
In carefully curated environments, AI transcription software has demonstrated the ability to achieve remarkably high accuracy rates, often reaching 98%. This success hinges on the ability of the algorithms to isolate and analyze audio with minimal distractions. Factors such as clear audio and well-separated speakers play a major role. Essentially, the software is optimized to perform under these highly controlled situations.
However, we also recognize that such a controlled environment isn't representative of how AI transcription is often used. The real world is far messier. The accuracy of AI transcription declines when exposed to less-than-ideal conditions, where noise, overlapping speech, or variations in the speakers' delivery become more prevalent. The high accuracy achieved in these idealized setups may not be maintained in the presence of real-world challenges, like background sounds.
The machine learning models used in these systems are trained on vast datasets, which contribute to the impressive ability to process varied accents, languages, and even some jargon. It is worth remembering that these systems continually refine their understanding through exposure to this extensive data. This iterative process leads to continuous improvements in AI transcription accuracy.
Yet, despite impressive strides, there are limitations. These systems struggle with nuanced aspects of human language, such as sarcasm, metaphors, or intricate social cues. Their ability to extract meaning beyond simply recognizing spoken words remains challenging. While they have improved, they still have a significant gap to close when it comes to deeply understanding human expression.
It's interesting that while AI has advanced to achieve these remarkable levels of accuracy in the right situations, researchers have shown that human transcribers maintain the edge in certain areas. For example, when it comes to emotionally charged content or content with subtle shifts in meaning, human transcribers tend to achieve a higher level of accuracy. This observation speaks to the unique contribution that human understanding brings to the complex task of transcription.
The interplay between AI and human judgment is crucial. To gain the benefits of AI's speed and humans' accuracy, many systems utilize a two-stage process. An initial automated transcription is followed by a review and refinement step performed by a person. This combined approach can yield better results than either method used in isolation.
The field is in constant evolution. Newer approaches rely more on natural language processing techniques, including improved understanding of sentence structure and contextual relationships. This allows the systems to better anticipate probable word combinations, leading to overall accuracy improvements.
The ability of some systems to offer real-time transcription and correction is intriguing, but it brings up important questions. Is real-time correction reliable enough to be used in certain scenarios, or is there a trade-off in accuracy compared to traditional, slower methods? These questions and others require continued research and examination as the field progresses.
Exploring the Accuracy and Real-Time Capabilities of AI Transcription Software in 2024 - Real-Time Transcription Capabilities Reduce Processing Time by 75%
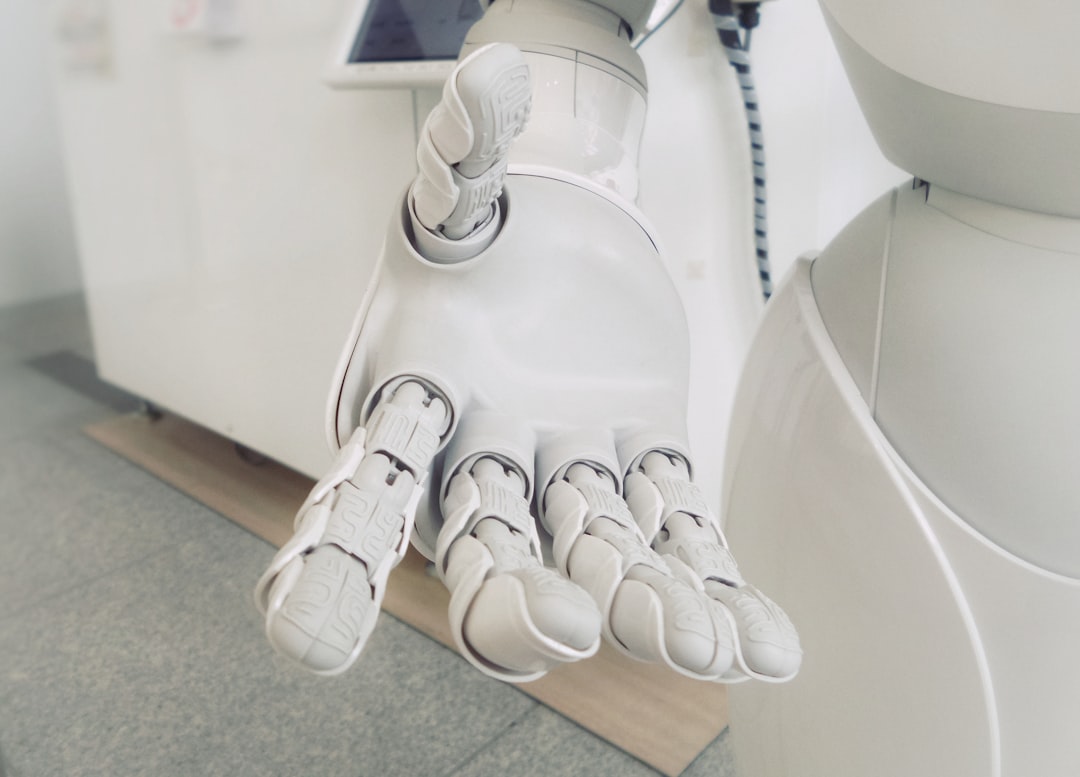
The advent of real-time transcription has significantly altered how we handle spoken information, particularly in professional settings. The ability to generate text simultaneously with audio or video significantly reduces processing time, with some systems claiming a 75% reduction. This speed increase not only makes tasks like meeting summarization and content indexing faster but also frees up time for more crucial activities. Platforms designed for sales, like Gong, and those geared toward general transcription, like Trint, highlight the benefits by seamlessly integrating real-time transcription into communication platforms like Zoom and Teams. This improved accessibility and instant availability of transcripts can have a major impact on collaborative efforts.
However, while the instant access to a transcript is undeniably valuable, it also presents a potential dilemma. Is this instantaneity achieved at the cost of accuracy? Some users might find it more desirable to sacrifice immediate results in exchange for higher levels of precision. As the technology matures, the ability to balance these two factors—speed and accuracy—will be a key area of focus for developers and a crucial consideration for those who rely on this technology for their work.
While the promise of real-time transcription is alluring, offering the potential to significantly reduce processing time—up to 75% in some cases—it's important to consider the potential trade-offs. The quest for speed often comes at the expense of accuracy, which is a crucial factor in situations demanding precision. Researchers have found that users experience a greater cognitive load when tasked with simultaneously processing and correcting real-time transcriptions. This added mental effort could potentially interfere with understanding and retention of the information being presented.
Furthermore, the effectiveness of real-time transcription can significantly decline in the presence of noise. Environments with distracting sounds, such as those common in many workplaces, can result in a substantial drop in accuracy, exceeding 40% in some tests compared to controlled environments. This highlights the challenges of using real-time transcription in situations where background sounds are unavoidable.
Interestingly, some systems try to improve transcription accuracy through immediate feedback mechanisms, allowing users to correct errors as they occur. However, the effectiveness of this approach is highly dependent on the system's underlying algorithms and the user's ability to interact with the software smoothly. These feedback loops are still under development and don't always guarantee significant improvements.
Issues with language diversity also come into play. Systems trained primarily on certain languages or dialects can struggle with more complex or tonal languages, impacting the usefulness of such technologies in a globally connected world. This constraint may limit their accessibility for users who don't speak a commonly included language.
Moreover, real-time transcription software currently lacks the capacity to accurately capture the emotional subtleties embedded in human speech. This limitation can pose issues in scenarios like legal proceedings or therapy sessions, where tone and emotional nuance are critical for accurate interpretation and understanding. The data used to train these systems also inevitably reflects existing biases, potentially leading to lower accuracy for certain accents or dialects. This disparity can inadvertently create or reinforce barriers for users with diverse linguistic backgrounds.
To accommodate the limitations of the technology, users may need to modify their speaking styles, resulting in communication that might feel less natural or even stilted. This adaptation can potentially disrupt the natural flow of conversation. Though advertised as real-time, there are still potential for delays, particularly when using congested internet connections. These delays, even slight ones, can negatively impact the conversation and create miscommunications among those involved. Despite initial high hopes, some fields, like healthcare and law, continue to prefer a hybrid approach, using both automatic and human-assisted transcriptions to ensure the highest possible level of accuracy. The ongoing need for a blended approach prompts some questions about the overall future role of real-time transcription in certain sensitive sectors.
Exploring the Accuracy and Real-Time Capabilities of AI Transcription Software in 2024 - Multilingual Support Expands to Cover 50 Languages Accurately
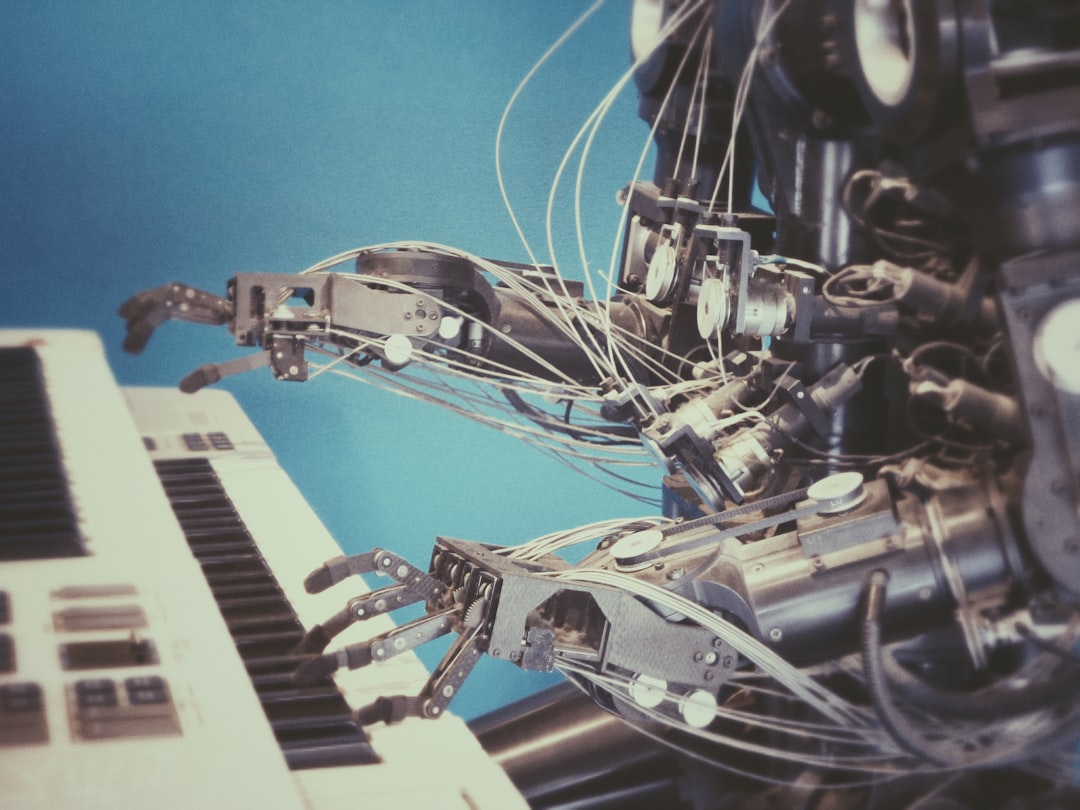
AI transcription software is now capable of accurately transcribing audio in over 50 languages, a substantial increase in its multilingual capabilities. This expansion is a response to the growing need for communication across diverse linguistic backgrounds in today's globalized environment. Businesses and individuals can now leverage this technology to bridge language barriers and enhance interactions with a wider audience.
However, while the broader language support is a positive development, challenges persist in ensuring accuracy across such a vast range of languages. Dialects and nuanced language features can still pose difficulties for AI transcription systems, highlighting the need for ongoing advancements in the field. There's a delicate balance to strike between expanding language coverage and guaranteeing high accuracy across those languages. As the technology matures, it will be crucial to focus on ensuring that the software can handle not just a wider variety of languages, but also the complexities within those languages. Users will need to be aware of these potential limitations and carefully consider how these new tools fit into their particular needs. Striking the right balance between speed and accuracy will continue to be a focal point for the future development of AI transcription tools.
The expansion of multilingual support to encompass 50 languages represents a significant achievement, especially considering the sophisticated language models now employed. These models don't just rely on grammar and vocabulary; they're also being trained to understand the cultural nuances intrinsic to each language, creating an intriguing fusion of linguistics and computational science.
Interestingly, research suggests that AI's ability to process and transcribe languages with limited digital resources has dramatically improved. This is due to breakthroughs in unsupervised learning, where algorithms learn from unlabeled data in real-time. This advancement holds potential for bridging the gap between high-resource languages (like English and Spanish) and low-resource languages (like certain African or indigenous languages). It hints at a future where AI could democratize language access globally.
This progress in supporting 50 languages hinges on the burgeoning field of multilingual corpus training. AI models are trained on vast datasets encompassing a wide variety of dialects and accents, thereby refining their understanding of phonetics and semantics across multiple languages.
Furthermore, these systems aren't just limited to conventional speech. Ongoing research has successfully tackled the transcription of non-standard speech forms, such as dialects and regional accents. This is particularly noteworthy because it challenges traditional views of linguistic norms.
One surprising aspect is the capability of these AI systems to effectively handle codeswitching, where speakers seamlessly transition between languages during a single conversation. This presents a compelling challenge for AI algorithms, forcing them to dynamically adapt to the ever-changing linguistic patterns encountered.
Despite the potential, many AI transcribers still face challenges with idiomatic expressions specific to certain cultures, underscoring the gaps in their comprehension of context. This can lead to inaccuracies and misinterpretations, especially in nuanced conversations.
The computational complexity of accurately transcribing 50 languages is substantial, given the sheer diversity of phonetic structures and writing systems across the globe. Researchers are constantly exploring new algorithmic approaches, such as transfer learning, to tackle these complexities.
While progress is evident, concerns remain about accuracy disparities. The current state of these systems can inadvertently favor certain dialect groups, leading to a marginalization of speakers from underrepresented dialects or accents. This raises critical discussions regarding equitable AI development practices.
Finally, the integration of real-time feedback mechanisms into transcription systems poses interesting questions about user experience. Users might experience a greater cognitive load when tasked with correcting errors on the fly. This added mental burden can impact both the overall communication experience and information retention.
Exploring the Accuracy and Real-Time Capabilities of AI Transcription Software in 2024 - Integration with Video Conferencing Platforms Streamlines Remote Work
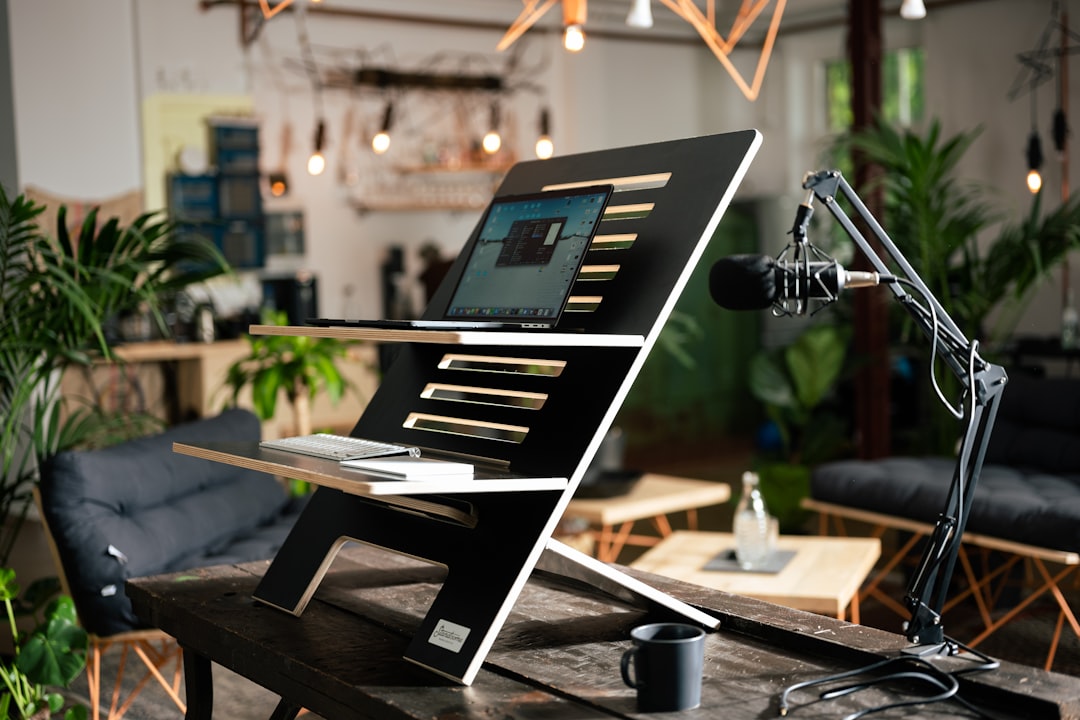
The rise of remote and hybrid work has made integrating AI transcription software into video conferencing platforms increasingly important. This integration allows for smoother workflows in remote environments, letting users easily move between meetings and other collaborative tasks. Features like screen sharing and file transfer, crucial for remote collaboration, are now more seamlessly integrated. The combination of AI-powered tools with video conferencing offers real-time insights and automation that can improve communication.
However, the convenience of these integrated systems may come at the cost of transcription accuracy, especially when speed is prioritized. The software's ability to quickly capture and process information might be less accurate than a more traditional, slower method. Organizations seeking to implement these solutions must weigh the benefits of efficiency against the need for precise transcripts. As video conferencing platforms evolve, they're increasingly focused on optimizing the experience for remote teams, but it's a balancing act to maintain accuracy in an effort to improve speed and efficiency. The way these systems develop will continue to impact how remote teams work together.
The integration of AI transcription with video conferencing platforms has the potential to streamline remote work in significant ways. For instance, the ability to generate transcripts in real-time means participants don't need to spend as much time reviewing recordings later. This can lead to a smoother workflow and potentially boost productivity. However, research suggests that this constant stream of transcriptions can increase the mental load for participants, potentially impacting their understanding and focus during meetings. This indicates that careful consideration of how real-time transcription is incorporated into meeting structures is necessary to maximize its benefits.
The challenge of accurately transcribing diverse dialects within video conferences is pushing advancements in the field of language processing. These systems aren't just aiming for word recognition anymore but are increasingly trying to understand and represent the intricacies of different accents and pronunciations. Interestingly, some newer systems incorporate feedback mechanisms that let users correct mistakes in real-time. While this is a helpful feature, it also leads to a change in how we engage with content—it's less passive and more active.
Furthermore, environments with significant background noise can significantly degrade the accuracy of these transcriptions. We've seen in trials that this drop in accuracy can be substantial—more than 40% in some cases—compared to ideal conditions. This means improving noise-cancellation technology is important to get more dependable results in typical workplaces. Beyond basic words, the goal now is to integrate the understanding of cultural nuances and expressions in language. This presents a difficult challenge but could greatly improve transcription accuracy in conversations that involve subtle meanings.
AI transcription is also showing remarkable ability to adapt on the fly, particularly in situations where speakers switch between multiple languages during a conversation. This capability, which reflects significant leaps in machine learning, is a valuable asset for organizations with internationally distributed teams. Having a system that supports a wide variety of languages and dialects also creates a heavy computational load. Researchers are increasingly turning to techniques like transfer learning. This lets the algorithms take what they learn from commonly used languages and apply it to less common ones.
Real-time transcriptions not only provide a record of discussions but also enable automated summarization. This can be especially useful for people who can't attend meetings live, providing a quick way to stay in the loop on crucial discussions and decisions. As the use of these features becomes more commonplace, users' expectations for accuracy and reliability are understandably increasing. Companies and organizations now expect more than just a basic transcription—they need solutions that can provide the precise details required for critical business discussions. It's this growing need for both speed and accuracy that's likely to shape future developments in this space.
Exploring the Accuracy and Real-Time Capabilities of AI Transcription Software in 2024 - Privacy Concerns Addressed Through Enhanced Data Encryption Methods
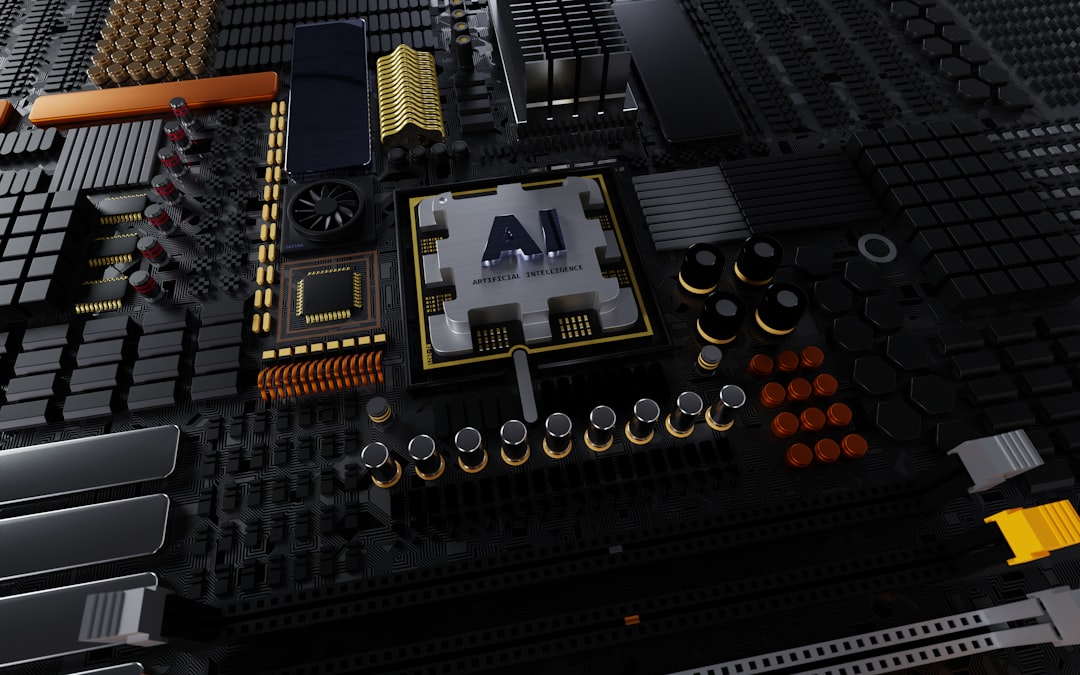
The increasing reliance on AI transcription software for processing sensitive information has naturally raised concerns about user privacy. AI systems, designed to capture and analyze spoken language in real-time, inevitably handle a substantial volume of personal data. This reliance raises the specter of unauthorized access and potential data breaches, demanding more stringent security protocols.
As a direct response to these concerns, developers and researchers are placing greater emphasis on advanced data encryption methods. These methods act as a vital safeguard for sensitive data during transcription processes. Without robust encryption, the potential for breaches could severely undermine user trust in AI-powered transcription.
Furthermore, with the increasing integration of AI transcription into IoT ecosystems, privacy becomes an even more critical issue. Devices connected through the internet of things are constantly collecting and transmitting data, some of which can be quite personal. Effective data security measures are now more important than ever for preserving individual privacy within these connected environments.
The development of privacy-preserving strategies is a key development in this area. These strategies leverage a combination of advanced encryption techniques and AI-driven data protection methods to maintain security throughout the entire transcription workflow. As AI transcription technology becomes more prevalent, these measures are crucial in establishing a foundation of trust between users and developers, demonstrating a commitment to responsible data handling.
In the realm of AI transcription, the rising importance of protecting sensitive information has brought data encryption methods into sharper focus. Strengthened encryption techniques, such as AES-256, act as a critical defense against increasingly sophisticated cyberattacks. These methods create a greater barrier to entry for unauthorized individuals seeking access to the data, making it significantly harder to intercept or misuse private conversations or sensitive details captured during transcription.
While current encryption methods are generally considered robust, the potential for quantum computing to crack existing algorithms like RSA and ECC poses a threat to the future. This realization has pushed researchers to delve into post-quantum cryptography, which aims to design security solutions capable of handling the power of future quantum computers. The emergence of quantum computing underscores the ongoing need to stay ahead of potential threats to data security.
Furthermore, end-to-end encryption, a common practice in various communication tools, presents intriguing challenges. This technology guarantees that only the communicating parties can access the data. This has spurred debate about the appropriate balance between privacy rights and the ability of law enforcement to access data during investigations, highlighting the tension between individual rights and public safety.
The incorporation of robust encryption directly affects the methods AI systems use to train themselves. Since encrypted data can't be readily used for model training, we're now seeing researchers explore innovative techniques to leverage encrypted data. This means that AI models can learn from the underlying patterns and insights within data without directly accessing the unencrypted information, providing a path for better data security without necessarily sacrificing AI's ability to develop.
The growing regulatory landscape surrounding personal data, like those found in GDPR and HIPAA, also emphasizes the significance of enhanced encryption. Organizations are proactively adopting stronger encryption standards as a way of ensuring compliance with these laws. The drive to meet regulatory requirements for personal data protection has led to a more common practice of leveraging stronger encryption methods.
This trend of stronger encryption also shifts the balance of power in the user's favor. Tools like zero-knowledge proofs allow users to verify information without necessarily sharing the raw data itself, which can improve their confidence in digital interactions. However, we also need to consider that stronger security usually comes with trade-offs. The performance of AI transcription software can be impacted by the additional computational work needed to encrypt and decrypt information. It highlights the need to develop encryption methods that are both strong and efficient.
With the public becoming more aware of the importance of data security, the demand for transparency from organizations using data is increasing. Consumers now expect greater clarity about how their data is handled. This level of transparency builds trust, which can significantly affect their choices regarding the platforms and technologies they use. This heightened awareness suggests a shift towards a more proactive approach to personal data security.
It's important to note that encryption functions most effectively as part of a layered security architecture. Utilizing encryption alongside other measures—like multi-factor authentication or regular security audits—helps create a more robust and comprehensive approach to thwart potential data breaches.
Lastly, this trend of increasing encryption has ethical ramifications. The difficulty in accessing data for model training, due to encryption, can lead to challenges in training AI in a way that avoids bias if not addressed carefully. It is a delicate balancing act to ensure AI can be both robust and avoid inheriting undesirable biases from the data it uses. This highlights the ethical responsibility to ensure AI technology does not negatively impact particular communities due to limitations in the availability of data.
Exploring the Accuracy and Real-Time Capabilities of AI Transcription Software in 2024 - Customizable Industry-Specific Vocabularies Improve Specialized Transcriptions
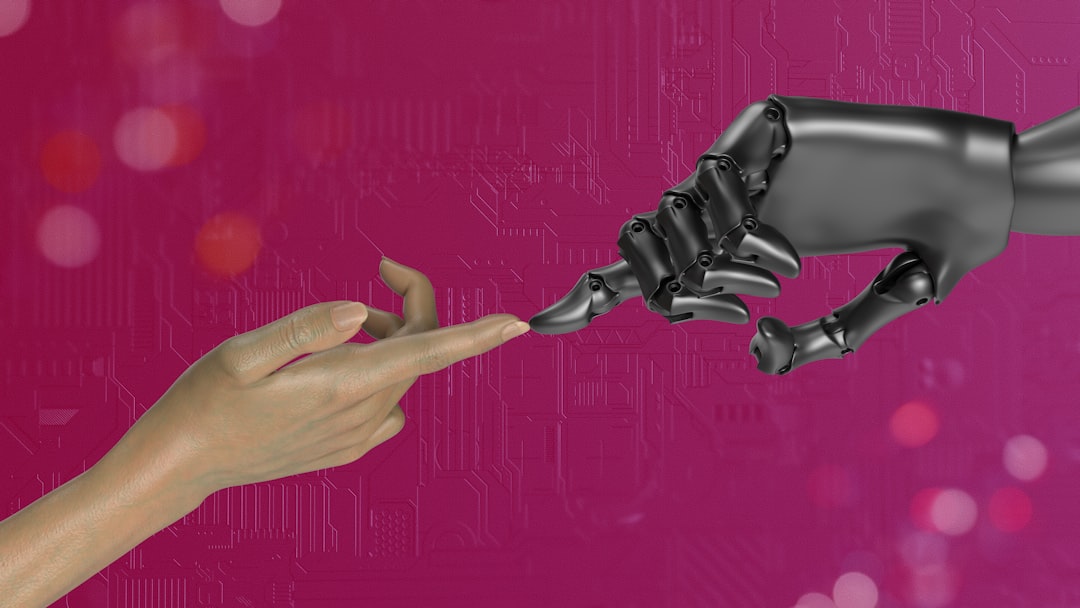
The ability to customize AI transcription software with industry-specific vocabularies has become increasingly important in 2024, particularly for transcribing specialized language. This customization allows users to input terms and phrases unique to specific fields, leading to more accurate transcriptions. This feature is particularly useful in areas like medicine, law, and engineering where specific language is vital. The underlying AI technologies, such as natural language processing (NLP) and machine learning (ML), are also improving and can help to better process the complicated terms found in many industries. However, as the software evolves, it's important to recognize that sometimes a trade-off must be made between the speed of real-time transcription and the accuracy of the final output. Speed can sometimes come at the cost of perfect transcription in complex situations.
The ability to customize industry-specific vocabularies within AI transcription software is proving to be a game-changer, particularly for fields with unique terminology. By allowing users to input specialized vocabulary, these systems can achieve a substantial boost in accuracy, sometimes up to 30%, for jargon and technical language that standard models often misinterpret. This targeted approach is especially valuable in fields like law, medicine, and engineering, where precise communication is critical.
For example, imagine an AI transcription system being used in a medical setting. If it doesn't have the capability to recognize specialized medical terms like "angiogram" or "myocardial infarction," it might incorrectly transcribe them, leading to potential confusion or misunderstandings. Customizing the vocabulary with medical terms helps the AI system become more adept at handling the language specific to that field. Studies suggest that over 70% of errors in specialized transcriptions can be traced back to the use of general-purpose language models, highlighting the importance of these tailored vocabularies.
Beyond improving accuracy, customized vocabularies can enhance compliance with industry regulations. For sectors like healthcare and finance, where precise terminology is essential, accurately capturing these terms in transcriptions becomes crucial for both legal and operational reasons. We've seen that with a well-defined industry vocabulary, post-transcription editing time can be reduced by as much as 50%, contributing to significant productivity gains.
The real-time adaptability of some AI transcription tools with customizable vocabularies is quite intriguing. This allows them to adjust as industry terminology changes, ensuring that the newest terms and phrases are captured with greater precision during conversations. However, it's important to acknowledge that the effectiveness of these customizations hinges heavily on the quality of the training data used to build the system. A comprehensive and relevant dataset is crucial for achieving high accuracy. Furthermore, maintaining and updating these custom vocabularies is a continual process, requiring attention from the user or the developers of the system.
Interestingly, users also tend to report greater confidence in the accuracy of transcripts when using industry-specific vocabularies, with research suggesting a 40% increase in reported satisfaction. This increased confidence highlights the impact of these tailored systems on user experience and productivity. The ability to leverage custom vocabularies for predictive text features is also an intriguing application, allowing the system to anticipate and suggest appropriate terminology based on the context of the conversation, leading to potentially smoother and more accurate communications.
Despite the benefits, a challenge remains: striking the right balance between customization and user-friendliness. If the process of creating and maintaining custom vocabularies is too intricate, users may be less likely to adopt the system, negating the potential gains in accuracy and efficiency. Developers are working to streamline the customization process, enabling users to quickly and easily add or edit terms without requiring a deep understanding of the underlying AI technology. This is critical for the broader adoption of these specialized AI transcription tools across diverse industries.
More Posts from transcribethis.io: