Experience error-free AI audio transcription that's faster and cheaper than human transcription and includes speaker recognition by default! (Get started for free)
AI-Powered Noise Reduction in Online Audio Editing A 2024 Analysis
AI-Powered Noise Reduction in Online Audio Editing A 2024 Analysis - AI Noise Reduction Accuracy Improvements in 2024
The landscape of AI-powered noise reduction has seen notable strides in 2024, with a stronger emphasis on accuracy and detail preservation. Tools are evolving beyond basic noise suppression, now focusing on maintaining intricate features within audio or images while selectively targeting unwanted sounds. We see examples like ON1 NoNoise AI refining its noise reduction models, specifically to retain detail in challenging areas while also offering enhanced selection tools like Quick Mask AI. The push for higher quality audio across different uses has driven the development of specialized services like Cleanvoice, particularly useful in fields like call centers, or Mediaio's Noise Reducer, tackling diverse audio issues like wind or hum. The market for AI noise reduction is indeed growing, with new features and implementations constantly being introduced. However, it's important to remember that the 'best' solution varies, depending on the specific task and context of the audio editing being done. While these advancements are promising, it's crucial to remain mindful that a universal, perfect noise reduction solution has yet to be achieved, requiring users to choose tools based on their individual needs.
The landscape of AI-powered noise reduction has seen a surge in accuracy throughout 2024, with various tools showcasing refinements to their underlying algorithms. While the core concept of utilizing AI to isolate and remove noise has been established, we are seeing a shift towards more nuanced and adaptable systems. This is particularly evident in tools like ON1 NoNoise AI, which has advanced its models to pinpoint specific details within images, implying the ability for greater precision in the frequency domain for audio as well. This development suggests that AI is beginning to understand complex audio textures more effectively.
The ability to target specific areas or frequencies within audio for noise reduction has become increasingly sophisticated. The Quick Mask feature in ON1 NoNoise AI and the frequency-mapping algorithms incorporated in other tools illustrate this trend. While this targeted approach is promising, it raises the question of whether we are reaching the limits of what can be achieved without introducing unwanted artifacts in the target audio. It is interesting that there are efforts being made to balance noise reduction with the preservation of the nuanced sonic characteristics of the original recording.
The range of applications for AI noise reduction has also expanded. Tools like Cleanvoice, catering to call centers, and Utterlyapp, focusing on online meetings, highlight the demand for AI-powered noise reduction in diverse communication contexts. It remains to be seen whether such specialization will ultimately lead to more versatile and capable general-purpose noise reduction tools or further fragmentation within the field.
Additionally, the development of AI noise reduction has sparked interest in how these tools should be used ethically. The potential for manipulation and questions of authorship when manipulating audio content are important considerations. While AI tools are increasingly effective at removing noise, it's crucial to be mindful of the implications of manipulating audio content, especially in settings where clear communication is paramount.
Overall, while the advancements in AI noise reduction are impressive, further research is needed to thoroughly understand the limitations and broader societal consequences of these technologies. It is a dynamic area where both technical innovation and ethical consideration are crucial aspects.
AI-Powered Noise Reduction in Online Audio Editing A 2024 Analysis - Integration of Machine Learning Models for Real-Time Audio Processing
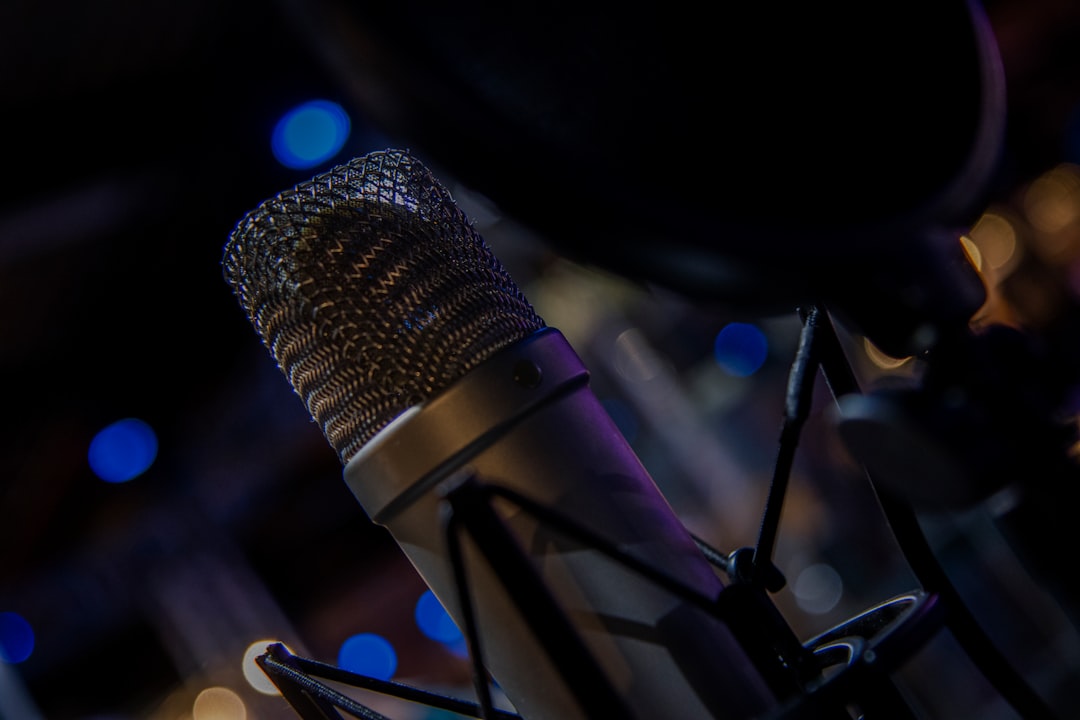
The integration of machine learning models into real-time audio processing is a rapidly developing field, with applications like noise reduction in online audio editing at the forefront. Recent advancements rely heavily on deep learning, offering solutions that enhance speech quality while simultaneously suppressing unwanted background noise in real-time. These improvements are particularly relevant to the field of automatic speech recognition, especially when tackling the complexity of multilingual communication in virtual meeting settings. However, alongside these exciting developments, the complexity and resource demands of training these sophisticated models continue to be a significant hurdle. Moving forward, researchers and developers will need to navigate this inherent tension between achieving optimal performance and managing the resource requirements of advanced models for effective implementation. This careful balancing act is crucial to the future of this field.
The integration of machine learning models into real-time audio processing is accelerating, particularly in areas like online audio editing where noise reduction is critical. Recent advancements in AI-driven noise reduction often involve deep learning, aiming to improve speech quality and suppress unwanted noise in dynamic environments. We're also seeing this applied to enhance automatic speech recognition (ASR) systems, for instance, in virtual meetings where real-time translation addresses language barriers. There's a growing interest in using large language models for audio signal processing, as highlighted by a recent review paper, which is fascinating. The MacawLLM model, for example, is designed for handling diverse data types, indicating a push towards more flexible audio processing pipelines. However, it seems that general-purpose models like Whisper, though trained on massive datasets, haven't quite surpassed specialized models tailored for specific tasks like those tested on the LibriSpeech benchmark in speech recognition.
Research suggests that newer deep learning models are becoming more viable options for real-time speech enhancement, showing clear improvements in processing capabilities. However, a consistent challenge across the field is balancing peak performance with the complexity of training deep learning models for particular audio tasks. Some of the early applications of deep learning in noise reduction, dating back to 2015, explored techniques like regression methods to generate frequency-specific audio masks. The progression of audio processing increasingly mirrors the advancement seen in fields like computer vision and natural language processing, hinting at a wider potential for machine learning within audio.
One notable development in real-time audio processing is the reduction of latency in machine learning-based systems, with some now handling audio in less than 10 milliseconds, which is crucial for live scenarios. Furthermore, many noise reduction systems have shifted to adaptive algorithms, capable of learning and reacting to the environment, allowing them to differentiate between desired and unwanted audio, even as the soundscape changes. These models are often trained on vast datasets with diverse audio types, helping them recognize and isolate various sounds, from human voices to a wide range of environmental noises. It's interesting how a transition has occurred from focusing primarily on the time-domain in early noise reduction methods to a greater emphasis on frequency-domain manipulation in modern real-time systems. This more nuanced approach offers the potential for precise noise filtering without negatively impacting the core audio content.
Some systems even incorporate feedback loops, where the model continuously analyzes its performance and iteratively adapts based on user feedback or new data, resulting in gradual improvements over time. A major hurdle still lies in the inherent complexity of audio signals. Sounds can be a mixture of overlapping frequencies and harmonics, presenting a challenge for models aiming to effectively disentangle these intricate mixtures. Luckily, improvements in machine learning have also made these models more resource-efficient, enabling broader accessibility on standard hardware. Many tools offer customizable settings, allowing users to control the level of noise reduction, indicating a growing awareness that the acceptable level of audio artifacts varies based on the specific application.
However, the power of noise reduction raises ethical questions, particularly in areas like journalism and documentation, where audio fidelity can directly influence how we perceive information and trust the source. The potential for manipulation becomes more prominent with highly effective noise reduction tools. Ultimately, the performance of noise reduction algorithms can vary across diverse environments and sound types, emphasizing the importance of ongoing rigorous testing to ensure these technologies are reliable and consistent in various settings.
AI-Powered Noise Reduction in Online Audio Editing A 2024 Analysis - Comparative Analysis of Leading AI-Powered Noise Reduction Tools
The field of AI-powered noise reduction tools is experiencing a period of rapid growth in 2024, with a diverse range of options emerging to tackle various audio challenges. Tools like Audiodenoise.com demonstrate proficiency in removing unwanted background noise from raw recordings, offering a valuable resource for those working with unrefined audio. Meanwhile, platforms like Utterlyapp focus on improving the clarity of online communication by minimizing background interference, making them particularly useful for virtual meetings and recordings.
While advancements in deep neural networks (DNNs) are evident in many of the newer tools, there's a notable gap in thorough comparisons between the performance of these AI-powered approaches and established traditional methods for noise reduction. This lack of comprehensive evaluation indicates a need for more research to properly assess the strengths and weaknesses of each approach. Tools like DaVinci Resolve and Topaz Labs DeNoise AI highlight the ability to integrate these powerful AI models, particularly when addressing the combined complexities of image and audio noise within media.
Although these technological developments are positive, it's crucial to remember that the perfect noise reduction solution remains elusive. The wide variety of noise types and audio scenarios encountered makes finding a universal solution incredibly difficult. As the field continues to evolve, users should be aware that the most effective tool will always depend on their specific audio editing needs and context.
Several AI-powered noise reduction tools employ sophisticated methods like spectral gating, effectively isolating and reducing unwanted frequencies while preserving the desired audio. This highlights a deeper understanding of audio frequency manipulation within the field.
Many tools are designed to identify specific noise types, such as hiss or hum, through training on categorized audio datasets. This classification approach allows them to distinguish between different auditory elements, improving their accuracy in targeting undesirable sounds.
However, the effectiveness of these tools can be significantly challenged in scenarios with complex audio layering. Handling multifaceted soundscapes remains a hurdle, prompting the need for continuous improvement in algorithm design to address this limitation more effectively.
Real-time noise reduction systems have made significant strides in reducing processing delays. With latency often below 10 milliseconds, these systems are becoming increasingly viable for live audio applications. This is a testament to the synergy between computational efficiency and adept signal processing.
Some more advanced noise reduction tools utilize adaptive learning mechanisms that dynamically modify their approach based on user feedback or other inputs. This presents a fresh perspective on preserving audio integrity while removing unwanted background noise.
The continuous evolution of noise reduction often focuses on mitigating audio artifacts, which are unintended distortions introduced during processing. This ongoing effort to balance noise reduction effectiveness with audio quality poses a major challenge for engineers in the field.
The ability of AI to significantly modify audio raises ethical concerns, especially in applications like journalism. Altered audio can easily mislead audiences, emphasizing the necessity of transparency in audio editing practices.
While numerous general-purpose AI models exist, specialized noise reduction algorithms have often outperformed them in benchmark evaluations. This challenges the practicality of universal solutions for audio processing, suggesting a need for tailored approaches depending on the specific use case.
The shift from primarily analyzing audio in the time domain to focusing more on the frequency domain reveals a growing comprehension of the intricacies of audio signals. This advancement has led to more precise and nuanced methods for noise reduction.
Improvements in the resource efficiency of machine learning models are democratizing access to high-quality noise reduction tools. This means users with standard computing equipment can now participate in professional-grade audio editing, opening up opportunities in this field.
AI-Powered Noise Reduction in Online Audio Editing A 2024 Analysis - Impact of AI Noise Reduction on Online Collaboration and Remote Work
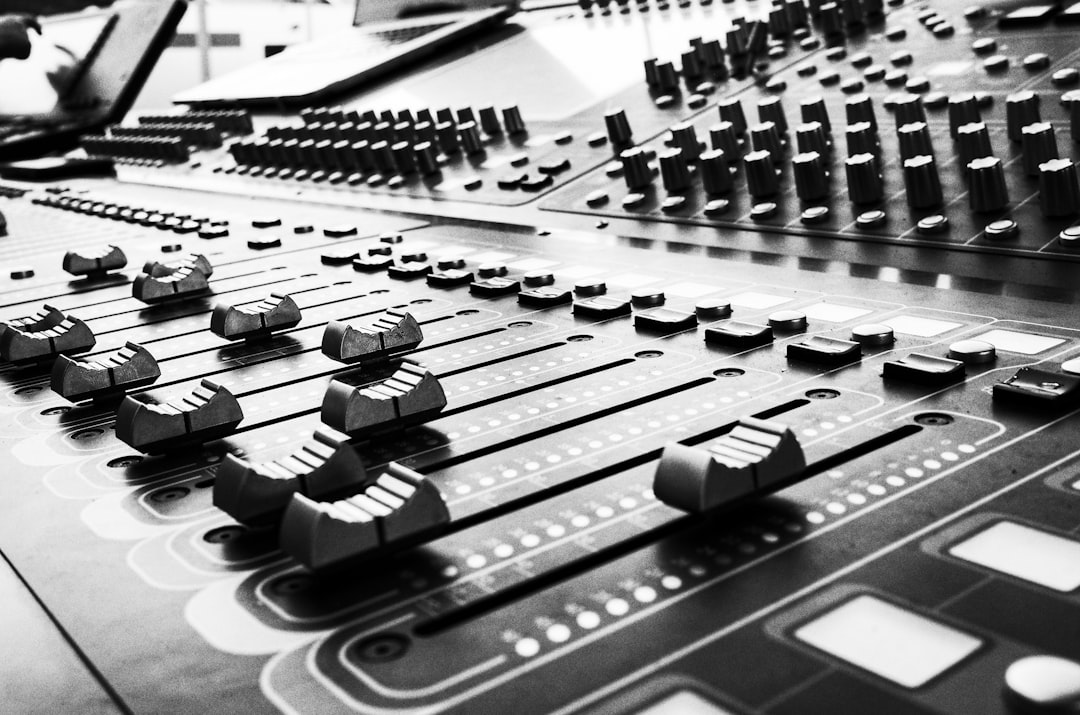
AI-powered noise reduction has significantly improved the landscape of online collaboration and remote work by enhancing communication clarity. This improved clarity is vital for maintaining productivity and fostering effective teamwork within remote environments. The reduction of background noise leads to smoother interactions, minimizing misunderstandings and boosting overall productivity among team members. The shift towards remote work, particularly accelerated during the COVID-19 pandemic, highlighted the need for robust noise reduction tools, supporting virtual interactions and keeping remote workers engaged and connected.
The ongoing advancements in deep learning are allowing for more refined noise-filtering capabilities within these tools. This improvement promises to lead to an even more seamless virtual collaboration experience, raising the quality of online meetings and interactions. However, despite these advancements, the intricate nature of audio processing remains a hurdle. The challenge of preserving audio integrity while effectively eliminating unwanted noise requires continued vigilance. Ensuring the accuracy and reliability of AI noise reduction technologies remains a critical factor for maintaining the quality and trustworthiness of communication within online collaboration.
The realm of AI-powered noise reduction is rapidly evolving, particularly impacting the landscape of online collaboration and remote work. We're seeing a trend towards more adaptable algorithms that can dynamically learn and adjust to different audio environments in real-time. This is crucial for remote settings where sound profiles constantly change.
One fascinating development is the dramatic decrease in processing delays. Latency in many real-time systems is now below 10 milliseconds, which is essential for smooth, uninterrupted online interactions. This improvement makes AI noise reduction a more viable option for live virtual meetings and other remote collaborations.
These systems are becoming more adept at handling the intricate complexity of overlapping sounds. They can more precisely filter out background noise while preserving the clarity of speech, a key benefit in a remote work context with numerous audio sources.
Interestingly, the computational demands of training these advanced AI models have decreased, making them more accessible on standard hardware. This broader accessibility lowers the barrier for remote workers who want to use high-quality audio editing tools without needing specialized equipment. This democratization of technology is a significant development.
We're also observing a trend towards specialization in noise reduction tools. Tools like Cleanvoice and Utterlyapp, designed for specific use cases like call centers and virtual meetings, demonstrate that understanding the context of the audio is vital for effective collaboration.
The field has shifted from primarily focusing on analyzing audio in the time domain to working more within the frequency domain. This transition suggests a deeper understanding of how audio signals function, leading to more sophisticated and nuanced methods for removing noise while maintaining audio quality.
Many contemporary noise reduction tools offer customized user settings. This is a recognition that the optimal amount of noise suppression varies depending on the situation. A business meeting might require more stringent noise reduction than a casual conversation.
However, the effectiveness of these AI tools also raises ethical considerations, especially concerning transparency. With the ability to so precisely alter audio, it's important to be mindful of how this can influence communication, particularly in settings where accuracy and accountability are paramount.
Furthermore, the improved clarity of audio brought about by noise reduction is a major benefit to automatic speech recognition (ASR) systems. These systems, increasingly utilized in multilingual virtual meetings, rely heavily on clean, clear audio to accurately translate and process speech, making collaboration across language barriers smoother.
Although the advancements in noise reduction are significant, the introduction of unwanted audio artifacts remains a concern. As we push for higher levels of noise reduction, ensuring that the original audio isn't distorted or altered in unintended ways becomes increasingly challenging. This delicate balance between effectiveness and audio quality will require continued research and development.
AI-Powered Noise Reduction in Online Audio Editing A 2024 Analysis - Ethical Considerations in AI-Enhanced Audio Editing
The increasing sophistication of AI-enhanced audio editing tools, particularly those focused on noise reduction, brings to the forefront a range of ethical considerations. The use of personal data in training AI models raises significant concerns about privacy and security. Ensuring the responsible handling and protection of this data is paramount to prevent potential misuse. Additionally, the capacity of these AI tools to manipulate audio content raises questions about authenticity and potential for deception. This is especially relevant in fields like journalism and documentation, where accurate and reliable audio is crucial for maintaining trust and informed decision-making. The challenge is to balance the technical advancements in AI with a robust ethical framework that promotes transparency and accountability in the audio editing process. This ensures users, whether creators or listeners, can trust the integrity of the audio they encounter. As AI-powered audio editing becomes more prevalent, ongoing conversations about its ethical implications are crucial for navigating the potential benefits and risks associated with these powerful technologies.
Exploring the ethical landscape of AI-enhanced audio editing reveals a number of interesting questions and potential concerns. While the improved clarity achieved by these tools can be beneficial, it's crucial to consider how this clarity might be perceived by listeners. For example, excessively clean audio might remove natural cues or ambient sounds, potentially leading to a sense of artificiality or an inaccurate portrayal of the original audio. This 'misleading clarity' could have unintended consequences, especially when presenting information or experiences that depend on a natural soundscape for interpretation.
Ownership and rights surrounding audio content become a bit more complex when AI tools are used. If an AI model modifies or enhances a recording, it's not entirely clear who can claim authorship or ownership of the final product. This question of intellectual property can become particularly relevant in creative fields where the original form of an audio clip is crucial for defining the artist's intent.
The nature of AI-powered audio enhancement can also lead to cognitive dissonance. When the background sounds are meticulously removed, listeners might encounter an unexpected and unsettling listening experience, lacking the familiar natural ambiance they would typically expect. This disconnect can potentially hinder listener engagement and create a jarring or unpleasant experience.
Further complicating this ethical landscape is the potential for automated bias within these AI systems. If the models are mainly trained on audio from specific groups or demographic, they may inadvertently favor those characteristics when processing audio from different backgrounds. This can lead to disparities in quality, potentially affecting how diverse groups are portrayed or perceived.
The ability to easily manipulate audio raises the specter of misinformation. Especially in fields like journalism or legal contexts where accurately recorded audio is fundamental for determining truth, AI tools that enable audio alterations could be used to misrepresent conversations, create misleading narratives, or create distortions of evidence.
The use of feedback loops in some AI systems can also present a unique ethical challenge. While such loops can lead to continuous improvements in noise reduction performance, they also potentially risk reinforcing any existing biases or issues present in the training data. Over time, this could lead to a gradual decline in the fidelity of audio edits or introduce unwanted distortions.
Even with considerable advancements in real-time audio processing, the speed of processing can still be limited by the tools' need to perform noise reduction accurately. Maintaining quality while minimizing delay remains a challenge, prompting a need to carefully evaluate any trade-offs made.
These tools make it much easier than ever to manipulate recordings in a subtle or major way, which is concerning in media creation. Audio of interviews or events could be significantly altered, without the audience knowing, giving the impression of a reality that doesn't actually exist.
The complexity of the AI techniques underlying these tools can also lead to a knowledge gap between tool users and the capabilities of the tool itself. It's important for individuals who work with audio, to fully grasp how these tools modify the audio, in order to use them responsibly and be able to explain their impact.
As AI noise reduction tools become more common, they could diminish traditional audio engineering skill development. If reliance on these tools becomes too dominant, it's possible that future generations of audio engineers may have fewer opportunities to develop traditional skills for analyzing and resolving issues with audio.
These are just a few initial observations on the complex ethical considerations associated with AI-enhanced audio editing. As the technology progresses, understanding these factors and adapting our use of the tools will be necessary for ensuring their responsible implementation and preventing any unintended or unethical outcomes.
AI-Powered Noise Reduction in Online Audio Editing A 2024 Analysis - Future Trends in AI-Driven Audio Enhancement Technologies
The field of AI-driven audio enhancement is evolving rapidly, with several trends emerging in 2024 that promise to transform how we edit and experience audio online. We're seeing a growing focus on creating more immersive audio environments, with technologies capable of simulating 3D soundscapes that enhance user engagement. Furthermore, AI is increasingly adept at automatically identifying and correcting audio flaws. Tools employing deep learning, like SonicEnhance AI, are capable of handling tasks like noise reduction, volume normalization, and clarity enhancement, making it easier for content creators to achieve high-quality audio for various platforms. While established companies like Adobe and Waves are continually improving their professional-level audio editing tools, we're also seeing a rise in accessible and user-friendly AI-powered solutions like LALALAI and Veedio. This trend democratizes audio editing capabilities, allowing a broader audience to participate in improving the quality of their audio recordings. Despite these advancements, challenges remain in achieving the ideal balance between effectively removing noise and ensuring the overall quality and integrity of the audio remains intact. This constant tension highlights the need for ongoing research and assessment of these AI-driven tools to ensure their ethical and effective implementation.
The field of AI-driven audio enhancement is poised for further evolution, with exciting new directions emerging. We can expect to see a greater emphasis on generative models, capable not just of noise reduction but also of audio synthesis, potentially filling in gaps or missing audio segments to improve the overall listening experience. This development suggests a more holistic approach to audio quality.
Adaptive noise reduction algorithms are another promising area, with researchers focusing on developing systems that can dynamically learn and adjust to specific user environments. Tools are moving beyond generic noise suppression, aiming to create personalized profiles based on the soundscapes where the tools are typically used. This targeted adaptation could lead to a significant improvement in performance across a range of audio scenarios.
Future advancements in frequency-specific filtering are also likely. The next generation of AI-powered noise reduction will probably be adept at selectively suppressing specific frequency ranges, which is crucial for preserving the fidelity of the original audio. This ability to finely tune noise reduction could be a significant improvement over current methods.
The convergence of AI and augmented/virtual reality is an intriguing area to watch. Imagine VR and AR experiences that dynamically adapt the soundscape in response to a user's environment, effectively filtering out distracting real-world noise while amplifying desired sounds for a truly immersive experience. However, it is unclear what long-term impacts this type of dynamic filtering could have on users.
Accessibility to advanced audio tools is increasing. As AI noise reduction matures, developers are focusing on delivering these capabilities to users with standard hardware. This broader accessibility could democratize audio editing, allowing individuals without specialized equipment to create high-quality audio.
User-centric design will likely play a bigger role in future audio enhancement technologies. Some tools could incorporate feedback mechanisms that allow users to fine-tune the noise reduction in real time, dynamically adjusting the algorithm's behavior during use. This could potentially lead to even more intuitive interfaces that evolve alongside user interactions.
The ethical considerations around AI-enhanced audio remain a critical concern. As the tools become increasingly sophisticated, it's imperative that clearer guidelines are developed to ensure transparency and prevent potential abuse. Especially in domains like journalism and legal settings, where accurate audio recordings are paramount, we must be vigilant about the risks of altered audio being used to distort the truth.
There's a growing body of research exploring how heavily processed audio impacts listeners. Studies suggest that overly "cleaned" audio can lead to feelings of disconnection or discomfort. This is an interesting area that could have a major impact on how we design future audio enhancement technologies.
AI audio enhancement is already reaching beyond traditional media applications. Fields like healthcare are exploring how these tools can improve the clarity of patient communications, while education is experimenting with using them to enhance online learning experiences. It's likely these applications will expand into other industries as the capabilities of AI-powered noise reduction continue to mature.
Lastly, the diversity of training data in AI models needs careful attention. If the datasets used to train these algorithms lack a diversity of audio sources, the resultant tools might exhibit biases in audio processing, potentially impacting how different voices and languages are processed. Promoting a broader and more inclusive training data set would help in addressing these challenges.
It's clear that the future of AI-driven audio enhancement is rich with potential. However, it's equally important to acknowledge the need for continued research and responsible development to maximize the benefits of these technologies while addressing potential risks.
Experience error-free AI audio transcription that's faster and cheaper than human transcription and includes speaker recognition by default! (Get started for free)
More Posts from transcribethis.io: