Experience error-free AI audio transcription that's faster and cheaper than human transcription and includes speaker recognition by default! (Get started for free)
AI Transcription Tools The Evolution of Accuracy Rates from 2020 to 2024
AI Transcription Tools The Evolution of Accuracy Rates from 2020 to 2024 - The leap from 90% to 99% accuracy between 2020 and 2024
The jump from roughly 90% accuracy to near-perfect 99% accuracy in AI transcription between 2020 and 2024 represents a substantial achievement. This leap forward is a product of refining algorithms, leveraging increased computing power, and integrating more sophisticated machine learning techniques. The improved quality of audio recordings undoubtedly played a role, but the underlying advancements in AI are the key drivers. The widespread adoption of AI transcription tools across different fields suggests a strong correlation between accuracy gains and enhanced operational efficiency. While this progress is undeniably positive, it prompts contemplation regarding the ultimate potential of AI transcription. Can artificial intelligence ever truly achieve flawless accuracy, and what ramifications might that have for ensuring the integrity and reliability of transcribed information? The implications for trustworthiness and ethical use of this technology become more pronounced as accuracy approaches perfection.
The period between 2020 and 2024 witnessed a remarkable leap in AI transcription accuracy, moving from a generally accepted 90% to a more impressive 99% in select systems. This advancement wasn't a singular event, but rather a result of multiple factors converging. Researchers explored innovative neural network designs, including Transformer architectures, to better grapple with the complexities of language and context within audio. Simultaneously, the availability of much larger training datasets became crucial. These datasets encompassed a wide range of audio samples, covering different accents, dialects, and background noise levels, ultimately leading to more resilient and accurate transcriptions in diverse real-world situations.
Unsupervised learning techniques also played a vital role, empowering AI systems to learn from unlabeled data, which proved particularly useful in adapting to new languages and terminology without needing extensive manual intervention. The integration of real-time feedback mechanisms further refined accuracy, allowing models to learn from mistakes instantly and adjust their algorithms on the fly. This continuous learning process contributed significantly to the overall reduction in errors. Furthermore, the ability to personalize transcription models for individual users, taking into account unique speech patterns and preferences, minimized the impact of generic model limitations on accuracy.
Progress in Natural Language Processing (NLP) also fueled the accuracy surge. Transcription systems became better at distinguishing between words that sound alike but have different meanings (homophones) and interpreting context-specific language, leading to clearer and more accurate text outputs. The use of ensemble methods, where multiple AI models work in tandem to produce transcriptions, also enhanced reliability by reducing the likelihood of errors associated with any single model. Improvements in hardware, particularly the development of specialized AI chips, played a crucial part. These chips facilitated faster processing, allowing models to handle larger datasets in real time without compromising performance.
Finally, the rise of automated error detection and correction systems formed a robust safeguard against major mistakes. These systems could identify and correct transcription errors quickly, preventing inaccuracies from being propagated. As a final contributing element, greater transparency around model decision-making processes became a priority, allowing researchers to better identify and address patterns in errors, accelerating the drive towards that 99% mark. While we have reached a milestone with this level of accuracy, it's clear that the ongoing pursuit of improvement will necessitate continuous refinement and exploration of new techniques within the field.
AI Transcription Tools The Evolution of Accuracy Rates from 2020 to 2024 - Machine learning advancements driving improved speech recognition
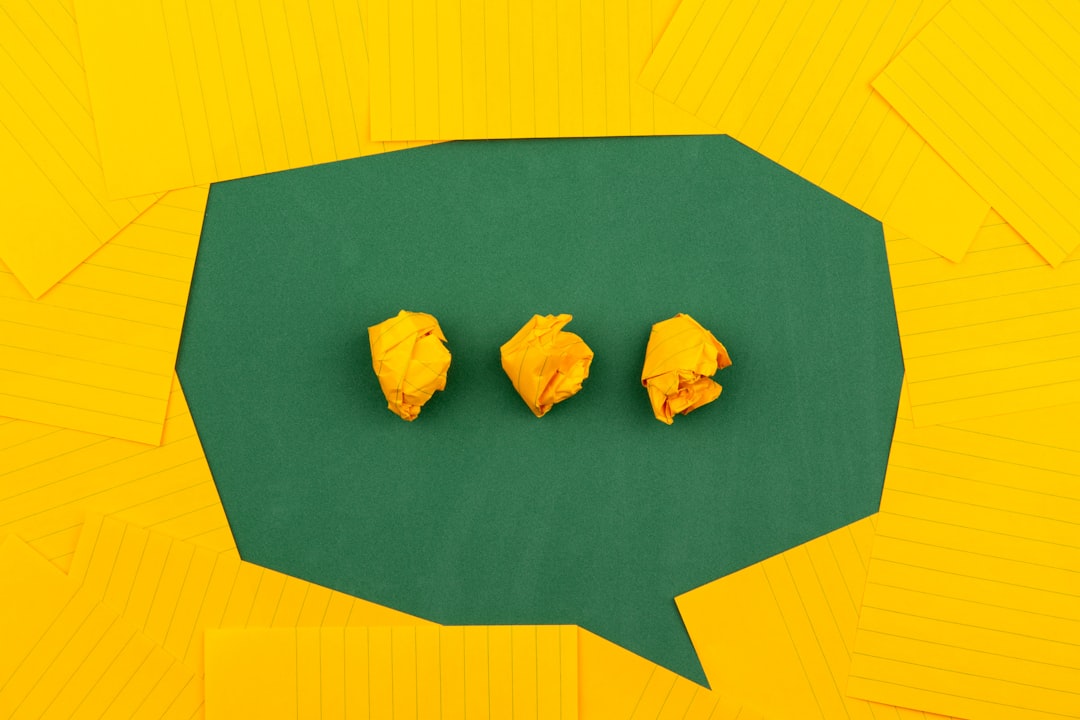
The period between 2020 and 2024 saw a surge in the capabilities of speech recognition, largely fueled by advancements in machine learning. AI transcription tools have benefited from the integration of more sophisticated algorithms, specifically deep learning approaches and neural networks. These improvements empower Automatic Speech Recognition (ASR) systems to analyze and interpret spoken language with remarkable precision. The push towards ever-greater accuracy is intertwined with the availability of expansive datasets that help the systems learn to cope with a wide array of accents and linguistic variations. Moreover, progress in natural language processing has been instrumental in enhancing the ability of transcription systems to grasp context and subtle language cues.
These advancements have undoubtedly brought about a new era of accurate and efficient transcription, yet this progress raises critical questions about the dependability and ethical use of such technology. As AI transcription systems inch closer to flawless accuracy, concerns regarding the integrity and trustworthiness of the transcribed information naturally increase. While these technologies are undoubtedly useful, it's crucial to acknowledge their limitations alongside their capabilities, as the journey towards truly perfect transcription continues.
The evolution of speech recognition over the past few years, particularly from 2020 to 2024, has been significantly propelled by advancements in machine learning. A major shift has been the development of models capable of not just recognizing individual words but also grasping the context in which they're spoken. This contextual understanding has led to a noticeable decrease in errors, especially when dealing with words that sound alike but have distinct meanings, a common challenge for earlier systems.
Furthermore, the integration of dynamic learning has enabled these systems to adapt to individual speakers in real time. This means that instead of relying on generalized data, the models can fine-tune their performance based on unique accents, speech patterns, and even vocal characteristics. This personalized approach has contributed significantly to increased accuracy.
Previously, a persistent challenge for speech recognition was handling noisy environments. Classical systems struggled to differentiate between speech and background sounds. However, recent innovations in neural network design have yielded models that are more effective at filtering out noise, making them significantly more robust in challenging audio conditions.
Another area of substantial improvement is the incorporation of sophisticated error correction mechanisms. These systems, driven by machine learning, can automatically pinpoint and rectify errors in transcriptions, ensuring a higher level of reliability. This automated quality control has become crucial in ensuring the integrity of the transcriptions produced.
We've also seen a growing reliance on unsupervised learning techniques. This approach empowers models to learn from massive quantities of unlabeled data. This approach has been especially valuable in adapting to new dialects and terminologies quickly, without requiring extensive human intervention.
The integration of multi-modal training data has been another key factor. By pairing visual information with audio, the models receive additional contextual clues, leading to a more comprehensive understanding of the speaker's intentions and the meaning embedded in the language.
The drive towards greater global applicability is also apparent. As more diverse datasets, including a wide array of accents and dialects, are incorporated into training, the models are becoming more universally adept at handling a broader range of linguistic variations. This trend towards global applicability makes the technology accessible across different regions and cultures.
The introduction of Transformer architectures has also dramatically improved the ability of these models to recognize and understand the intricate relationships within language. These architectures have played a crucial role in the significant gains in transcription accuracy we've seen.
Moreover, the advent of specialized AI hardware, such as tensor processing units, has dramatically accelerated processing times. This increased speed enables the models to analyze incoming audio in real time, without sacrificing performance, making real-time transcription more practical and efficient.
Finally, these advancements extend to the finer details of language. Sophisticated machine learning methods are now starting to address linguistic nuances, such as idioms and colloquial expressions, contributing to transcriptions that are more natural and contextually relevant. This increased sophistication in handling subtle language variations marks a significant step forward in achieving more human-like understanding of speech.
While the progress in speech recognition is encouraging, there's still a long way to go before achieving truly perfect accuracy. Understanding the intricate complexities of human language and its diverse manifestations continues to be a challenging research pursuit. However, the rate of progress in recent years strongly suggests that the future holds even more advancements in this critical area of artificial intelligence.
AI Transcription Tools The Evolution of Accuracy Rates from 2020 to 2024 - Real-time transcription capabilities emerge in 2022
The year 2022 saw a notable shift in AI transcription tools with the emergence of robust real-time capabilities. This advancement enabled the immediate conversion of spoken words into written text, providing users with a more streamlined workflow. These tools expanded their reach, offering support across a wide range of languages, sometimes exceeding 40, while boasting accuracy rates that approached 99% in ideal situations. Despite these improvements, the reliability of real-time transcription remained susceptible to variables like subpar audio, noisy environments, and diverse accents. Interestingly, many tools began incorporating features that allowed for real-time collaboration, where multiple users could work together on transcriptions. This feature reflects the increasing demand for seamless workflows in various settings. The ongoing development of these tools prompts critical reflection on their potential ethical implications and the inherent limitations in fully guaranteeing the reliability of automated transcriptions.
The year 2022 saw a notable shift in AI transcription tools with the rise of real-time capabilities. This meant that transcriptions were produced with minimal delay, a significant improvement for applications like online meetings where quick turnaround is crucial. It's interesting how these systems were able to adapt, because researchers started using a technique called transfer learning, where knowledge gained from one language or dialect could be applied to others. This helped improve the accuracy of models across a variety of speech patterns, and sped up the process of introducing transcription tools in new regions.
One of the breakthroughs in 2022 was the development of multi-speaker recognition. Many AI systems became capable of differentiating between different voices in group conversations. This capability is essential for tasks involving collaboration, like transcribing interviews or meetings with multiple participants. Another key aspect was the refinement of audio preprocessing. The AI models got better at separating speech from background noise, making the systems more resilient and reliable in real-world scenarios, where conditions often aren't ideal.
We also saw advances in how AI transcription systems handle context. They became much better at differentiating words that sound the same but have different meanings (homophones), and at understanding vocabulary within a particular context. This helped reduce misunderstandings that were common in earlier transcription tools. The use of real-time feedback mechanisms also started gaining ground. Systems began to adapt based on user corrections, effectively allowing users to train the AI and make it more accurate. It was also in this period that user customization options for transcriptions became more refined. Users were able to tailor the transcription settings to their own unique speech patterns and preferences, leading to more personalized and accurate results.
In addition, visual information began to play a more significant role in 2022. The models incorporated visual data to improve their understanding of what was being said. This approach was helpful in educational and training environments, where understanding the context of speech is critical. It was also the time when we saw the tighter integration of these tools with popular communication platforms. Users could easily access transcription services within the software they were already using, making it much simpler to use and incorporate into daily workflows.
However, with these advancements came discussions around the ethical implications of real-time transcription. As the technology became more widely used, there were growing concerns about user privacy and the security of transcribed data. It became clear that guidelines and best practices needed to be developed to ensure the technology was used responsibly. The progress in AI transcription technology was remarkable in 2022, paving the way for more sophisticated and widely used tools. However, it's equally important that we continue to grapple with the ethical aspects of this technology to ensure it benefits everyone responsibly.
AI Transcription Tools The Evolution of Accuracy Rates from 2020 to 2024 - Multi-language support expands from 10 to 50 languages
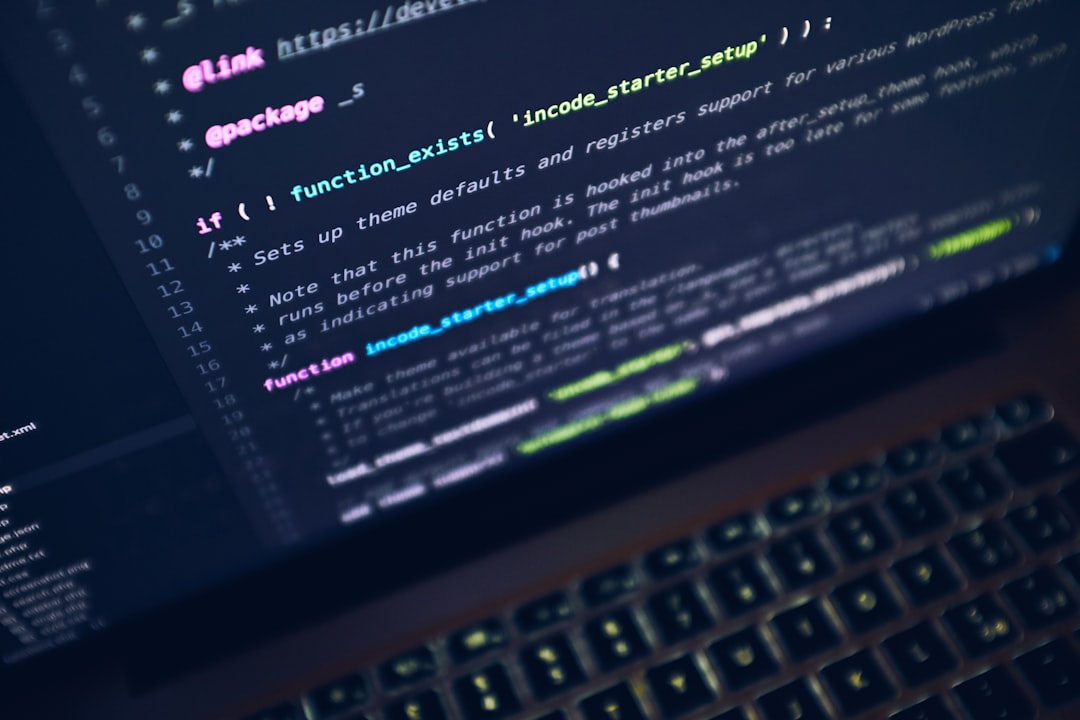
AI transcription tools have significantly expanded their language support, increasing the number of supported languages from a limited 10 to a much broader 50. This growth highlights a clear push towards broader global accessibility and usability. The ability to handle a wider range of languages, including various dialects and accents, has improved the overall performance of AI transcription, demonstrating a commitment to inclusiveness. While the progress is impressive, the maintenance of consistent high accuracy across such a vast linguistic landscape is challenging and requires continued improvements in the sophistication of AI algorithms and their ability to comprehend context. The implications of this rapid expansion raise important questions about the appropriate use of the technology and potential ethical considerations.
The expansion of multi-language support in AI transcription tools from a mere 10 to 50 languages represents a considerable leap. This 400% increase in linguistic coverage highlights a strong drive to make these tools accessible to a more globally diverse user base. However, this expansion comes with a growing set of complexities.
Supporting such a wide range of languages requires significantly more computational power. Each language presents its own unique set of phonetic, grammatical, and semantic structures, necessitating extensive retraining of the AI models. This increased complexity can strain the underlying algorithms and make them more susceptible to errors. Further complicating matters are the nuances embedded within each language. Regional dialects and cultural variations can subtly alter the meaning of words and phrases, potentially leading to errors in transcriptions if not carefully considered.
The accuracy of these multi-language tools relies heavily on having large amounts of training data. For each new language, unique datasets are needed, capturing the diversity of accents, background noises, and informal speech patterns. Gathering this kind of comprehensive data can be a challenging and resource-intensive endeavor. The issue of homophones – words that sound the same but have different meanings – becomes even more pronounced with the inclusion of multiple languages. This can lead to a rise in errors in contexts where homophones are prevalent.
When it comes to real-time transcription, difficulties arise with languages that have complex writing systems or intricate grammatical structures. Ensuring that the transcribed text is immediately readable and comprehensible can pose challenges. The push for user personalization, while beneficial, introduces another layer of complexity. Users from different linguistic backgrounds have varying speaking styles and accents. AI models must be capable of adapting to these individual patterns, and discrepancies can arise during this customization process.
Expanding the language support also introduces integration challenges. AI transcription tools must seamlessly interact with existing software and platforms across multiple languages. This necessitates a level of technical versatility that can be challenging to achieve. Furthermore, with an increased number of languages, the risk of errors accumulating grows, particularly in contexts where languages are intermingled. Mistranslations or misinterpretations stemming from inaccurate transcriptions become increasingly problematic.
Expanding into new languages also necessitates careful consideration of the ethical implications. While widening access, we must acknowledge the potential for biases embedded in training data to surface in translations. Ensuring that AI transcription tools are both accurate and fair in their handling of different languages becomes crucial. As AI transcription tools continue to evolve in this direction, it's clear that continuous research and refinement will be needed to address these complex challenges and ensure the technology is used responsibly.
AI Transcription Tools The Evolution of Accuracy Rates from 2020 to 2024 - Integration with video platforms becomes seamless by 2023
By 2023, AI transcription tools had become remarkably well-integrated with numerous video platforms, making them much easier to use and improving how people interact with them. This smoother integration was highlighted by tools with real-time editing capabilities and the capacity to transcribe in a variety of languages, making them accessible to more users. The addition of live transcription features in several platforms promoted more interactive and collaborative experiences, enabling users to engage together in real-time discussions. However, these improvements also brought into sharper focus questions about the accuracy of these automated transcriptions and their ethical implications across different settings, emphasizing the need for thoughtful consideration of how this technology is used as it becomes more interwoven into our daily communication patterns. While the ease of use provided by these integrations is valuable, we should remain mindful of their potential flaws and any inherent biases that might be present.
By 2023, we saw a significant shift in AI transcription with the integration into various video platforms becoming much smoother. This development has made the transcription process more streamlined. Users can now get text outputs directly from videos, eliminating the need for separate steps and potentially reducing frustration.
It seems like many video platforms have begun to adopt common interface standards (APIs), which has made it simpler for transcription tools to connect. This standardization is beneficial, as it reduces the workload for developers. They no longer have to write custom integration code for each platform individually.
Real-time transcription abilities within video platforms also took a leap forward. It's now possible to generate subtitles in real time, during live streams or video calls. This is a major advantage for accessibility, especially for people with hearing difficulties.
Additionally, more advanced speech recognition algorithms were incorporated into these video transcriptions. They're getting better at handling situations with multiple people talking at once, which was a difficult task for earlier systems. This improvement in handling overlapping speech is noteworthy, as it addresses a longstanding issue in transcription.
The ability to handle different video formats also expanded, as AI transcription became increasingly capable of transcribing content from gaming streams to corporate webinars. This broad application of the technology reflects a wider acceptance across various uses cases.
In 2023, transcription tools started using information from the video itself. They're becoming better at interpreting the visuals alongside the audio, improving their understanding of context. This extra contextual information can help distinguish between words that sound similar but have different meanings (homophones), and generally makes for better transcripts.
There has also been a rise of feedback loops within video platforms themselves. Viewers or content creators can now directly report mistakes or suggest edits within the video interface. This type of continuous feedback mechanism, in theory, should lead to a steady improvement in the quality of the transcriptions.
Multilingual support in video transcription has also grown substantially, with systems able to handle real-time translations into various languages. This trend is making video content more accessible to a global audience without needing creators to redo their subtitles for every region.
The increased use of video transcription has required the backend systems to be more robust. These systems have been upgraded to handle bursts of usage, preventing slowdowns during peak times. This ensures users get their transcripts quickly and efficiently.
Despite the impressive progress, security concerns around video transcription have started to gain traction. As the technology gets increasingly woven into video platforms, the risk of data breaches and misuse of private content has become a topic of discussion. It's a critical area that developers and users need to address in the future.
AI Transcription Tools The Evolution of Accuracy Rates from 2020 to 2024 - The impact of larger training datasets on accuracy rates
The connection between the size of training datasets and the accuracy of AI transcription tools is multifaceted. While larger datasets generally lead to better accuracy, the quality of the data is critically important. Low-quality data can negatively impact performance regardless of quantity. Furthermore, phenomena like "double descent" highlight a complex interaction between dataset size and the model's capability. In certain situations, this interaction can cause a temporary decrease in accuracy before further improvements materialize. It's noteworthy that even with relatively small, but high-quality, datasets, AI models can demonstrate strong performance. This observation challenges the common assumption that bigger datasets always lead to better results. This progression underscores the significance of not only gathering extensive amounts of data but also guaranteeing that it accurately and effectively reflects the characteristics of the transcription tasks being performed.
While larger training datasets have been a key factor in boosting the accuracy of AI transcription tools, the relationship isn't as straightforward as one might assume. Increasing the size of a training set doesn't always translate to proportional gains in accuracy, leading to questions about resource management in model development. There seems to be a point where simply adding more data becomes less beneficial, indicating a phenomenon known as diminishing returns. We've seen studies where a surprisingly small fraction of the original data was sufficient to achieve a high level of accuracy, prompting researchers to rethink the prevailing belief that bigger is always better.
One challenge with larger datasets is the inherent increase in noise and inaccuracies within the data itself. As a dataset grows, there's a greater chance of encountering irrelevant or flawed information. If these issues are not addressed effectively, the model's ability to perform accurately could be negatively affected during training. It's important to acknowledge that simply increasing data quantity without addressing quality issues can be counterproductive. We've learned that data quality and careful annotation are crucial for model performance, often producing better results than simply piling on more data points.
Furthermore, we must be cautious of inadvertently amplifying biases that may be present in the training data. If a dataset lacks sufficient diversity, the trained AI model may struggle with categories that are underrepresented, limiting its applicability in various contexts. It's a bit like teaching a child about the world based solely on one neighborhood. The child might have a good grasp of that specific environment but will lack the broader understanding needed to interact confidently in diverse settings. This highlights a tension between generalizing model capabilities across many situations versus achieving high accuracy in more specific niches.
The pursuit of larger datasets also has implications for model latency, particularly in real-time applications. The larger the dataset, the more processing power is generally required for the model to perform inferences efficiently, potentially slowing down tasks. This trade-off between greater accuracy and potential delays is something we need to carefully consider when developing these AI systems.
Another crucial factor is that simply applying transfer learning from one large dataset to another isn't a guaranteed recipe for success. The underlying characteristics of the data must align for the technique to be effective. We need to be selective about what we transfer and ensure it's relevant to the specific task at hand. Also, as we train on increasingly larger datasets, we've found that AI models need to continuously refine their learning mechanisms. It's not just about incorporating new information but also re-evaluating and potentially discarding inaccurate patterns that may have been learned in earlier stages of training.
Beyond the technical considerations, using large datasets raises complex ethical considerations. We've encountered concerns about data privacy and user consent when gathering extensive amounts of information. Ensuring responsible data practices and establishing clear ethical guidelines for collecting, storing, and using these datasets is vital for preventing harmful outcomes and upholding user rights. The journey towards ever-increasing accuracy in AI transcription is tied to the continued development and refinement of techniques for managing these larger datasets in a manner that is both effective and responsible.
Experience error-free AI audio transcription that's faster and cheaper than human transcription and includes speaker recognition by default! (Get started for free)
More Posts from transcribethis.io: