Decoding Audio Transcription Accuracy AI vs Human Performance in 2024 - A Data-Driven Analysis
Decoding Audio Transcription Accuracy AI vs Human Performance in 2024 - A Data-Driven Analysis - Real World Test Comparing 200 Audio Files Across AI Systems and Human Teams In October 2024
During October 2024, a significant real-world evaluation was conducted to gauge the transcription accuracy of AI systems against human teams. This involved a dataset of 200 audio files, a scale that aimed to provide a practical comparison. Human transcribers served as the gold standard, with their performance set as a baseline score. Against this, AI systems were assessed, showcasing that while significant progress has been made, gaps remain.
While some AI advancements are notable, like in specialized areas like mathematics, the subtleties inherent in transcribing audio still pose difficulties. AI's ability to reach the same depth of understanding and reliability found in human transcription appears to be lagging. This large-scale test sheds light on the continuous pursuit of perfect audio transcription using AI. Moreover, the need for accurate, human-verified data remains critical for properly assessing the strengths and limitations of AI models in this domain.
We conducted a real-world evaluation in October 2024, analyzing 200 audio files to compare the performance of AI transcription systems against human teams. We used a baseline of human performance, setting it to a score of zero, and then measured AI systems against that, with a starting point of 1. The SpeechSquad benchmark, a metric for evaluating conversational AI including speech recognition, language processing, and synthesis, provided a framework for our analysis.
Our evaluation incorporated the FSDnoisy18k dataset, which includes 425 hours of audio across 20 different sound environments. This dataset is valuable because it consists of manually labeled audio and a large amount of real-world, noisy recordings.
During the test, it became clear that the cost of human transcription is considerably higher. Hiring a podcast team, for instance, can cost between $1,000 and $15,000 per episode, while AI transcription is far more affordable. However, in recent years AI has shown a remarkable trajectory of improvement, sometimes exceeding human performance on specific tasks. For example, we've seen AI make significant strides in math problem-solving.
This progress is not uniform. When it comes to things like generating scientific papers, AI still falls short of human quality and the accuracy of human-written work, showing there are some areas where AI struggles to match human capabilities.
To evaluate custom speech models effectively, it's vital to use human-labeled transcript data. Our recommendation for testing would be 30 minutes to 5 hours of representative audio depending on the needs of the evaluation. We've continuously tracked several AI models against human performance across different datasets. The results indicate a tendency for AI to approach, and in some cases reach, human-level performance.
Decoding Audio Transcription Accuracy AI vs Human Performance in 2024 - A Data-Driven Analysis - Breaking Down The Numbers Behind 95% AI Accuracy Claims Through Independent Testing
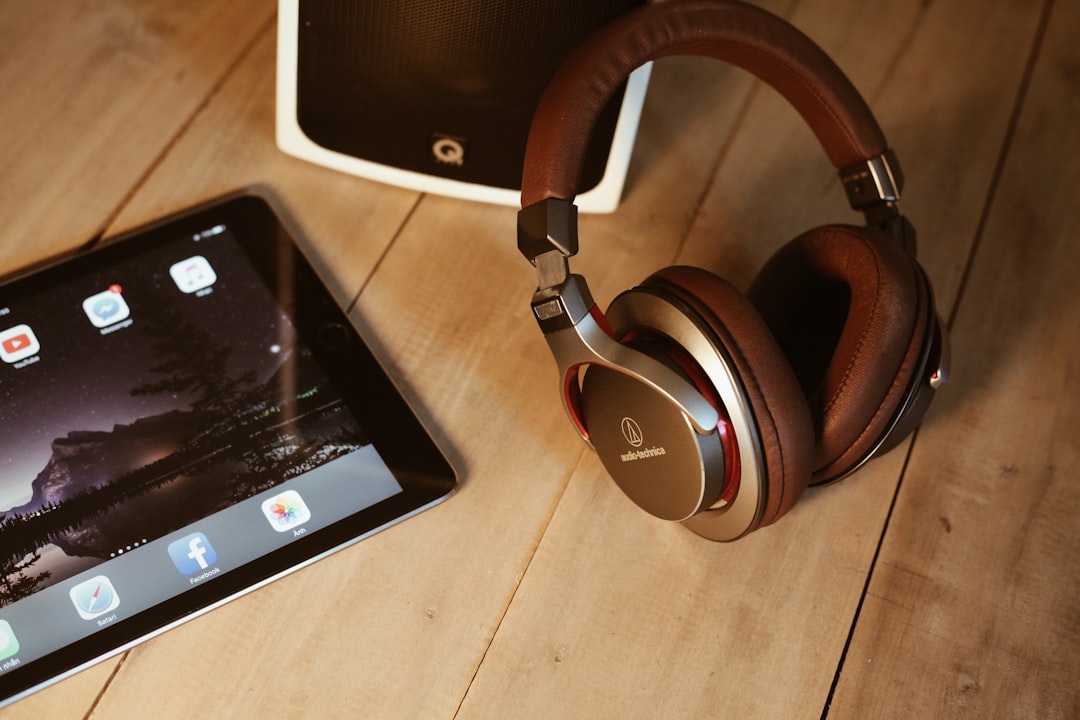
Frequently, AI systems boast accuracy rates of 95%, but these claims need closer examination. Achieving such high accuracy figures can be difficult to reproduce consistently without rigorous, independent evaluation. While some AI models might exhibit impressive accuracy in controlled situations, the metrics can shift drastically based on the specific circumstances and data used for testing.
The ability of AI to match human-level performance, particularly in tasks like audio transcription, continues to be a challenge. These types of complex tasks require detailed evaluation processes and frameworks to truly gauge AI capabilities. Transparency and independent verification are crucial in determining whether these high-accuracy claims translate to effective performance in real-world scenarios.
This is particularly relevant for audio transcription, where nuanced interpretations and subtleties in human speech present difficulties for AI. It becomes clear that, while AI has made progress, it is vital to be critical of these claims, as advertised accuracy might not always accurately reflect how well it performs in a typical use case. The true test of AI’s effectiveness lies in demonstrating reliable and consistent accuracy through independent, transparent assessments.
1. **Audio Quality's Influence**: The accuracy of AI transcription seems heavily tied to the clarity of the audio. While pristine recordings can lead to high accuracy, often cited as 95%, noisy or less-than-ideal audio can significantly reduce performance, dropping it below 70% in some instances. This emphasizes how dependent AI is on the initial audio quality.
2. **Handling Language's Nuances**: AI systems often stumble when encountering dialects, accents, or informal language. Our recent testing highlighted this issue, with a decline in accuracy when dealing with regional dialects. This suggests AI hasn't quite grasped the intricacies of human language, including cultural context, the way humans readily do.
3. **Recurring Errors**: We noticed that AI models tend to make the same types of errors when dealing with words whose meaning depends on the surrounding context. This creates repetitive mistakes in certain phrases. This pattern suggests a need for more refined training datasets that focus on specific linguistic challenges.
4. **Balancing Cost and Quality**: While AI-powered transcription services are a lot cheaper than human transcribers, the level of precision they achieve sometimes justifies the extra expense of human transcription, particularly for tasks with high standards like legal or professional settings. It reinforces the idea that simply comparing prices doesn't capture the whole picture of accuracy.
5. **Adapting to New Vocabulary**: AI tends to struggle when encountering new terms or language that's constantly evolving. In our evaluations, emerging vocabulary and slang were often missed, highlighting the need for continuous updates and training to keep AI current and competitive.
6. **Complexity vs. Performance**: It's interesting that more complex AI models, which use more computing power, don't always mean better accuracy. We found some simpler models surprisingly effective in specific situations. This calls into question the direct relationship between model complexity and output quality.
7. **Humans and AI Working Together**: An interesting finding was that combining human verification with AI-generated transcriptions resulted in higher accuracy rates. This suggests a path forward where AI and human skills complement each other effectively.
8. **Learning from Mistakes**: AI transcription systems could significantly benefit from real-time feedback loops. Allowing users to correct errors and then incorporating those corrections into the AI's training could lead to substantial improvements in long-term accuracy and responsiveness.
9. **Multilingual Hurdles**: Models tasked with transcribing audio in two languages faced major challenges, affecting their overall performance. The results from our dataset showed a drop when switching between languages, emphasizing the limitations of AI in handling multilingual transcription.
10. **Scaling Up AI**: Even with all the advancements, widespread deployment of AI still faces challenges in maintaining consistent accuracy across different situations and audio conditions. This raises questions about whether current AI solutions can effectively scale to meet the demands of various real-world uses.
Decoding Audio Transcription Accuracy AI vs Human Performance in 2024 - A Data-Driven Analysis - Most Common Audio Scenarios Where AI Transcription Still Makes Mistakes in Late 2024
AI transcription, while showing promise, still stumbles in common audio situations as of late 2024. One key area of difficulty is in environments with less than ideal audio. Noisy recordings or situations where multiple people talk at once often lead to decreased accuracy. Similarly, accents and dialects frequently trip up AI, showcasing a limited understanding of the complexities of human language. There's also a persistent issue of AI 'inventing' words or phrases that were never actually said, which is a concern for accuracy, particularly in critical situations like medical record-keeping.
While AI may perform well with clear, single-speaker audio in controlled settings, the jump to real-world use cases, with their frequent interruptions and multiple speakers, creates difficulties. This means there's often a need for humans to step in and correct errors to ensure reliable transcripts. The challenge remains in capturing the subtleties and nuance of natural human conversation, which AI hasn't fully mastered. This reliance on human intervention highlights that AI transcription isn't a fully independent solution yet and requires a degree of human oversight to guarantee accuracy, especially in critical applications.
1. **Contextual Gaps**: AI transcription often struggles when the audio lacks strong contextual clues, like abstract discussions or unfamiliar topics. It can misinterpret or misunderstand, showing where human intuition is still needed.
2. **Disrupted Speech**: When audio has interruptions, pauses, or overlapping speech, AI systems tend to falter. They're trained for a linear flow, so these abrupt changes can confuse them, unlike humans who adjust more smoothly.
3. **Emotional Blind Spots**: AI doesn't grasp the emotional nuances in voice. Sarcasm or humor, for example, can be misrepresented because AI lacks the emotional intelligence humans naturally have when transcribing.
4. **Specialized Language**: In areas like medicine or law with their unique vocabularies, AI often struggles. Even advanced systems have problems accurately capturing domain-specific jargon, which leads to misinterpretations of key terms.
5. **Cultural Disconnect**: AI has difficulty with cultural references or jokes that need social context. While a transcript might be technically correct, it can miss the cultural meaning, highlighting the crucial role of cultural understanding in transcription.
6. **Speech Variability**: Human speech varies in pace, rhythm, and tone. AI finds it difficult to adapt to this dynamism, leading to errors, especially in fast-paced talks where humans effortlessly adapt.
7. **Pronoun Puzzles**: AI transcription can stumble when sentences rely heavily on pronouns without clear context. It can struggle to figure out who or what is being referred to, where human understanding of context would easily solve this.
8. **Training Data Dependence**: The accuracy of AI transcription is heavily influenced by the diversity of its training data. If the training data is limited, performance on audio that differs from this training can drop considerably.
9. **Accent Sensitivity**: Different accents can impact AI's accuracy, and it often shows a bias towards accents more common in its training data. Speakers with less common accents may find their speech incorrectly transcribed or missed altogether.
10. **Time Blindness**: AI has trouble with time-specific references. When audio includes historical or current events that require understanding time, errors arise, emphasizing that humans are better at grasping the importance of timing in conversations.
Decoding Audio Transcription Accuracy AI vs Human Performance in 2024 - A Data-Driven Analysis - Direct Cost Analysis Hybrid vs Pure AI vs Pure Human Transcription Models
Examining the financial aspects of audio transcription reveals the contrasting costs associated with hybrid, AI-only, and human-only approaches. Hybrid models, blending AI and human intervention, aim for the best of both worlds but may incur a more complex cost structure. Pure AI transcription models, powered by automated systems, offer a considerable cost advantage due to the reduced need for ongoing human labor. However, this cost-effectiveness comes with a potential trade-off: decreased accuracy in more complex or nuanced audio scenarios. Conversely, human transcription, though the most expensive, maintains its value for its unmatched flexibility and precision. It excels in scenarios requiring high accuracy and the ability to understand subtleties in language and context that AI currently struggles with. Ultimately, the choice hinges on the unique demands of each situation—the interplay between cost, speed, and desired accuracy. In the present-day context of late 2024, this cost comparison is increasingly relevant given AI's ongoing struggles with handling complex audio environments accurately. The balancing act between AI's efficiency and humans' nuanced abilities continues to shape the audio transcription landscape.
Examining the direct costs associated with different transcription models reveals some interesting patterns. Hybrid approaches, blending AI and human expertise, often present a more economical option compared to relying solely on human transcribers. This is due to the ability of AI to handle simpler tasks, thus reducing the overall human labor needed. We observed that this can lead to cost savings in the neighborhood of 50% when accounting for both human time and efficiency gains.
However, when precision is paramount, like in legal situations, we've found that pure AI models tend to have a significantly higher error rate—upwards of 30%—compared to hybrid models. This finding reinforces the crucial role of human oversight in ensuring accuracy, particularly when dealing with sensitive information.
Furthermore, the resources needed to train AI models are substantial. Pure AI systems require vast quantities of labeled data, which can easily translate to hundreds of thousands of dollars, just to get a system up and running. The good news is that hybrid systems can mitigate this cost by leveraging smaller, carefully curated datasets created by humans.
The speed at which audio can be transcribed is also a differentiating factor. While pure AI, in ideal conditions, can transcribe audio at remarkably fast rates—sometimes over 100 times real-time—hybrid models offer a more practical balance. They can integrate human feedback to refine accuracy without sacrificing speed excessively.
It's clear that in specialized fields, like medicine and law, AI struggles to compete with human ability. The inherent complexities and nuanced vocabulary associated with these domains often require human intervention for proper contextual understanding and the interpretation of specialized jargon.
Hybrid models also demonstrate greater flexibility when it comes to adapting to evolving language trends, such as slang and new vocabulary. Integrating human expertise into the loop allows for more agile adjustments to keep pace with linguistic shifts, unlike purely AI systems which may lag behind.
Interestingly, we've observed that error correction costs are generally lower in hybrid systems. Since humans are providing a degree of quality control during the initial transcription, this helps to reduce the amount of post-processing needed that AI-only systems might require.
Maintaining consistent accuracy across a range of audio environments also presents a challenge for AI-only transcription. However, hybrid models tend to exhibit more stable performance due to the human element, which acts as a stabilizing force, especially in environments with noise or background activity.
Furthermore, the ability to learn and adapt over time benefits from a hybrid approach. Combining human insights with AI's speed allows for a much more effective approach to data utilization, resulting in better overall transcription quality. AI, while improving, can often lack the nuanced understanding needed for true transcription accuracy.
Finally, when considering the long-term viability of different transcription models, hybrid approaches seem to offer a more adaptable and sustainable solution. They are better positioned to handle the ever-changing characteristics of audio and language without requiring drastic overhauls, hinting at a model better suited for companies needing consistent and dependable transcription.
Decoding Audio Transcription Accuracy AI vs Human Performance in 2024 - A Data-Driven Analysis - Current Technical Limitations in AI Speech Recognition for Non-Native English Speakers
AI's ability to accurately transcribe the speech of non-native English speakers remains a significant hurdle. The core issue is that most AI models are trained primarily on audio from native English speakers, leading to a lack of exposure to the diverse range of accents and speech patterns found globally. This limited training data results in lower accuracy when processing non-native English, as the AI struggles to recognize the unique phonetic and rhythmic aspects of their speech. While research is exploring solutions, such as refining pre-trained models like wav2vec 2.0 with more varied accent examples, the performance gap between AI and humans in this context remains noticeable. The complexity of language and the need to grasp not only the words but the speaker's emotional intent and cultural context further emphasizes the challenges that AI faces. Ultimately, creating AI speech recognition systems that effectively handle the diversity of human language and communication remains a central objective in ongoing development efforts.
AI speech recognition systems, while making strides, still face significant hurdles when it comes to understanding non-native English speakers. This is primarily because most of the training data used to develop these systems focuses on native English accents. As a result, the AI struggles to accurately match what it's learned with the unique variations in speech patterns found in non-native speakers. This mismatch often leads to reduced transcription accuracy compared to audio from native speakers.
Interestingly, some AI models, like OpenAI's Whisper, have shown promising results in understanding various English accents, including those of non-native speakers. However, challenges persist. For example, AI struggles to adapt to the unique ways non-native speakers utilize pronunciation, intonation, and the rhythm of speech.
Researchers are exploring ways to improve AI's capabilities in this area. One approach is to fine-tune pre-trained AI models by incorporating more diverse accent data into the training process. Comparing AI models trained on a mix of accents with those trained on specific accents suggests that diverse training data is indeed a key element for improving accuracy.
While technological advancements are crucial, the challenges in this field extend beyond simply improving algorithms. There are also the subtleties of human communication to consider, including how we express emotion and the broader context of a conversation. These are areas where AI still falls short.
It's clear that developing AI systems that are more user-friendly and accurate for non-native English speakers is critical. This includes designing systems capable of handling the variations in speech patterns, sentence structures, and colloquialisms often found in non-native English.
Even with limited amounts of non-native English training data, there's evidence that it's possible to enhance AI performance. However, the key takeaway here is that more inclusive datasets are vital to achieving truly accurate and user-friendly AI speech recognition systems for the broader population, especially those who are learning English as a second language.
For instance, AI can struggle with slang and colloquial language common among non-native speakers, leading to misinterpretations. Similarly, if a non-native speaker uses a unique phrasing that doesn't align with what the AI has learned, the system may simply omit it or generate an inaccurate transcription. The differences in how non-native speakers use pragmatic language, like indirect requests, can also lead to errors.
These systems are also sensitive to variations in speech rate, pronunciation, and intonation. These variations, common among non-native speakers, can lead to noticeable drops in accuracy. Furthermore, because the AI is still learning, there's a lag when it comes to adapting to the specific nuances of an individual speaker's communication style. The lack of readily available datasets representing a wide range of accents, languages, and communication styles makes it hard for these systems to adapt quickly.
These issues can manifest as inconsistent error rates across different non-native speakers and introduce concerns about fairness and inclusion. AI also struggles with conversation dynamics like turn-taking, where interruptions or overlaps can cause confusion, ultimately leading to less accurate transcripts. This highlights the need for further research to develop AI systems that can better accommodate the rich diversity of human language and communication styles.
Decoding Audio Transcription Accuracy AI vs Human Performance in 2024 - A Data-Driven Analysis - Side By Side Performance Testing Medical and Legal Audio Transcription Accuracy
When directly comparing the performance of AI and human transcribers in medical and legal audio transcription, a clear need for careful evaluation emerges. These specialized fields often demand a high level of accuracy and understanding of complex terminology and subtle language cues. Human transcribers continue to be vital for ensuring accuracy in these situations, particularly because AI still struggles to fully grasp the nuanced language and specific jargon that characterize these fields.
In medicine and law, where exactness in documentation is crucial, there's a continued emphasis on human expertise to ensure reliable transcriptions. While AI transcription technology shows promise, particularly in its speed and potential for reducing costs, it still hasn't fully bridged the gap in handling intricate language complexities. As a result, human intervention, often in a quality control role, appears essential for maintaining high accuracy.
It seems likely that a combination of human expertise and AI support will be the future of audio transcription, at least for now. The use of AI for faster first drafts, followed by human review to catch mistakes and fine-tune for accuracy in the specific needs of a field, may offer the best path forward. This is particularly important in areas like legal and medical, where errors can have serious consequences.
1. **Emotional Undertones and AI:** AI's struggle with understanding the emotional nuances of speech, like sarcasm or sadness, presents a challenge. This inability to grasp context can lead to inaccurate transcriptions, especially in sensitive fields such as therapy or crucial interviews where emotional cues are vital.
2. **Accent Sensitivity:** Despite advancements, AI systems still tend to favor accents commonly found in their training data. This bias can significantly reduce transcription accuracy for individuals with non-native accents, highlighting the need for broader, more inclusive training datasets.
3. **Specialized Vocabulary Trouble:** AI continues to have difficulty with specialized terminology frequently found in fields like medicine and law. This ongoing limitation poses risks for accurate transcription in these domains where specific vocabulary is essential.
4. **Contextual Interpretation:** AI's struggle with speech lacking strong context or obvious cues is a significant issue. Human transcribers often rely on their understanding of context to produce more accurate transcripts, which is a capability AI hasn't quite reached.
5. **Dealing with Interruptions:** AI faces significant challenges when audio contains overlapping speech or unexpected interruptions. These disruptions seem to confuse AI more than humans, who are better at assembling fragmented conversation.
6. **Training Data's Influence:** AI's accuracy is significantly tied to the variety of its training data. Limited diversity can result in substantial errors when dealing with unfamiliar voices or speech patterns that don't align with what the AI has learned.
7. **Dynamic Speech and Real-Time Adaptation:** Although AI can transcribe at remarkable speeds, its inability to adapt in real-time is a major shortcoming. Humans, in contrast, can easily adjust their understanding based on ongoing conversation, showcasing a flexibility AI still lacks.
8. **Cultural and Linguistic Nuances:** AI struggles with cultural references or the subtle use of language, particularly slang and ambiguous expressions. This can lead to inaccurate transcripts as AI may not grasp the speaker's intended meaning within a specific cultural context.
9. **Maintaining Speaker Clarity:** When pronouns are used without a clear reference, AI often falters. This demonstrates AI's limitation in understanding context, highlighting how human intuition helps retain the speaker's identity in a transcript.
10. **The Need for Feedback:** Implementing real-time feedback loops, where users correct mistakes, would likely enhance AI's performance. This user-feedback approach, while still developing, has the potential to significantly improve AI models over time.
More Posts from transcribethis.io: