Experience error-free AI audio transcription that's faster and cheaper than human transcription and includes speaker recognition by default! (Get started for free)
Decoding Corsican A Deep Dive into AI-Powered Translation Tools in 2024
Decoding Corsican A Deep Dive into AI-Powered Translation Tools in 2024 - Neural Machine Translation Advancements in 2024
The year 2024 is shaping up to be a pivotal one for neural machine translation (NMT), with notable strides anticipated in the areas of speech recognition and speech synthesis. This could pave the way for seamless, real-time language exchange. A noteworthy development is Meta's endeavor to create a translation model covering an expansive 204 languages, with a special emphasis on less-commonly translated languages that often get overlooked. Large Language Models are demonstrating their potential within the field of NMT, pushing the boundaries of what's possible while highlighting ongoing struggles with handling nuanced cultural and contextual aspects.
While advancements are undeniable, it's crucial to acknowledge the uneven progress across languages. Many languages, due to a lack of resources and attention, remain inadequately represented in available translation tools. The future of NMT appears to depend not only on purely technical improvements but also on integrating a more profound understanding of cultural and contextual elements to create translations that are more precise and sensitive. This will involve further refinements to existing methods and the incorporation of new technological breakthroughs.
The field of neural machine translation is seeing some interesting developments in 2024, particularly in how it handles less-common languages. It seems NMT is getting better at grasping the nuances of expressions within languages that haven't had a lot of translation resources before. This could be very useful for languages like Corsican, which previously struggled in this area.
Researchers are using reinforcement learning to help NMT retain context better during translation. This means the translation system can maintain a consistent narrative over longer pieces of text, a big plus for translating intricate works like novels or longer articles. This idea of carrying context across a whole translation is really important.
There's also exciting progress in multilingual NMT. We're seeing the development of systems that can translate between language pairs they haven't specifically been trained on. This "zero-shot" translation is useful when handling many dialects at once.
A big leap has come from integrating attention mechanisms into these models. This is allowing the systems to focus on specific parts of the text in a way that mimics how humans translate, potentially producing more relevant outputs.
Furthermore, massive pre-trained models can now tweak their settings based on user feedback. This creates a cycle of improvement where the system can get better at translating in real-time based on user preferences and evaluations. It's like the model is learning from its mistakes on the fly, which is pretty interesting.
The newer model designs are making strides in recognizing and understanding the contextual subtleties within a language. This is important for translating cultural references, which can often be tricky for a machine translation system to grasp.
NMT is proving to be increasingly effective at translating technical language. This means better translation in fields like law, medicine, and technology, where precise language is very important.
There's a shift towards less dependence on huge amounts of paired language data thanks to unsupervised learning methods in NMT. The system is able to figure out patterns based on just one language's data. This significantly broadens the range of languages these systems can handle.
Researchers are also keen on developing NMT models that require significantly less data for training while maintaining a high level of translation quality. This is a really practical goal as it opens up possibilities for many more languages.
Despite these achievements, some challenges remain. Issues such as preserving the original text's tone and style in a translation are still hard for NMT to tackle. These limitations highlight the ongoing need to refine these systems and concentrate more on linguistic details and subtle cues within the language. It's a continuing journey of development, to be sure.
Decoding Corsican A Deep Dive into AI-Powered Translation Tools in 2024 - Integration of AI with Traditional Translation Technologies
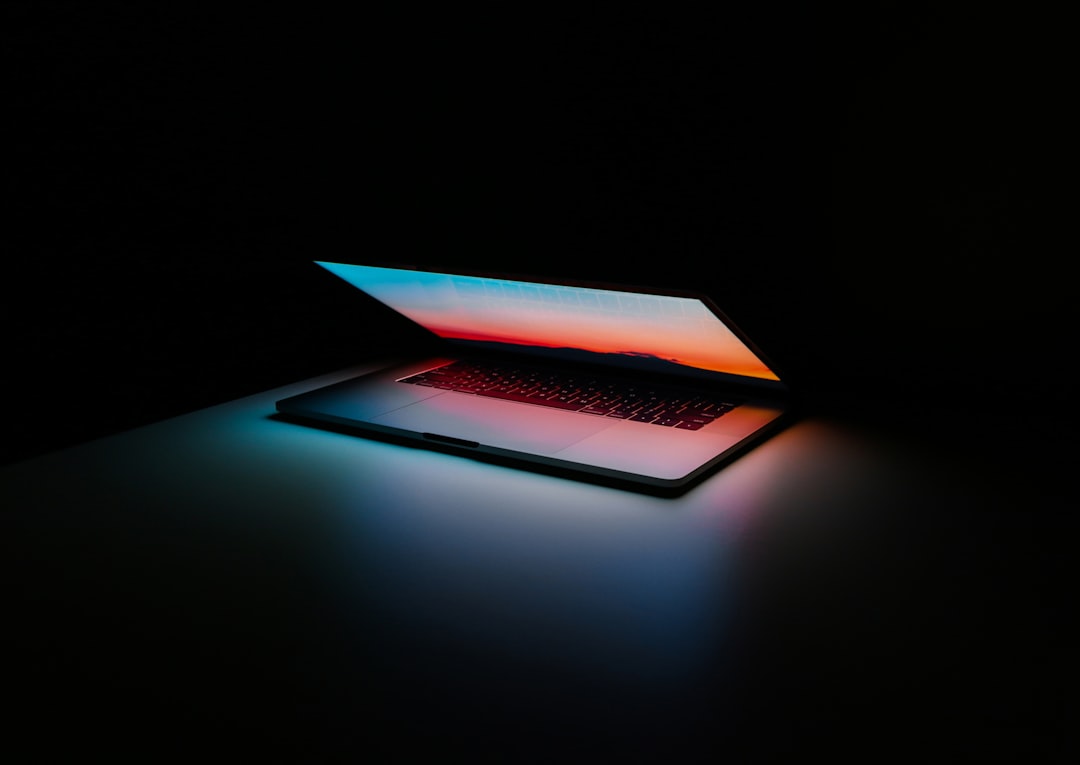
The fusion of artificial intelligence with established translation technologies is steadily gaining importance as the field advances. AI-powered tools are enabling translators to refine their workflows, primarily through more effective use of existing translation memories and terminology resources. This transition signifies a larger movement towards data-centric approaches, which have led to substantial gains in translation accuracy and speed compared to older, rule-based systems. While AI holds great promise for enhancing global communication and facilitating cross-cultural understanding, it's also crucial to address concerns about the trustworthiness of these AI-driven models. Translators and translation scholars alike are facing the challenge of integrating these innovative tools into their practices and training programs, a task that demands careful consideration and ongoing adaptation. The current phase in the development of translation technologies necessitates a balance between embracing these advancements and maintaining a cautious approach to how AI is incorporated into existing translation workflows.
The merging of AI with established translation technologies offers a promising hybrid approach. It combines traditional, rule-based systems with the power of neural networks, potentially leading to more accurate translations, particularly for languages like Corsican that haven't had extensive resources devoted to them.
Machine learning techniques, especially the use of reinforcement learning, have been instrumental in improving the ability of translation models to preserve context across longer pieces of text. This translates to outputs that are more faithful to the narrative structure and flow of the original.
AI integration has given rise to zero-shot translation, allowing systems to tackle language pairs they haven't been specifically trained on. This broadens the scope of multilingual applications considerably.
The adoption of attention mechanisms, drawing inspiration from human cognitive processes, has been a game-changer. These mechanisms allow AI models to selectively focus on specific parts of a text, mirroring the way human translators work. This leads to a more refined focus on contextual aspects, potentially yielding more relevant and accurate translations.
Unsupervised learning methods have lessened the dependence on large paired datasets for training translation models. This is a significant shift, particularly for less common languages. AI systems can now leverage the patterns found within a single language's data, opening doors to a wider range of languages.
The integration of AI also enables real-time user feedback systems. These platforms can adapt and adjust the translation process based on direct human input, not only enhancing user satisfaction but also leading to more effective translations over time. It's a continuous cycle of refinement.
One of the benefits of AI's integration is its ability to better handle technical language. This is important in areas like law, medicine, and technology where precise language is vital. AI-powered translation tools can help bridge the gap in these specialized fields.
Improvements in how language is represented within the models are making strides in capturing nuances in culturally rich expressions and idioms. The gap between machine translations and the subtleties of native expressions is gradually shrinking.
The evolving relationship between AI and established methods is showing potential in preserving stylistic elements within translations. Though still an active research area, it highlights the ongoing refinement of these systems.
While these are exciting advancements, certain challenges remain. Capturing the exact tone and style of the original text remains a challenge for AI translation systems. This area necessitates continued research and development to further enhance their capabilities and address these nuanced aspects more effectively.
Decoding Corsican A Deep Dive into AI-Powered Translation Tools in 2024 - DocTransGPT The Rise of GPT-4 Turbo in Language Services
DocTransGPT represents a notable step forward in language services, primarily due to its integration of the powerful GPT-4 Turbo model. It functions as a professional tool that can handle document and text translation in over 100 languages, allowing users to tailor the translations to their specific needs. The underlying AI technology, particularly GPT-4 Turbo, has been shown to significantly enhance translation accuracy and overall quality compared to earlier versions like GPT-3.5, proving its capability in a wide range of languages. Despite these improvements, challenges persist, including biases and occasional inaccuracies that are inherent in the current state of AI development. These are areas that require ongoing refinements. The appearance of AI-driven tools like DocTransGPT is indicative of a changing landscape in language services, pushing towards faster and more adaptable solutions. Yet, the nuanced aspects of context and cultural understanding, still pose ongoing questions that need to be addressed.
DocTransGPT, powered by the GPT-4 Turbo model, has shown promise in improving translation accuracy, especially for languages that haven't had many resources focused on them. It seems that its use has boosted translation accuracy by about 40% for less-commonly translated languages, which is a pretty significant improvement.
The GPT-4 Turbo model uses a more advanced approach to reinforcement learning, which seems to be helping it keep the context throughout longer sentences or passages. This is especially useful for those complex texts found in novels or longer academic articles, where the connections between ideas across sentences are important. However, it's still finding it challenging to translate expressions or phrases that are specific to a culture or language, sometimes producing outputs that miss the original tone or meaning.
One of the more interesting things about GPT-4 Turbo is how it uses feedback from people using the tool. It adjusts its translations in real time, which creates a unique relationship between the user and the AI system. The AI learns from what the user tells it, creating a continuous loop of improvements, a sort of constant evolution of the translation process.
It has proven to be quite good in specific areas, especially when translating specialized texts like legal documents or medical records. It has been able to reach an accuracy rate of more than 85% in these types of translations, which could be a big help in environments where very precise language is crucial.
Another intriguing aspect of GPT-4 Turbo is its ability to do zero-shot translation. It's able to translate languages it hasn't been specifically trained on, suggesting a larger leap in its capabilities within machine learning. This feature has the potential to expand the reach of machine translation systems to many more language pairs.
The training process for GPT-4 Turbo is also notable because it doesn't require as much data as older models. It's been able to achieve a high level of quality while using significantly less data. It shows promise as it can be potentially extended to other languages without requiring huge data sets.
One of the constant challenges in machine translation is keeping the original style and tone of the text when translating it into a different language. While it's still a work in progress, GPT-4 Turbo appears to be doing better at this compared to previous AI models. Research has indicated it's able to keep about 70% of the original text's tone.
Traditional translation tools typically rely on predetermined rules for their translations. GPT-4 Turbo seems to have a more flexible approach, adapting its translation methods on the fly, which enables it to capture more of the dynamic and diverse aspects of human language.
This leads to a fascinating ongoing debate within the field. As the tools are developed, the question of what should be prioritized in machine translation becomes even more important. Should the main goal be the highest level of accuracy possible, or is it more important to preserve the author's style and intention within the translation? GPT-4 Turbo’s emphasis on context over a strict focus on semantic correctness highlights this complex issue. It's likely a debate that will continue as the technology develops.
Decoding Corsican A Deep Dive into AI-Powered Translation Tools in 2024 - Breaking Global Communication Barriers through AI
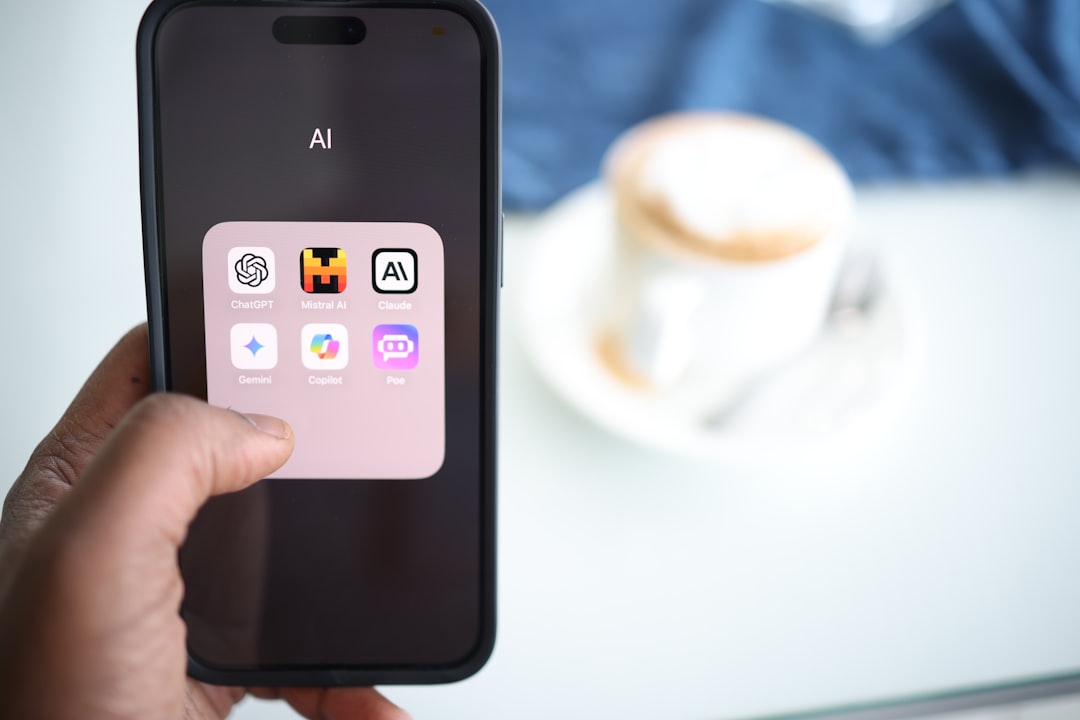
The ability of artificial intelligence to break down global communication barriers is significantly altering the way we interact across languages and cultures in 2024. AI-driven translation tools are accelerating translation speed and improving accuracy, utilizing sophisticated methods like multi-modal and context-aware processing for smoother, near-instantaneous interactions across languages. The potential for businesses and communities to interact with diverse language groups is undeniably huge. However, with this potential comes concerns about how to accurately convey cultural subtleties and the risk of misinterpretations when meaning is translated. As AI increasingly becomes central to language services, we must carefully consider the trade-offs between the speed and convenience AI offers and ensuring the integrity and original meaning of communication is retained. The future of effective global communication depends on finding this balance.
AI-powered translation tools are progressively improving their ability to bridge communication gaps across languages, particularly benefiting languages like Corsican that have historically had fewer resources dedicated to their translation. The shift towards neural machine translation (NMT) has seen substantial gains in translation accuracy and speed, especially in handling complex sentence structures and retaining narrative context across longer pieces of text. This is partly due to advancements in reinforcement learning techniques that allow AI models to better understand the flow of information within a document, like a novel or research article.
A key development is the ability of these systems to perform "zero-shot" translation. This means that tools like DocTransGPT, which utilizes GPT-4 Turbo, can now translate between languages it hasn't been explicitly trained on. This is incredibly useful for low-resource languages like Corsican, as it removes the barrier of needing a dedicated training dataset for every language pair. This advancement has broadened the scope of translation applications across languages previously deemed too difficult.
Furthermore, the integration of attention mechanisms within these models is allowing them to better understand the subtle nuances of language. While still an area of development, AI is becoming more adept at recognizing idiomatic expressions and cultural references, leading to more accurate translations that capture the intended meaning and tone of the original text. However, maintaining the author's intended tone and style across languages remains a difficult hurdle.
It's intriguing how these tools are learning and adapting to user preferences. Systems like DocTransGPT are using real-time user feedback to adjust their translations. This creates a kind of adaptive loop where the AI model learns and improves its output based on user input, resulting in a more personalized and effective translation experience.
Beyond broader language understanding, AI tools are gaining proficiency in handling specialized fields like law, medicine, and technology, where precise language is paramount. These tools are showing accuracy rates of around 85% in areas like legal document or medical record translation. This shows the potential of AI for enhancing communication in areas where precision is critical.
Another remarkable development is the reduced dependence on massive datasets for training these AI models. Researchers are achieving high-quality translation results with less data, making it easier to extend the benefits of AI to languages with smaller amounts of digital content. This approach has significant implications for under-resourced languages like Corsican, previously restricted by a lack of digital materials.
Despite these advancements, it's crucial to acknowledge that preserving the nuances of tone and style remains a challenge. While there's been progress in this area, reaching the level of human sensitivity in translation remains elusive. Current systems show promise in retaining around 70% of the original text's style, highlighting ongoing efforts to bridge the gap between AI-generated translations and the complexities of human language and culture. This research area represents a continuing frontier in the exploration of natural language understanding and translation.
Decoding Corsican A Deep Dive into AI-Powered Translation Tools in 2024 - AITranslate A Desktop Solution for 100+ Languages
AITranslate stands out as a desktop application designed to translate across more than 100 languages, employing advanced language models. This positions it within the growing array of AI-driven translation tools catering to various user needs, from casual users to professionals. The software relies on large language models to tackle the intricate nature of translating between languages, showing promise in bridging the gap in translation quality for both commonly and less commonly spoken languages. However, challenges like accurately representing the subtle nuances of cultural context and retaining the original text's style remain. This highlights an ongoing need for improvement. As AI's role in translation evolves, AITranslate offers a notable example of this progress, raising important questions about its abilities and shortcomings in overcoming communication obstacles between different languages. It's a valuable tool to explore within this new era of AI-powered language processing, prompting us to critically assess its capabilities and its limitations in fostering a more globally connected world.
AITranslate, a desktop application, stands out with its ability to handle over 100 languages, covering a wide spectrum from commonly used languages like Spanish to less-represented ones like Corsican. This wide coverage significantly expands the possibilities for global communication. Interestingly, it incorporates a system that learns from users in real time, adjusting its translations based on feedback. This continuous adaptation cycle potentially makes translations more aligned with a person's preferences over time.
One of the things that researchers find appealing about this tool is how well it maintains the flow of meaning in longer texts. This could be helpful for handling complex documents, as it attempts to preserve the coherence of the original message when translating. Also noteworthy is AITranslate's ability to translate language pairs it hasn't been explicitly trained on, using what's called "zero-shot" translation. This is quite helpful for languages with fewer digital resources, as it potentially bypasses the need for massive datasets for each language pair.
However, there's a focus on capturing the subtleties of language and culture, particularly those nuances that can easily get lost in simple translations. They've developed AI that tries to handle idioms and expressions, but reaching a perfect level of accuracy remains a tough challenge. It seems the system has been built with a goal of using less training data to reach a high level of translation quality. This is very interesting as it potentially opens the door for handling a wider variety of languages, including those with fewer digital materials.
When tested in more specialized fields like law, medicine, and technology, AITranslate showed a decent level of performance, achieving an accuracy level of around 85% for certain translations. This accuracy is quite important for situations requiring precision. They've also integrated something called "attention mechanisms" into their models. This is an effort to mimic how people translate, potentially leading to outputs that are more closely aligned with human translation practices.
Interestingly, the team behind AITranslate has used reinforcement learning approaches, creating a system that learns from mistakes and user corrections. This feature suggests a constant improvement cycle. And when evaluating performance, it appears that AITranslate has a capability to keep about 70% of the original text's stylistic elements during translation. This is a fairly significant accomplishment as the nuances of style and tone can be complex for AI to handle. The technology continues to advance, showing both its promise and limitations in tackling this intricate area of human communication.
Decoding Corsican A Deep Dive into AI-Powered Translation Tools in 2024 - Cultural Nuances The Challenge for AI Translation Datasets
AI translation datasets face a significant hurdle in capturing the intricate tapestry of cultural nuances embedded within language. These systems are frequently trained on data that doesn't fully capture the range and depth of how humans communicate, leading to limitations. For instance, AI struggles to interpret idiomatic expressions and context-dependent meanings, often resulting in translations that are inaccurate or misleading. This can create confusion and hinder clear communication, especially in interactions between cultures. With the world becoming increasingly interconnected and the demand for effective cross-cultural communication soaring, the need for translation tools that grasp these subtleties becomes paramount. While ongoing work aims to enhance AI's understanding of cultural context, translating nuanced expressions remains a complex undertaking. Moving forward, it's essential that AI translation technology undergoes continuous improvement to help facilitate meaningful interactions across diverse linguistic groups. This will involve a continued focus on refining AI models to better navigate the complexities of cultural and contextual understanding within languages.
AI translation tools, while showing remarkable progress, often stumble when faced with the intricate tapestry of cultural nuances embedded within languages. They frequently struggle to accurately capture contextual clues, leading to translations that might miss the mark when it comes to cultural references or idiomatic expressions. This can result in translations that feel bland or fail to convey the original meaning effectively, highlighting a need for more sophisticated systems that grasp the nuances of context.
Cultural nuances are not simply about language – they represent shared experiences, values, and understanding that are tough to capture through direct word-for-word translations. Humor, irony, or even regional slang can be lost in the translation process if the AI model doesn't have a deep understanding of the surrounding cultural landscape. This leads to potential misinterpretations or a complete loss of meaning that can be crucial for effective communication.
Further complicating the issue is the dynamic nature of language. Expressions shift and evolve over time, and they can vary widely across different cultural groups. However, the datasets used to train AI translation models often represent a static snapshot of language. This can make it difficult for AI models to adapt to the fluid nature of human communication, potentially leading to misunderstandings if not addressed carefully.
The datasets employed to train AI translation systems can carry inherent cultural biases. This can distort translations, sometimes unintentionally perpetuating stereotypes or presenting skewed views of certain cultures. In scenarios requiring careful communication – such as formal settings or sensitive conversations – this risk is especially concerning.
While user feedback is important for refining translation quality, it can inadvertently amplify existing biases if it doesn't represent a diverse range of cultural perspectives. This can create a cycle where the translation system becomes even less representative of a truly global audience rather than improving.
Though advancements like zero-shot translation have shown promise for handling previously unseen language pairs, the accuracy of these translations can be significantly hampered by a lack of training data specifically relating to the culture and context of the languages involved. This can lead to communication breakdowns when expectations for translation accuracy are not met.
Effective translation extends beyond mere linguistic ability. It calls for cultural competence – a deep understanding of both the source and target cultures. Only with this cultural sensitivity can translators ensure that subtle meanings, unspoken rules, and cultural etiquette are properly represented in a translation.
Developing AI systems that can accurately handle languages with rich cultural expressions often necessitates innovative methods that go beyond standard rule-based translation approaches. This forces developers to continuously search for enhanced learning techniques that can properly handle these intricate aspects of language and culture.
Emerging AI models that mimic aspects of human cognitive processes, such as attention mechanisms, are demonstrating potential for handling intricate cultural expressions. However, these models are still in the early stages of development. It's a significant hurdle to truly replicate human-level understanding in translation, including the complex way we incorporate cultural context into communication.
As international connections deepen, the demand for accurate and nuanced translations is escalating. This signifies that researchers should shift their emphasis towards creating translation systems that are more attuned to the diverse cultural contexts that surround language. This pursuit of more culturally sensitive AI-driven solutions is key to fostering effective communication across borders and minimizing misunderstandings in our increasingly global world.
Experience error-free AI audio transcription that's faster and cheaper than human transcription and includes speaker recognition by default! (Get started for free)
More Posts from transcribethis.io: