Common Pitfalls in Mandarin Voice-to-Text Transcription Improving Accuracy in 2024
Common Pitfalls in Mandarin Voice-to-Text Transcription Improving Accuracy in 2024 - Tonal Ambiguity in Automated Transcription Systems
Mandarin's tonal nature poses a significant obstacle for automated transcription systems. The four distinct tones, each with its unique pitch and contour, can dramatically alter word meanings. This makes accurate tone recognition crucial for ensuring correct transcriptions. Machines struggle to discern these tonal variations, especially when dealing with natural speech patterns and environmental noise.
Researchers have explored new approaches to address this issue. Combining Haptic Voice Recognition (HVR) and neural network classifiers has shown potential in better distinguishing tones. These systems can potentially improve accuracy, particularly in environments with background noise. The field is actively researching how to incorporate machine learning techniques to further refine tone recognition. The goal is to make voice interactions more seamless and user-friendly. While progress is being made, the challenge of accurately recognizing Mandarin tones continues to be a complex area that warrants ongoing investigation and development.
One of the most prominent hurdles in transcribing Mandarin is the inherent ambiguity caused by its tonal system. A single syllable, depending on its tone, can have multiple distinct meanings, making it a significant challenge for automated systems. Mandarin's tones, characterized by their pitch and shape, are numerous, with at least five distinct variations. This intricate tonal structure places a heavy burden on transcription algorithms, which often struggle to accurately capture these subtle variations.
While humans can achieve an impressive accuracy rate of nearly 95% in recognizing tones during transcription, automated systems lag behind, currently averaging around 70%. This sizable gap in performance poses a significant obstacle to achieving truly accurate transcriptions. Notably, automated systems frequently misinterpret tones within multi-syllable words, which can completely alter the intended meaning. This flaw highlights a critical need for deeper contextual understanding within the systems.
Environmental noise presents a further challenge, capable of significantly distorting tonal patterns, making accurate transcription nearly impossible in noisy environments. The issue of dataset limitations also impacts tone accuracy. When machine learning models are trained on a restricted set of data, they may lack the ability to generalize across different Mandarin dialects and accents, leading to a decline in tonal recognition capabilities.
Sophisticated techniques like deep learning and neural networks have been deployed to improve tone recognition, but even these advanced approaches still face difficulties. They struggle with the subtle fluctuations in tones that can vary between individual speakers. Furthermore, some transcription systems encounter difficulties with homophones—words that sound alike but have different meanings based on their tones. This illustrates a critical limitation in relying solely on surface-level features for language processing.
Beyond impacting transcription accuracy, tonal ambiguity can affect user trust in automated systems. Many individuals prefer manual transcription due to the consistent inaccuracies in tone recognition. Emerging solutions aim to address these challenges by employing real-time feedback mechanisms. These systems leverage user corrections to improve the performance of the underlying machine learning models, potentially leading to significant improvements in tonal recognition over time.
Common Pitfalls in Mandarin Voice-to-Text Transcription Improving Accuracy in 2024 - Handling Regional Accents and Dialects
Mandarin voice-to-text transcription faces a significant hurdle when dealing with regional accents and dialects, primarily due to variations in tone production. Accents can dramatically alter the way tones are expressed, creating a challenge for automated systems designed to interpret Standard Mandarin. For example, speakers from areas like Shanghai and Guangzhou might produce the dipping tone (T3) in a manner quite different from those who speak Beijing Mandarin. This variation can lead to errors in transcription.
Furthermore, the wide range of Chinese dialects, influenced by geography and population shifts, adds another layer of complexity to the transcription process. These dialects often have unique pronunciation patterns and tonal structures that diverge significantly from Standard Mandarin. This linguistic diversity presents a substantial hurdle for current speech recognition technology. To accurately transcribe speech containing regional accents, a deeper understanding of these variations is essential. It highlights a need for both specialized approaches and continuous research to improve the accuracy and reliability of voice-to-text transcription in diverse Mandarin-speaking populations. Ultimately, achieving high-quality transcriptions across diverse accents requires tailored solutions and an ongoing commitment to overcoming these linguistic challenges.
1. **Regional Accent Diversity:** Mandarin, while a unified language, is spoken with a variety of regional accents, including those in Beijing, Shanghai, and Cantonese-speaking areas. These accents often introduce unique tonal variations and pronunciation differences, which can be difficult for automated transcription systems to decipher accurately.
2. **Tone Sandhi Challenges:** Some dialects exhibit tone sandhi, where tones merge or shift in connected speech. This phenomenon can lead to confusion for automatic systems, as they might struggle to identify the intended tone and, consequently, the correct word.
3. **Intonation's Role**: Beyond tones, regional accents possess distinct intonation patterns that also contribute to meaning. Transcription systems trained mainly on Standard Mandarin may misinterpret these subtle shifts in intonation, hindering their ability to grasp the intended message.
4. **Pitch Contour Divergence**: Different regions exhibit unique pitch contour variations within Mandarin. For instance, speakers in southern China may employ a distinct pitch range or melody, which may not align with the models that are often based on northern Mandarin. This mismatch can lead to inaccuracies in the transcription.
5. **Speaker Variations and Social Context:** How individuals speak Mandarin is also shaped by age and social background. Older speakers may have accents that differ from younger ones who may incorporate slang or trends in pronunciation. These shifts in language use can pose difficulties for transcription systems trained on older data.
6. **Homophone Ambiguity in Accents:** The issue of homophones—words that sound the same but have different meanings—becomes even more challenging with regional accents. Automatic systems already face a hurdle in distinguishing homophones based on tone; regional accents can further blur these distinctions, increasing the risk of errors.
7. **Training Data Limitations**: Many machine learning models for Mandarin transcription rely on data predominantly focused on Standard Mandarin pronunciations, neglecting a large amount of regional dialect data. This lack of inclusivity in the training process results in poor transcription accuracy in areas with strong dialectal influence.
8. **Phonetic Overlap Issues**: Some regional dialects share phonetic similarities that can confuse transcription systems. For example, certain sounds like "q" and "ch" might be pronounced in a similar way in specific dialects, making it difficult for systems to differentiate them accurately.
9. **Potential for AI Bias**: Transcription technology can be biased towards the demographic makeup of its training data. If a system is primarily trained on data from a certain region, it might struggle with accents from other parts of the country, highlighting the need for a more balanced approach.
10. **User Adaptation Influence**: It's interesting to note that when aware of being transcribed, users often modify their speech unconsciously, adjusting their tone or accent. While this adaptation can improve accuracy in some cases, it also complicates the goal of developing a universal transcription system capable of understanding a diverse range of speech.
Common Pitfalls in Mandarin Voice-to-Text Transcription Improving Accuracy in 2024 - Contextual Misinterpretation of Homophones
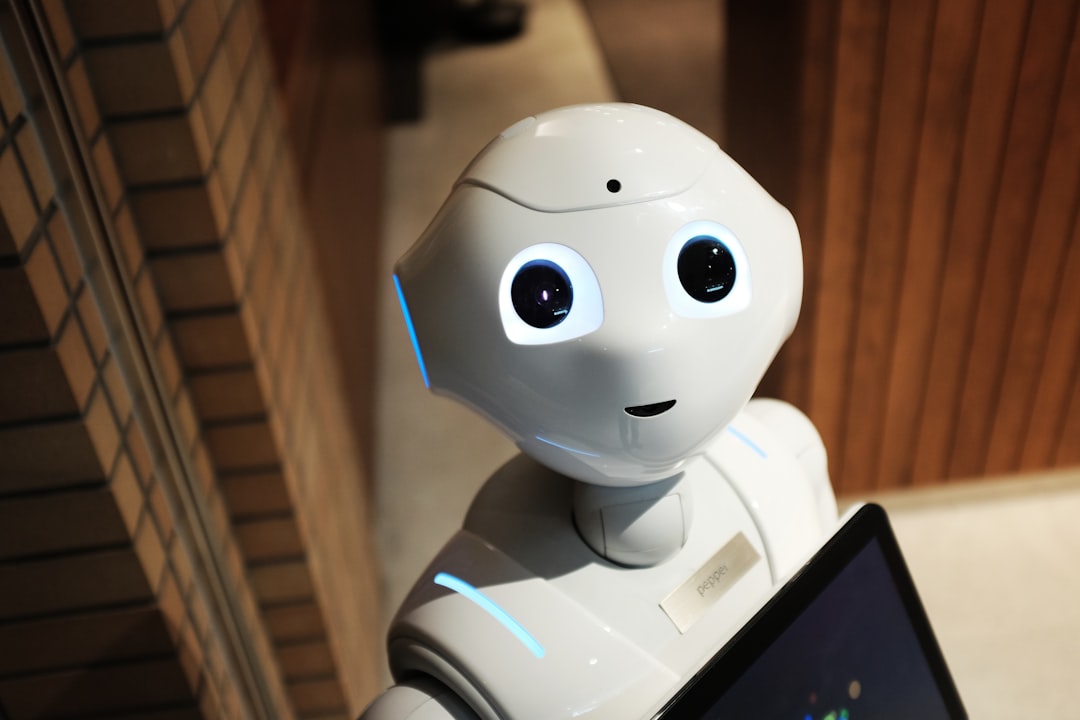
Within the realm of Mandarin voice-to-text transcription, a persistent hurdle lies in the misinterpretation of homophones due to a lack of context. Mandarin, with its abundance of homophones – words that sound alike but carry distinct meanings – necessitates a strong reliance on contextual cues for accurate transcription. Research highlights the crucial role of context in homophone recognition, showing that transcribing words within a complete sentence generally leads to better accuracy compared to isolated words. This implies that comprehending the surrounding words and phrases is pivotal for minimizing transcription errors arising from homophones. Moreover, recent developments in language models, coupled with the integration of semantic analysis, are showing potential in improving the accuracy of transcriptions. These improvements allow systems to leverage contextual clues to deduce intended meanings more effectively. As scientists continue to delve into these intricate linguistic patterns, the prospect of constructing more reliable Mandarin voice-to-text systems becomes increasingly promising.
1. **Contextual Dependence of Homophone Interpretation:** Homophones in Mandarin, words that sound alike but have different meanings, are heavily reliant on context for accurate interpretation. This is especially problematic for automated transcription, as a single phonetic sequence could represent multiple characters with distinct meanings, like "行" (to walk) and "行" (to be acceptable).
2. **Sentence Structure's Impact on Meaning:** Mandarin's flexible sentence structure plays a significant role in resolving homophone ambiguity. The placement of a homophone within a sentence can drastically alter its meaning. While humans seamlessly utilize contextual clues, machines often struggle without sophisticated algorithms designed to capture these nuanced relationships.
3. **Historical and Cultural Context:** The interpretation of certain homophones can be deeply rooted in historical or cultural references. This adds another layer of complexity, as machines lack the extensive background knowledge needed to fully grasp such subtle meanings, leading to frequent misinterpretations.
4. **Challenges of Homophone Clusters:** Mandarin contains groups of homophones that differ only by a single tone, yet their meanings can be drastically different and span diverse semantic domains. This presents a challenge for transcription systems, which might not be able to adequately distinguish these subtle tonal variations.
5. **Impact on Communication and Trust:** Misinterpretations caused by homophones can significantly distort the intended message, potentially undermining user trust in automated transcription systems and disseminating erroneous information. This issue highlights the importance of accurate transcription in maintaining communication integrity.
6. **Cognitive Load on Users:** Users often subconsciously adapt their speech to avoid homophone-related misinterpretations, creating a cognitive load that can impede natural speech delivery. Ironically, these conscious modifications can make it more difficult for some voice-to-text systems to perform accurate transcriptions.
7. **Reliance on Non-Verbal Cues:** Humans frequently rely on non-verbal cues and the broader contextual landscape to resolve homophone ambiguity. Automated systems, however, struggle to interpret these cues, making them more susceptible to errors, especially when dealing with homophones.
8. **Unpredictability of Errors:** Homophone-related mistakes in automated transcription systems can be surprisingly inconsistent. Small changes in tone or phrasing can sometimes lead to large errors in meaning. This unpredictability poses a challenge for ensuring the overall reliability of voice-to-text systems.
9. **Human Auditory Processing Advantages:** Research suggests that humans can sometimes begin interpreting the meaning of homophones before the full sentence context is available. This illustrates that human auditory processing is currently more advanced than our current transcription technology in handling these complex linguistic challenges.
10. **Limitations of Feedback Mechanisms:** While user feedback is being incorporated into transcription systems to improve accuracy, its effectiveness for homophone-related errors is often limited. This lack of efficiency in real-time adjustments to handle homophones hinders the overall improvement capabilities of many transcription systems.
Common Pitfalls in Mandarin Voice-to-Text Transcription Improving Accuracy in 2024 - Background Noise Interference in Audio Quality
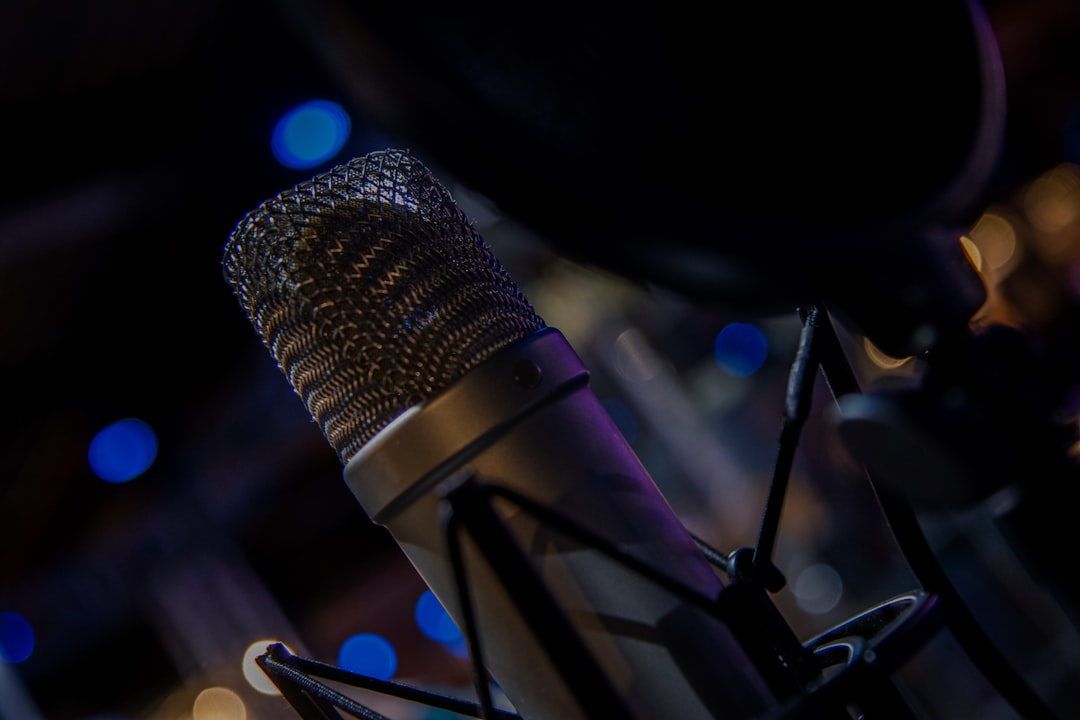
**Background Noise Interference in Audio Quality**
Background noise poses a considerable challenge for accurate Mandarin voice-to-text transcription. The presence of environmental sounds can significantly distort the audio quality, impacting the speech signal's power and the clarity of tonal variations, which are vital for understanding Mandarin. These disturbances can particularly hinder individuals learning Mandarin, as they may struggle to discern the intended meaning in the presence of noise more than native speakers. This is especially relevant when dealing with the nuanced tonal distinctions that are crucial in the language. Current research focuses on improving noise reduction methods within ASR systems to combat this problem. As we strive for higher accuracy in Mandarin transcription, particularly in 2024, it is essential that such noise mitigation strategies are effectively implemented. The push for greater accuracy in Mandarin transcription services necessitates the development of robust techniques to counteract the effects of noise, as ignoring them can compromise transcription quality and lead to inaccuracies.
1. **Noise's Impact on Mandarin Transcription:** Background noise, a pervasive issue in real-world audio, presents a significant challenge for transcribing Mandarin. Studies show that even seemingly innocuous white noise can substantially reduce accuracy, with drops of up to 30% reported. This is especially problematic for machine learning models primarily trained on pristine audio data.
2. **Frequency Masking in Tonal Languages:** The spectral characteristics of background noise can interfere with specific frequencies that are critical for identifying tonal shifts in Mandarin. This "masking effect" hinders the transcription system's ability to extract vital audio features, which can lead to inaccurate or incomplete interpretations of the speech.
3. **Human vs. Machine Noise Filtering:** Humans naturally filter out background noise using auditory context to focus on the important speech elements. This seemingly effortless ability relies on neural processes that haven't yet been fully replicated by automated transcription systems. Understanding these human mechanisms is a critical step towards improving machine performance.
4. **Dynamic Environments Pose a Challenge:** Real-world environments aren't static. They're filled with a dynamic array of background noise, from bustling crowds to passing traffic. These variable acoustic environments complicate the training of machine learning models, which often rely on more controlled and uniform datasets. This leads to weaker generalization of learned patterns to real-life situations.
5. **Microphone Sensitivity to Noise:** The quality and positioning of the microphone can significantly impact how background noise affects audio capture. While directional microphones can help mitigate extraneous sounds, they can also unintentionally limit the capture of softer or more subtle speech, particularly in more complex sound landscapes.
6. **Noise's Cognitive Burden:** Background noise increases cognitive effort during speech communication, leading to variations in how individuals speak. This unpredictability impacts both human interactions and machine learning algorithms, creating challenges for algorithms to adapt to those variations caused by noise.
7. **Neural Network Robustness to Noise:** Current neural network architectures can struggle with fluctuating noise conditions because they're often trained on limited datasets that may not represent real-world diversity. Even subtle changes in background sounds can cause drastic performance dips in transcription accuracy. Developing more robust and broadly trained models is crucial.
8. **Noise's Amplification of Tonal Confusion:** Background noise exacerbates tonal confusion, resulting in increased transcription errors. This is particularly detrimental when dealing with homophones, where the subtle differences in tone are crucial for distinguishing their distinct meanings.
9. **Real-Time Noise Reduction's Limitations:** While some transcription systems integrate real-time noise reduction algorithms, these approaches can struggle to effectively isolate speech from noise in the complex soundscape of tonal languages. The intricate nature of Mandarin tones makes it difficult to differentiate desired speech from noise effectively.
10. **The Perception Gap in Noise Handling:** Even if a transcription system performs internally robust noise reduction, the users' perception of background noise can affect their satisfaction. This perception gap highlights the need for transcription technology to consider not just machine-level accuracy but also a seamless user experience where noise interference is mitigated for the individual listener.
Common Pitfalls in Mandarin Voice-to-Text Transcription Improving Accuracy in 2024 - Adapting to Rapid Speech and Colloquialisms
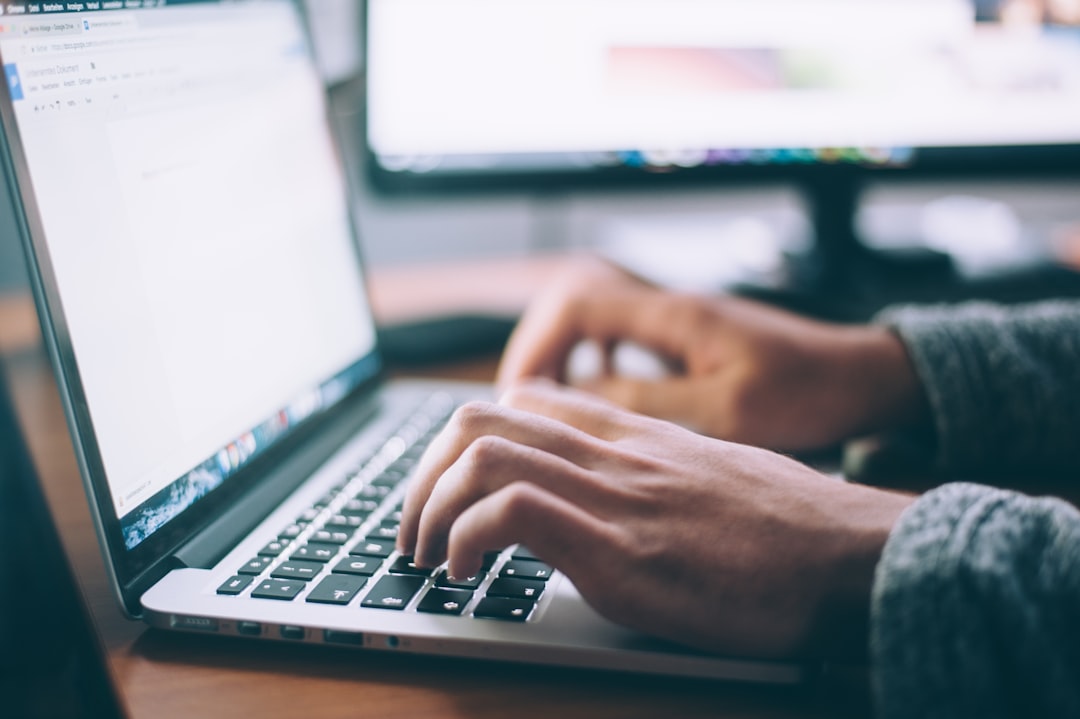
**Adapting to Rapid Speech and Colloquialisms**
Mandarin voice-to-text transcription often struggles with the fast pace and casual language common in everyday speech. When people speak quickly, the systems may miss words or misinterpret sounds, especially when combined with informal language that deviates from standard Mandarin. Many current systems aren't well-equipped to handle the natural flow and variations of spoken Mandarin, which can lead to inaccuracies in transcriptions. There's a clear need for better algorithms that can adapt to these conversational styles, including regional slang and informal phrases that are frequently used. It is important that these systems evolve to keep up with these changes, especially as Mandarin evolves and communication styles become more diverse. Overcoming these hurdles is essential for improving the accuracy and usefulness of automated transcription tools.
1. **Informal Language and Slang:** Mandarin is full of casual expressions and slang that are common in everyday conversations. However, these terms often don't appear in the training data for automated transcription systems. This mismatch can lead to errors when transcribing casual speech, as the systems might take these phrases too literally.
2. **Fast-Paced Speech:** Mandarin speakers, especially in informal settings, tend to speak quickly. Some studies suggest conversational speeds can surpass 300 words per minute, making it harder for systems to keep up and accurately capture the nuances of spontaneous conversation. This speed can increase transcription errors.
3. **Sound Reduction in Casual Speech:** In casual conversations, Mandarin speakers often simplify or blend syllables for a smoother flow. This can confuse transcription systems not accustomed to these speech patterns, leading to misinterpretations and incorrect transcriptions.
4. **Importance of Context:** The meaning of colloquial expressions depends heavily on the context they are used in. Social situations or the relationship between speakers can subtly influence the meaning. Transcription systems, lacking true contextual understanding, often miss these nuances and get the meaning wrong.
5. **Figurative Language:** Mandarin is rich in idiomatic expressions—phrases whose meaning isn't easily inferred from their literal components. Automated systems can struggle with these expressions and misinterpret their intended meaning, especially when dealing with culturally specific references often not covered in their training data.
6. **Pop Culture Impact:** Pop culture influences spoken Mandarin, with social media and entertainment trends quickly introducing new slang and colloquialisms. Because training data updates lag behind these rapid language shifts, transcription systems find it difficult to recognize and correctly interpret emerging terms.
7. **Multiple Meanings of Colloquialisms:** Many informal expressions have several meanings depending on tone, context, and the speaker's intention. This multiple meaning feature needs complex parsing algorithms, which haven't been fully developed in current transcription systems, thus creating inaccuracies in the output.
8. **Overlapping Speech and Interruptions:** Casual Mandarin conversation is often filled with interruptions and people talking over each other. This overlapping speech is challenging for automated systems because it is hard to differentiate between speakers, resulting in confused or incomplete transcriptions.
9. **Regional Variations in Slang:** Just as accents vary pronunciation, they also influence slang and colloquialisms. Transcription systems trained on one dialect might struggle to recognize slang from other regions. This highlights a need for training data that reflects the broader spectrum of Mandarin language use.
10. **Adapting to Language Changes:** Despite the difficulties with rapid speech and informal language, systems that use adaptive learning techniques can become more accurate over time. By continuously gathering speech data and incorporating user feedback, these systems can gradually learn the language preferences of their users and improve transcription quality.
Common Pitfalls in Mandarin Voice-to-Text Transcription Improving Accuracy in 2024 - Continuous Learning for Evolving Language Patterns
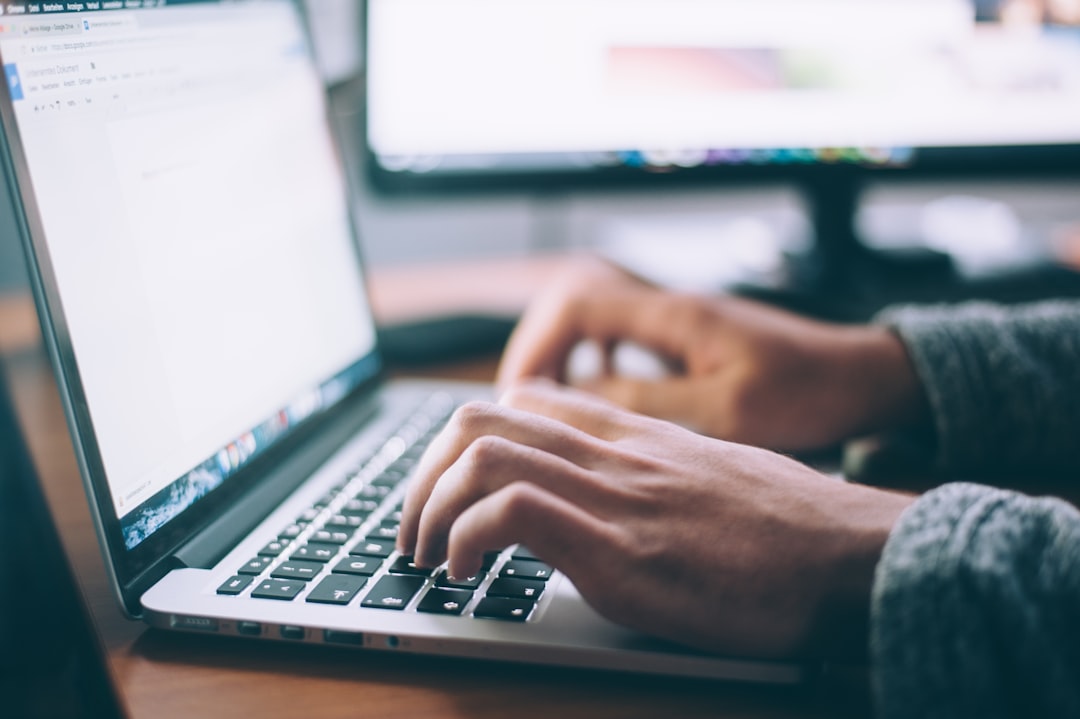
The dynamic nature of Mandarin necessitates a continuous learning approach for voice-to-text transcription systems to remain accurate. Mandarin's vocabulary, slang, and informal speech patterns are constantly changing as users interact with the language in daily life. This creates a significant challenge for maintaining high transcription accuracy as systems need to not only recognize varied regional accents and dialects, but also adapt in real-time to diverse speech patterns – including fast-paced conversations and subtle tonal shifts. The ability to incorporate user feedback is also crucial for improving machine learning models, helping transcription tools better adapt to the natural flow of spoken Mandarin. However, if these systems don't continuously adapt and refine their understanding, they run the risk of lagging behind in a language that is constantly evolving. Without ongoing adaptation, transcription accuracy can suffer, and user experience will ultimately decline.
1. **The Abundance of Homophones:** Mandarin presents a unique challenge with its high number of homophones – words that sound alike but have different meanings. It's estimated that nearly a fifth of Mandarin words can be homophones based on tone alone. This creates a huge challenge for transcription systems that rely primarily on sound for recognizing words.
2. **Tonal Nuances Across Regions:** Mandarin's tonal system, while consistent in its basic structure, experiences subtle regional differences. These differences, particularly in how tones are produced and shaped, can be significant. Automatic transcription systems often struggle to adapt to these local variations, leading to inaccurate interpretations of spoken language.
3. **Colloquialisms Need Context:** Informal language, frequently used in casual conversations, is heavily reliant on context for understanding its meaning. Everyday expressions can shift meaning depending on who's speaking and what they're discussing. For transcription systems to accurately interpret this language, they require a higher level of sophistication in understanding how words interact within different contexts.
4. **Rapid Speech: A Cognitive Load:** Fast speech patterns aren't just a problem for transcription; they also create a cognitive burden on speakers and listeners. It requires significant effort to maintain clarity and ensure understanding while speaking quickly. This shared burden can sometimes lead to speaker errors that complicate the transcription process even further.
5. **Emotional Tone Affecting Clarity:** A person's emotional state can significantly affect the quality of their speech. Stressful or emotional situations can impact pronunciation and tonal patterns. This creates a roadblock for transcription systems, which aren't equipped to interpret the emotional context of speech, making accurate transcription harder.
6. **Training Data Bias Towards Formality:** A large proportion of the existing training data used in Mandarin voice-to-text systems is based on formal language. This gap between the data and the diversity of real-world conversations leads to inaccurate interpretations of informal speech and casual language.
7. **Overlapping Speech's Challenges:** It's not uncommon for Mandarin speakers to interrupt each other in conversations, leading to situations where multiple people are speaking at once. This dynamic can cause confusion for transcription algorithms, which typically focus on a single audio stream at a time. Consequently, transcriptions can be fragmented or incomplete.
8. **Language Evolves Faster Than Models:** The speed at which new slang and informal language enters Mandarin, primarily fueled by social media and popular culture, far outpaces the ability of many transcription systems to keep up. These systems require ways to continuously learn and update their knowledge of current language trends.
9. **Lessons from Neuroscience:** Humans have developed an incredible ability to quickly filter out unwanted sounds and focus on relevant speech information. Our auditory processing excels at recognizing crucial language cues, but the field of artificial intelligence is still in its early stages of mimicking these abilities. There's vast potential for improving machine learning algorithms by studying the ways in which the human brain processes auditory information.
10. **Varied Speech Patterns:** Mandarin speakers frequently employ techniques to smooth the flow of conversation, such as blending or reducing sounds. However, this can confuse systems that are primarily trained on clear, separated speech elements. These fluent and varied speech patterns need to be accommodated in transcription systems through advancements in both speed and flexibility of recognition technologies.
More Posts from transcribethis.io: