Experience error-free AI audio transcription that's faster and cheaper than human transcription and includes speaker recognition by default! (Get started for free)
Decoding the Soundscape A Deep Dive into Modern Analog-to-Digital Audio Converters in 2024
Decoding the Soundscape A Deep Dive into Modern Analog-to-Digital Audio Converters in 2024 - The Evolution of ADC Technology from 2020 to 2024
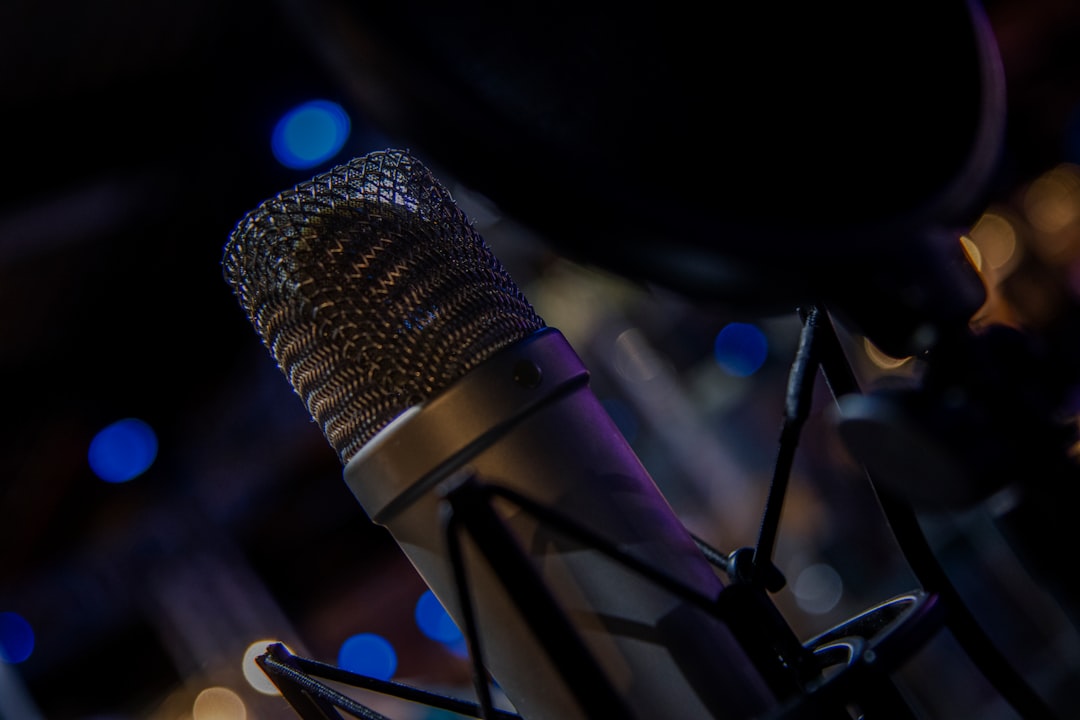
From 2020 to 2024, the evolution of ADC technology has been marked by a push for higher performance while managing power constraints. Traditional ADC design, relying heavily on analog circuits, faced challenges as chip technology shrank. This led to innovations like time-encoding techniques, which sidestep some limitations of traditional approaches by focusing on signal transitions rather than amplitude. Furthermore, the incorporation of deep learning into photonic ADC design has opened up new avenues for improved signal processing.
Looking ahead to 2024 and beyond, the field anticipates the infusion of AI and explorations into quantum ADC principles. These developments promise more precise and efficient conversion processes. There's a clear trend toward minimizing energy consumption in ADC designs, reflecting the growing need for power-efficient solutions. This trend, combined with the continuous refinement of design techniques, helps solidify the ADC's vital role in fields requiring precise data capture and conversion, such as audio engineering, telecommunications, and biomedical applications. As the landscape of digital audio and data processing continues to evolve, the ADC architecture will undoubtedly continue to be refined, reflecting the demand for even higher-fidelity and more efficient systems.
Looking back at the recent past, from 2020 to 2024, the ADC landscape has seen a fascinating evolution. The move to 32-bit floating-point processing has been a game-changer, unlocking a broader dynamic range and offering audio engineers more flexibility, especially in high-fidelity setups. The introduction of advanced noise shaping in sigma-delta ADCs in 2023 has been noteworthy, resulting in a cleaner audio signal even at lower levels.
AI's increasing influence on ADCs has been intriguing. We're now seeing machine learning techniques being integrated into the conversion process itself, enabling the converters to dynamically adapt to the audio environment and optimize for noise reduction and signal enhancement without needing extra hardware. The development of ADCs capable of handling sample rates exceeding 1 MHz in 2021 has expanded the applications for these converters, notably in ultrasound and high-frequency signal analysis, opening up new avenues in data capture and interpretation.
There have also been significant shifts in the underlying materials and design paradigms of ADCs. Gallium nitride (GaN) transistor-based circuits are offering improvements in power efficiency and performance, posing a challenge to the established dominance of silicon in ADC design. The growing popularity of modular ADC designs since 2022 provides designers with increased flexibility and customization options. This trend makes it easier to tailor ADC systems to specific requirements by readily swapping out individual components.
Furthermore, researchers have explored techniques like adaptive differential methods to minimize total harmonic distortion in recorded audio. The increased adoption of high-speed optical data transmission in audio systems is promising, as it can eliminate jitter issues commonly associated with conventional electrical signal connections in ADCs. It's also encouraging to see more emphasis on temperature compensation in newer designs, enhancing the stability and reliability of ADCs across various environments, particularly crucial for portable recording setups.
The convergence of ADCs with software-defined radio (SDR) is another exciting development. This integration grants unprecedented control and adaptability in signal processing, making it possible to tailor the conversion process dynamically based on the audio environment or specific application. This continuous exploration of new architectural approaches and component technologies indicates that ADC development will continue to drive innovation in audio engineering and related fields well into the future.
Decoding the Soundscape A Deep Dive into Modern Analog-to-Digital Audio Converters in 2024 - Understanding the Core Components of Modern Audio Converters
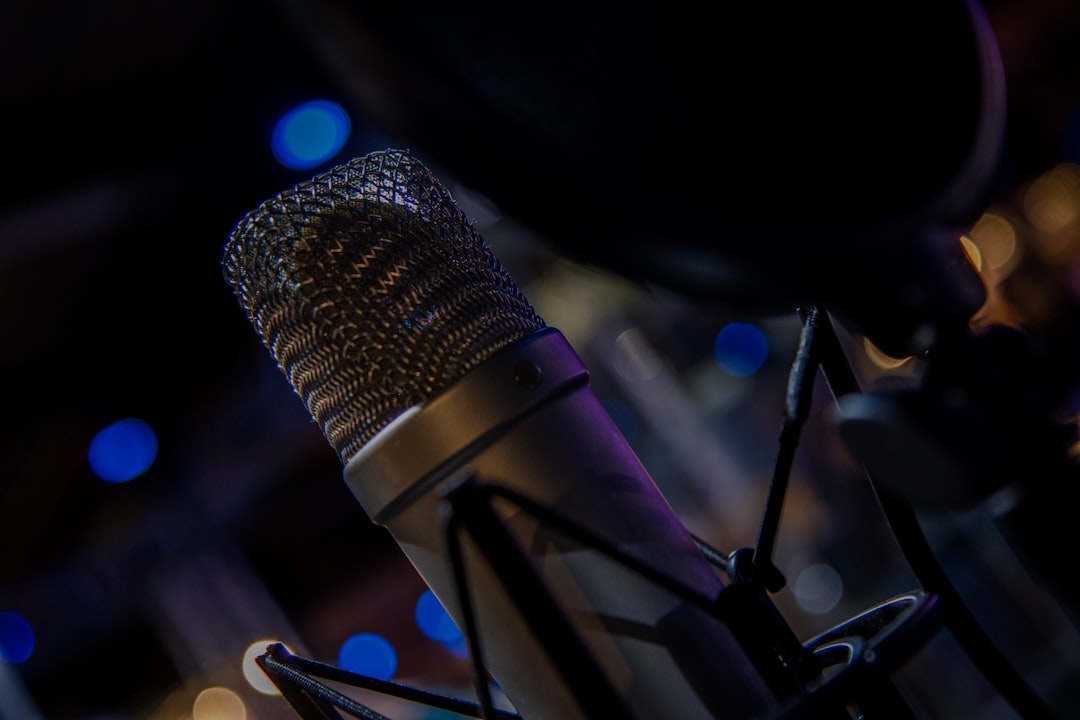
To truly understand the intricacies of modern audio, it's essential to grasp the fundamental components responsible for bridging the gap between the digital and analog worlds. At the core of any audio system, we find the Digital-to-Analog Converter (DAC), a critical component that translates the digital audio signals – the 1s and 0s – into the analog sound waves we perceive through speakers or headphones. Without the DAC, the digital audio we store on computers, streaming services, and other devices would remain inaudible.
Conversely, the Analog-to-Digital Converter (ADC) performs the opposite task, converting analog signals – such as the sound picked up by a microphone – into a digital format suitable for processing and storage. This conversion process is crucial for everything from recording audio to manipulating sound in various software applications.
As these core components continue to evolve, we see increasingly sophisticated techniques being integrated into the conversion processes. Innovations like advanced noise-shaping algorithms in ADCs and the application of machine learning in both DACs and ADCs refine sound quality and offer new opportunities for audio manipulation and enhancement.
The choice of high-quality DACs and ADCs is therefore critical for audio professionals and enthusiasts striving for the best possible sound reproduction. The continuous drive for improved audio fidelity highlights the critical roles these converters play in the evolving world of digital audio. As technology continues its forward march, we can anticipate further refinements and new innovations in ADC and DAC design, shaping the future of how we capture, process, and experience sound.
Digital audio conversion, at its core, relies on the interplay of digital-to-analog converters (DACs) and analog-to-digital converters (ADCs). DACs are the essential components that translate the digital information stored in audio files, like MP3s or those from CDs, into the analog sound waves we perceive through speakers or headphones. Every time we listen to digital audio, a DAC is working behind the scenes. The quality of this conversion, especially the quality of the DAC, is central to achieving a superior listening experience, making DACs a crucial element in modern audio systems like home theaters and stereos.
The process of transforming analog audio into digital data involves complex algorithms that manipulate and refine sound, a field often called digital audio signal processing. This is the role of the ADC, which performs the inverse operation of a DAC. This whole area of digital audio has its origins in technologies like Pulse Code Modulation (PCM), developed in the 1960s, a foundational technique for encoding audio digitally.
A strong understanding of how these converters operate and their various applications helps one appreciate the intricacies of audio reproduction. Audio professionals and enthusiasts are particularly focused on using high-quality DACs and ADCs for optimal sound quality. This focus is tied to the fact that digital audio excels over traditional analog methods in the consistency it provides, ultimately leading to better quality by reducing inconsistencies during recording and playback.
Interestingly, some newer ADC designs are leveraging noise, particularly through advanced delta-sigma modulation, to effectively redistribute it, subtly improving audio quality by shifting it away from the most sensitive frequencies. Another challenge to traditional thinking is that multi-bit quantization, despite earlier assumptions, is increasingly preferred in ADCs over simpler one-bit approaches. This shift toward multi-bit conversion leads to better dynamic range and reduced distortion, which leads to more accurate reproduction of the original audio.
The world of ADC design is also seeing the growing application of optical signal processing, with light signals taking the place of traditional electrical signals. This shift is allowing for higher bandwidth and a significant reduction of a common problem called jitter, opening up possibilities for high-fidelity audio with minimal signal delays. Noise cancellation is also a rapidly developing area in ADC design, which is critical in ensuring the capture of the actual sound we want and the rejection of any unwanted noise.
And the push towards higher fidelity shows no signs of stopping; 64-bit processing in high-end converters is becoming more common, allowing audio engineers to capture sounds and levels of detail that were once inaudible. Researchers are also looking at applying quantum bits (qubits) to audio conversion in new ways, which could revolutionize audio signal processing by achieving levels of detail not available with current methods.
Modern ADCs often incorporate DSP capabilities right into the conversion process. This allows features such as real-time audio effects and enhancements to be added during conversion, eliminating the need for separate processing. And increasingly, machine learning algorithms are being applied to ADC design so that they can adapt and learn to optimize audio based on the specific environment they are in. This is a particularly important application for live sound situations where the acoustic environments can change constantly.
Finally, we are also seeing the integration of MEMS (micro-electromechanical systems) into ADC designs. This combination offers both size advantages and improvements to efficiency, and the technology is easily scaled for use in diverse applications. Additionally, a growing focus on synchronous sampling in ADCs contributes to lower latency and improved synchronization, which is critical for the demanding world of live performance where any delay or timing issues can ruin the experience. The trend of continued evolution in the ADC space is a promising sign for the future of audio reproduction.
Decoding the Soundscape A Deep Dive into Modern Analog-to-Digital Audio Converters in 2024 - High-Resolution Audio and Its Impact on ADC Design
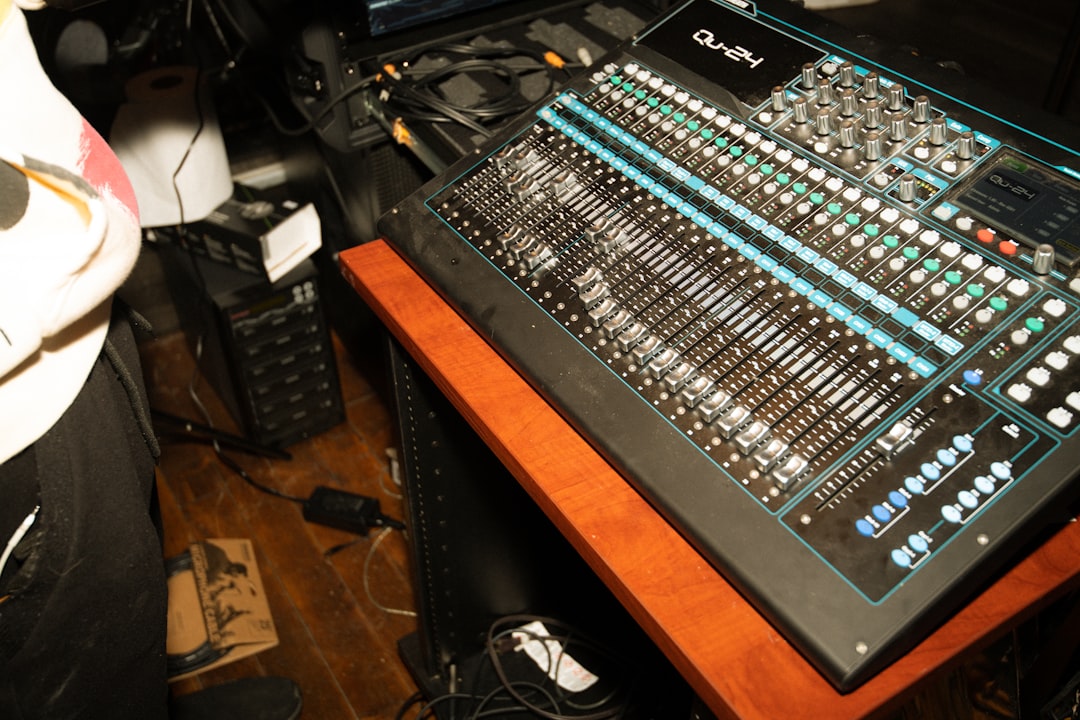
The pursuit of high-resolution audio signifies a drive to capture and reproduce sound with greater accuracy and detail, effectively bridging the gap between the rich, warm qualities of analog recordings and the precision of the digital realm. This pursuit has a direct impact on the design of analog-to-digital converters (ADCs), pushing them to capture a wider range of audio subtleties and nuances.
To achieve high-resolution audio, ADCs are evolving with higher sampling rates and refined quantization techniques, resulting in a more accurate digital representation of the original sound. However, these improvements often come with a trade-off, reducing the bandwidth that the ADC can process. Furthermore, innovative strategies like digital predistortion algorithms and advanced noise shaping are being implemented in ADC designs. These advancements aim to minimize the effects of distortion and improve the overall clarity of the captured audio signal.
As the demand for high-resolution audio experiences continues to rise, ADCs must adapt to meet these increasingly sophisticated requirements. This ongoing evolution ensures that audio engineers and enthusiasts alike can enjoy a more complete and faithful representation of the soundscape, capturing every subtle detail and tonal variation.
High-Resolution Audio (HRA), often characterized by 24-bit depth and 96 kHz or higher sampling rates, aims to capture a wider range of audio information compared to the standard CD quality of 16-bit/44.1 kHz. This increased resolution promises a more detailed and nuanced representation of the sound, potentially leading to a more immersive listening experience. However, achieving this higher fidelity has placed increasing demands on ADC design, particularly when it comes to managing the expanded dynamic range and noise floor. Traditional ADC architectures can struggle with these stricter requirements, prompting researchers to explore new noise reduction techniques and design approaches.
Delta-sigma modulation, a well-established technique, has gained prominence in ADC designs for HRA. It strategically redistributes quantization noise to frequencies outside the audible range, leading to cleaner audio, particularly at lower sound levels. While these improvements can be measured, high-resolution audio also presents interesting challenges related to subjective perception. Studies have shown that listeners often perceive quality differences with HRA, even when objective measurements reveal minimal discrepancies. This highlights that our perception of audio is not solely determined by physical characteristics but also involves complex cognitive processes.
Furthermore, the role of artificial intelligence (AI) in ADC design is becoming increasingly prominent. AI-driven algorithms, specifically machine learning, can dynamically analyze the incoming audio and adapt the conversion process in real time. This ability to optimize performance based on the recording environment or listener preferences offers exciting possibilities for audio enhancement. For instance, the ADC could learn to effectively reduce noise or optimize for a certain sound profile.
Optical techniques have emerged as a promising alternative to traditional electrical signal processing in ADCs. The ability to replace electrical signals with light offers significant advantages in terms of bandwidth and eliminates issues associated with jitter, a common timing error in digital audio that can cause distortions. The benefits of increased bandwidth and reduced jitter could pave the way for even more accurate high-resolution audio capture and processing.
The materials used in ADC fabrication also significantly influence their capabilities. For instance, GaN transistors offer improved linearity and bandwidth compared to silicon, which has been the traditional foundation for many ADCs. This potential shift in the underlying semiconductor technology could lead to even higher fidelity in the conversion process and reshape ADC design philosophies.
While single-bit designs were initially favored, we've seen a growing trend toward multibit quantization in modern ADCs. This approach leads to reduced harmonic distortion and a wider dynamic range, providing the ability to capture and process the intricate details present in high-resolution audio formats.
Synchronous sampling, where the ADC samples the audio at precise intervals, is becoming increasingly important for high-resolution audio, especially in live settings. This technique minimizes latency and ensures precise synchronization, which is crucial for optimal listening experiences where any timing errors can degrade the overall quality.
Finally, the integration of MEMS technology into ADC design opens up exciting possibilities for miniaturization without sacrificing performance. This development is especially beneficial for applications involving portable audio devices, making it feasible to capture and process high-resolution audio in a compact and energy-efficient form factor. These innovations in ADC design showcase the ongoing pursuit of capturing and reproducing ever-more nuanced and detailed audio experiences.
Decoding the Soundscape A Deep Dive into Modern Analog-to-Digital Audio Converters in 2024 - The Role of Machine Learning in Enhancing ADC Performance
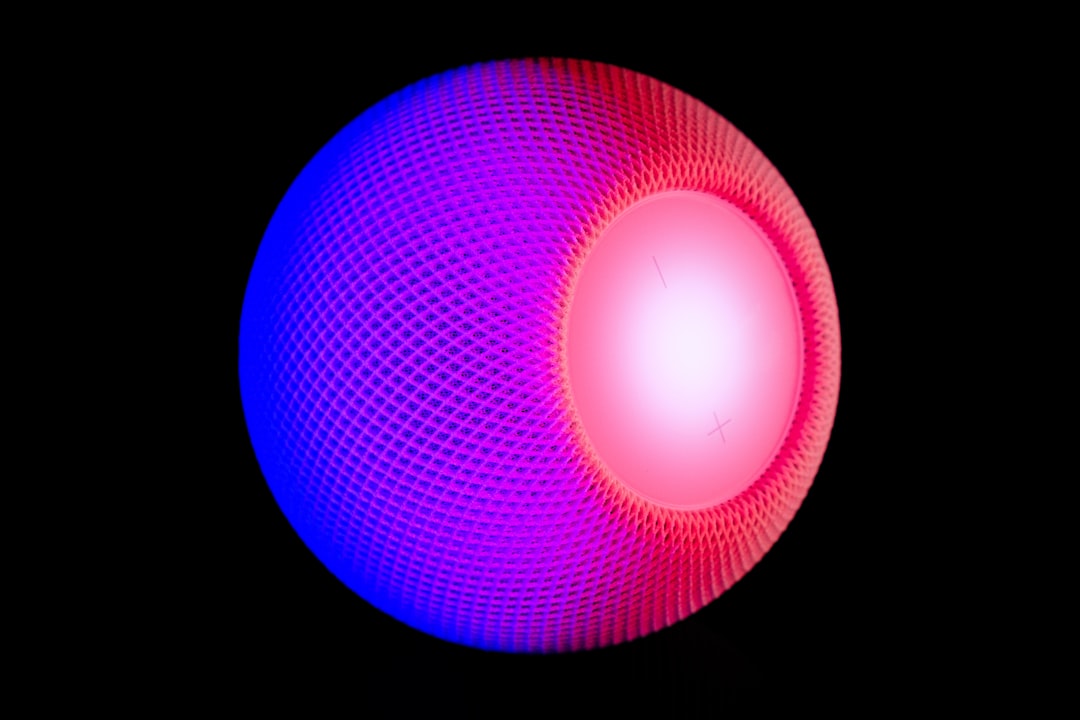
The role of machine learning in enhancing ADC performance is becoming increasingly significant in 2024. We're seeing how machine learning algorithms, particularly within the design of successive approximation register (SAR) ADCs, are being used to address both static and dynamic errors. This approach is noteworthy because it can correct for these errors without needing pre-existing knowledge of the errors themselves. This helps streamline the design process and leads to more straightforward correction techniques. Beyond SAR ADCs, deep learning technologies are also starting to play a more significant role in photonic ADCs. This advancement has allowed for significant improvements in overall signal processing, particularly with regard to the accuracy of the signal.
Additionally, deep learning is helping develop more sophisticated calibration schemes for ADCs that can reduce the need for a lot of on-chip hardware. This leads to a more efficient calibration process, but more importantly it also frees up resources for other parts of the ADC's overall operation. One of the main advantages of using machine learning for ADC calibration is that it can greatly reduce the need for complex and time-consuming matrix operations in the digital domain. These operations are often associated with slow performance, and machine learning provides an efficient workaround for this issue. The overall trend indicates that the demand for better accuracy and efficiency in ADC operations, coupled with the advances in machine learning, will continue to further refine the design and performance of ADCs as a core part of modern audio processing.
Machine learning (ML) is increasingly integrated into ADC design, offering a path to smarter and more adaptive conversion processes. For instance, ML algorithms can now analyze audio signals in real time, allowing ADCs to dynamically adjust their settings. This real-time adaptation leads to improvements in noise reduction and enhances the clarity of the converted audio. We're also seeing ML used for predictive calibration, where historical data helps anticipate performance degradation due to things like environmental changes or component aging. This capability could lead to more proactive maintenance and ultimately, extend the lifespan of ADCs by consistently maintaining a high level of performance.
One area of exploration is using ML to automatically choose the optimal sample rate for different audio signals. This means ADCs can tailor their operation to specific sound characteristics, striking a balance between achieving high resolution and managing efficient processing. Even established techniques like noise shaping are being revisited with ML techniques. Through techniques like reinforcement learning, ADCs can learn to distribute quantization noise in a way that's customized for particular types of audio signals, resulting in cleaner audio reproduction, especially at low volume.
Another impact of ML is on calibration efficiency. Modern ADCs are increasingly designed to self-calibrate with less manual intervention and improved precision due to ML. This reduction in human error helps ensure a consistently accurate conversion. Further, the ability of ML to recognize patterns within sound is a game-changer. An ADC equipped with ML algorithms can potentially enhance specific audio features like music or speech, leading to improved intelligibility or capturing subtle details that might be lost in conventional processing methods.
Compared to traditional signal processing algorithms, ML handles audio signal complexities and non-linearities more gracefully. This can contribute to higher-quality conversions, especially in demanding audio environments like live music performances. There's also a potential for reducing hardware complexity. With ML doing adaptive processing, ADCs might require fewer specialized circuits while still achieving high performance, resulting in both simpler and more efficient designs.
The application of ML allows for smart noise filtering, where filtering techniques can be automatically tailored to the type of audio being converted. This could mean different noise filtering strategies for things like voice versus music, leading to a more nuanced and tailored output signal. Finally, this potential is not limited to audio. The adaptability of ML makes ADCs potentially useful in various fields including telecommunications and biomedical engineering, suggesting that ML-enhanced ADCs might play an increasingly wider role in data capture outside of traditional audio applications. The ongoing exploration and refinement of ML integration within ADC designs point to a future where ADCs are less static devices and more like dynamic, intelligent signal processors, constantly learning and adapting to deliver superior performance across various application domains.
Decoding the Soundscape A Deep Dive into Modern Analog-to-Digital Audio Converters in 2024 - Challenges in Balancing Power Efficiency and Audio Quality
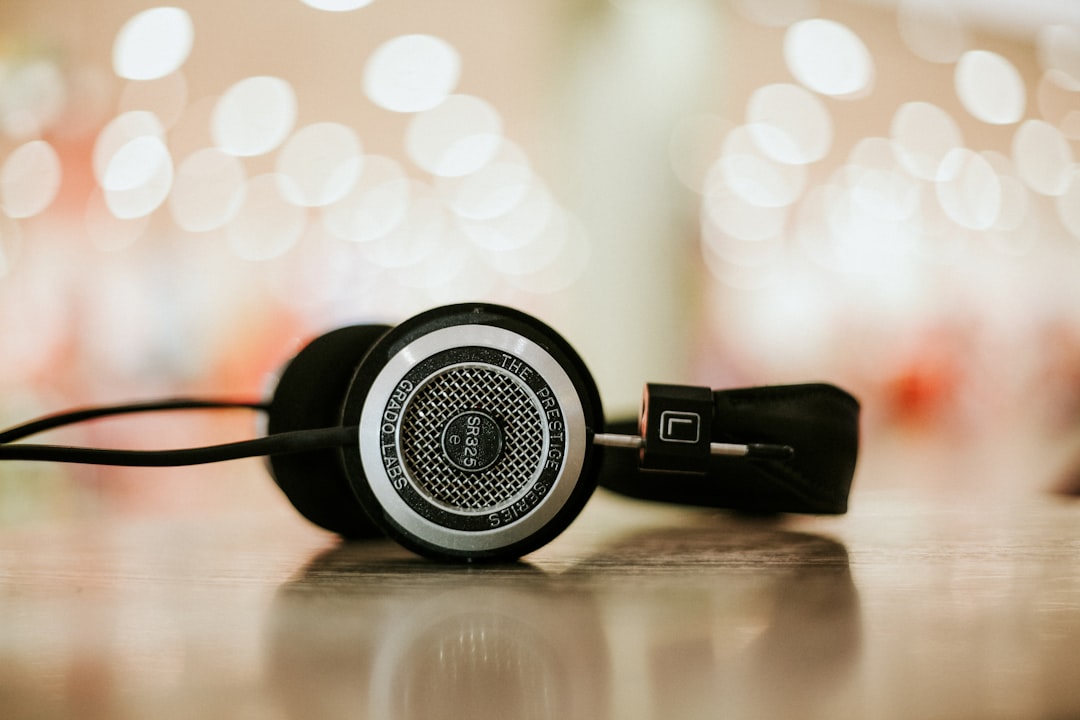
The drive for higher-quality audio in 2024 presents a key challenge: striking a balance between power efficiency and audio fidelity in analog-to-digital converters (ADCs). The increasing popularity of high-performance audio in smaller devices creates a tension between minimizing power consumption and maintaining the intricate details necessary for capturing high-resolution sound. Traditional ADC designs often struggle to meet these competing requirements, prompting innovation in several areas. Advanced noise shaping, for example, has emerged as a method to improve audio quality. Moreover, the integration of machine learning allows for real-time optimization of the conversion process. The move toward multibit quantization and the use of synchronous sampling aims to enhance audio clarity and extend dynamic range, but these features often come with a greater demand on the converter's resources. Consequently, achieving an optimal equilibrium between energy efficiency and top-tier audio quality remains a central hurdle in the continuing evolution of modern ADC technology.
In the realm of modern audio, the pursuit of high-quality sound often clashes with the need for power efficiency, especially in compact devices. Balancing these competing demands presents a significant challenge for engineers designing analog-to-digital converters (ADCs). For instance, lower power levels can lead to an increase in the noise floor, demanding more complex noise management strategies to maintain the intended audio quality.
Designing ADCs involves making trade-offs between low-power architectures and high-performance designs. Opting for low power consumption can often result in some compromise of audio fidelity, while higher-performance designs tend to demand more energy. This balancing act influences the types of algorithms employed in noise shaping and error correction within the ADC.
The selection of sampling rates also contributes to the power consumption dilemma. While higher sampling rates are crucial for capturing more intricate details in audio, they also require more processing power and energy. This can lead to design choices where bandwidth is intentionally limited in specific high-resolution audio applications, highlighting the delicate interplay between resolution and energy efficiency.
Minimizing quantization noise, a fundamental aspect of enhancing audio quality, involves implementing advanced quantization methods. Techniques such as delta-sigma modulation effectively redistribute quantization noise outside the audible range, leading to cleaner audio. However, these refined techniques often translate to higher energy consumption, which can pose challenges for portable devices.
The evolution of hybrid ADC architectures, where traditional techniques are combined with newer technologies like sigma-delta modulation and successive approximation register (SAR) methods, has resulted in notable advancements in audio quality. Nevertheless, this increased complexity can create a challenge in managing power as diverse components may have different power efficiency profiles.
Temperature sensitivity remains a crucial factor affecting ADC performance. Fluctuations in temperature can lead to variations in crucial parameters like gain and offset, prompting the use of complex compensation techniques. These techniques not only add design complexity but can also raise power demands.
The use of adaptive sampling methods allows ADCs to dynamically adjust performance based on signal characteristics. While this adaptability improves audio fidelity and, in certain scenarios, can reduce power consumption, it generally requires more sophisticated computational resources, which can potentially increase overall power usage.
The integration of machine learning to optimize signal processing presents its own set of power consumption trade-offs. Employing machine learning for tasks like noise reduction and error correction can result in higher computational demands and thus increased power usage. Designers must carefully consider this computational load and strike a balance with the desired performance improvements, requiring meticulous power budgeting during the design phase.
The transition from single-bit to multi-bit quantization in ADCs has offered considerable benefits in terms of harmonic distortion reduction. However, this advancement brings about increased complexity in the ADC's power system. Effectively managing efficiency while guaranteeing output fidelity poses a formidable challenge for ADC design.
Future improvements in ADC design and performance might rely on utilizing new materials such as gallium nitride (GaN). This holds the promise of significantly improving both performance and power efficiency. However, transitioning from established materials like silicon comes with substantial engineering hurdles and likely increases in production costs, adding another layer to the optimization process.
The challenge of balancing power efficiency and audio quality is a complex one, requiring a careful interplay of design choices and architectural considerations. The continued pursuit of high-fidelity audio in diverse applications necessitates finding creative solutions to address the trade-offs involved, shaping future ADC designs to achieve an optimal balance of power consumption and audio fidelity.
Decoding the Soundscape A Deep Dive into Modern Analog-to-Digital Audio Converters in 2024 - Future Trends Shaping the ADC Landscape Beyond 2024
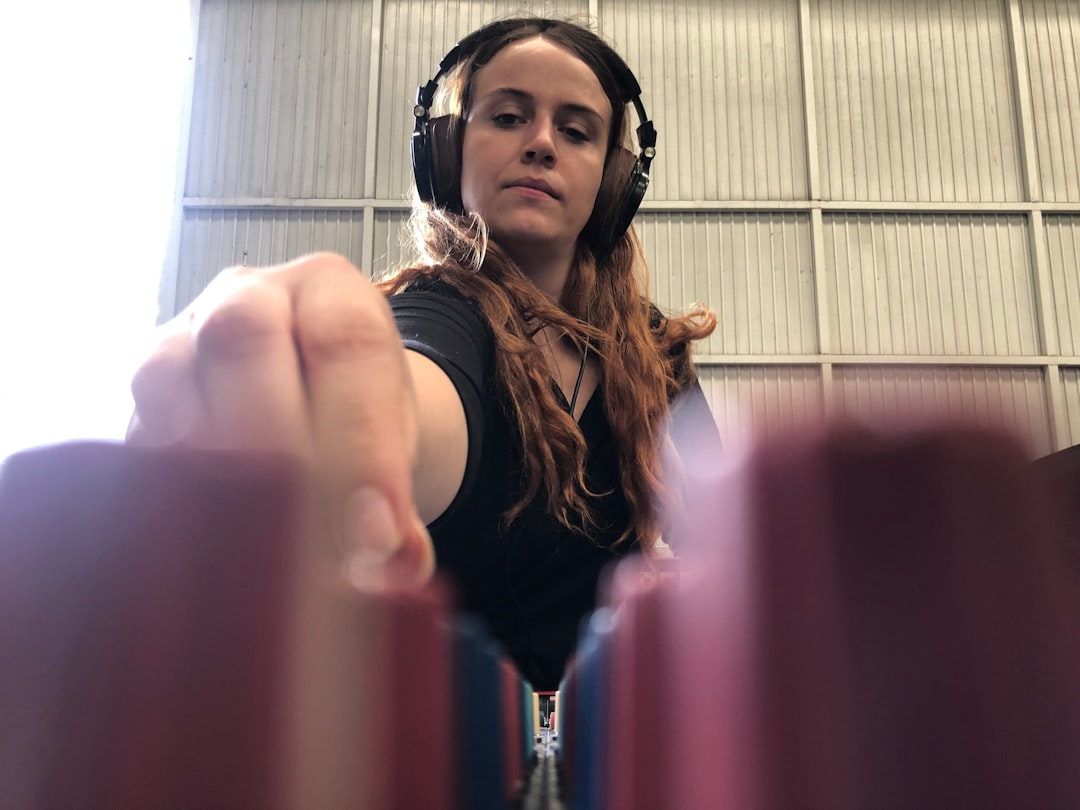
Looking beyond 2024, the field of analog-to-digital conversion is expected to undergo significant shifts, guided by a number of emerging trends. Artificial intelligence and machine learning are increasingly integrated into ADC design, promising more intelligent conversion processes that can adapt to different audio environments and optimize performance. This includes things like noise reduction and signal enhancement without the need for additional hardware. We also see continuing efforts to refine ADC technology to capture audio with greater fidelity, higher sampling rates, and an expanded dynamic range, all while keeping power consumption at bay. These advancements are being pushed by the ever-increasing demand for higher resolution audio recordings and playback in more formats. Meanwhile, there is a strong push for miniaturization, meaning smaller, more efficient ADCs are being designed, which is crucial for portable devices and wearable technology. Further complicating the landscape are evolving industry standards that are impacting the requirements for ADCs in everything from streaming audio to real-time sound processing. These competing demands are leading to novel solutions that address the need for high performance and adaptability while keeping power consumption within reasonable limits. It seems clear that the ADC will need to become more flexible, customizable, and efficient in order to maintain its central role in the processing of audio and other forms of data.
The future of analog-to-digital converters (ADCs) beyond 2024 appears to be intertwined with a number of evolving trends. We might see a shift towards quantum-based ADCs in the coming years. These hypothetical ADCs could use qubits to process audio at speeds and resolutions previously considered impossible, potentially redefining what we consider high-resolution audio. It's plausible that ADCs could become more personalized, using machine learning to adapt the audio output based on an individual's listening habits. This shift would potentially foster a more personalized listening experience.
Material science may also play a role in shaping ADC designs. GaN semiconductors are showing promise in improving ADC efficiency and performance, potentially leading to higher operating frequencies and lower noise floors. It's conceivable that neural network-based ADC designs could emerge, potentially leading to ADCs that bridge the gap between traditional conversion tasks and sophisticated signal processing. This could lead to more advanced noise suppression techniques using AI-driven adaptive filtering, offering even cleaner audio capture.
Future ADCs could become more flexible, perhaps utilizing multi-mode operations that allow them to switch between various sampling rates depending on the needs of the application. Optical signal processing is poised to reduce or eliminate jitter, a significant timing error in electrical signals that affects audio quality. We might see ADCs with dynamic ranges exceeding 140 dB thanks to advanced noise shaping techniques.
Automatic, on-the-fly calibration features could minimize or eliminate manual calibration processes in the future. This would allow ADCs to maintain accuracy and performance in response to changing environmental factors. Improved synchronous sampling methods could help reduce the delay between sound capture and processing in live audio applications, ensuring that the processed sound is aligned with the original captured audio, which is important for sound engineers in live events.
While exciting, these advancements also present a number of open questions. The potential impact on power consumption of new designs or the potential limitations of using AI and machine learning for calibration and audio output are just two factors that must be carefully explored. Further research will help determine which of these trends are indeed feasible and the long-term implications for ADC design and audio quality. It's clear that as the field of audio and digital data processing continues to advance, the need for even more sophisticated and adaptable ADCs will only grow.
Experience error-free AI audio transcription that's faster and cheaper than human transcription and includes speaker recognition by default! (Get started for free)
More Posts from transcribethis.io: