Experience error-free AI audio transcription that's faster and cheaper than human transcription and includes speaker recognition by default! (Get started for free)
AI Adoption in Tech Sector Reaches 63% Analysis of Industry-Specific Implementation Rates Through 2024
AI Adoption in Tech Sector Reaches 63% Analysis of Industry-Specific Implementation Rates Through 2024 - Tech Giants Microsoft and Google Drive 63% AI Adoption Rate Across Software Development
Leading technology companies like Microsoft and Google have seen a substantial jump in AI implementation within their software development workflows, reaching a 63% adoption rate. This adoption surge points towards a broader acknowledgment of AI's ability to revolutionize software creation, leading to more efficient processes and the development of innovative products. However, the path hasn't been without bumps. Obstacles such as a shortage of AI-skilled workers and the intricacies of managing large datasets remain key issues. The recent emergence of generative AI has spurred organizations to rethink their operations, using these new tools to modify their processes. It's important to note that the rapid growth of these AI tools also comes with ethical considerations that still need to be addressed. Moving forward, the way AI integrates into the software development life cycle will continue to be a central theme as companies grapple with these new possibilities.
By late 2023, a remarkable 63% of software development activities at tech giants Microsoft and Google involved AI integration. This isn't just limited to coding, though. It's fascinating how AI has been absorbed into project management and automation, making the entire software development process smoother. It's almost as if AI has become the new "normal" in software development, impacting everything from initial planning to the final product release.
This widespread use of AI has altered hiring practices. Businesses are actively searching for engineers with not just traditional programming skills, but also a grasp of AI concepts. This shift highlights the evolving demands of the tech workforce.
While the advantages of AI are apparent, there are still reservations about relying too heavily on AI tools, especially when it comes to debugging and understanding the ‘how’ and 'why' behind the code. We're seeing a concern that depending too heavily on AI might actually hinder the development of core engineering skills.
But there are some clear benefits. One example is software testing where, remarkably, the use of AI-powered testing has shown a 25% reduction in errors. This strongly suggests that AI can enhance quality and reliability throughout the development process.
Naturally, this increased AI integration has brought with it questions about bias, transparency, and ethical considerations. Developers are starting to call for clear guidelines to ensure that AI is used responsibly within their workflow.
It's interesting to see how AI is breaking down traditional silos. We are starting to see engineers work more closely with data scientists and AI specialists to craft better, more efficient solutions.
The financial impact is also intriguing. Companies implementing AI-driven tools are seeing faster project completion times and, as a result, are experiencing a 20% average revenue growth from quicker time-to-market.
However, while leading the AI integration race, Microsoft and Google also face their own set of hurdles. Some engineers have concerns about the increased complexity in the codebase as AI features are added, potentially impacting software performance.
Lastly, this fast-growing trend is starting to redefine educational requirements for future engineers. Universities and technical schools are updating their programs to include AI literacy, recognizing that these skills will be essential for future job success. It is clear that the future of software development will be intricately woven with the use of AI, and future generations of engineers need to be prepared.
AI Adoption in Tech Sector Reaches 63% Analysis of Industry-Specific Implementation Rates Through 2024 - Machine Learning Applications Transform Data Processing at Financial Services Firms
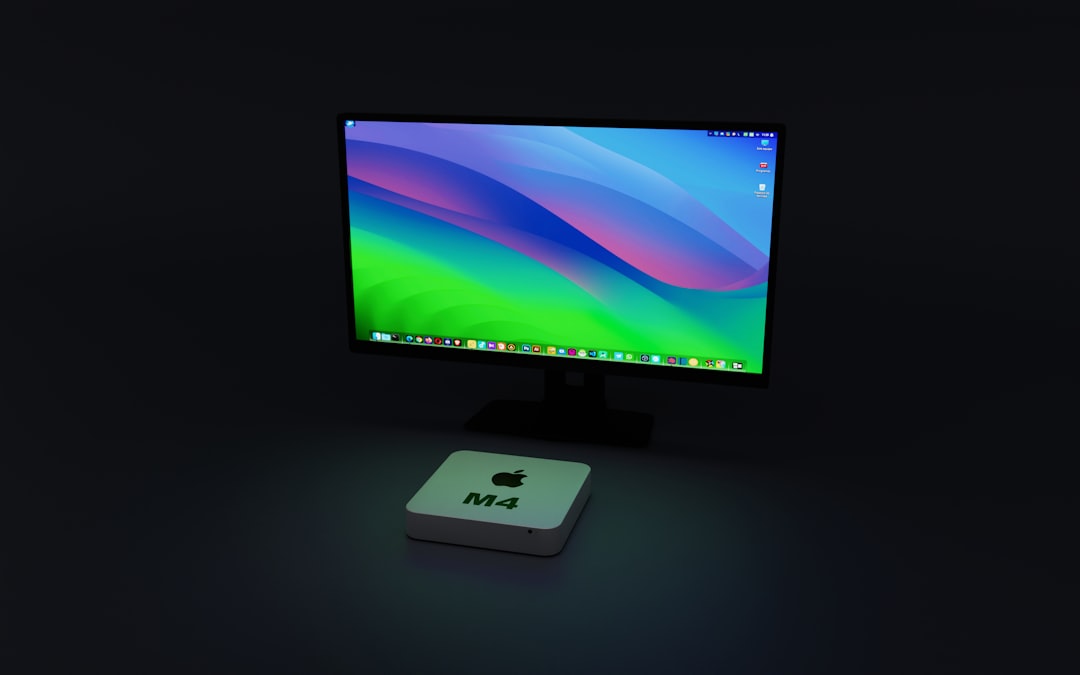
Financial institutions are increasingly leveraging machine learning to transform their data processing capabilities. This shift is driven by a need to streamline operations, personalize customer interactions, and better manage risk. We're seeing a move away from just exploring AI's potential and into a phase of broad implementation. Many firms now rely on machine learning for essential tasks, demonstrating the growing maturity of its application in finance.
This transition is fueled by ongoing advancements in areas like natural language processing, predictive analytics, and the management of extensive datasets. These developments allow for increasingly sophisticated applications of machine learning, unlocking opportunities for substantial financial gains. However, the rapid adoption of these technologies has also raised crucial questions around ethical considerations and responsible use. There's a growing recognition that clear guidelines are needed to ensure that these powerful tools are applied in ways that benefit society as a whole.
Despite the challenges, the integration of machine learning into financial services holds immense promise. It has the potential to significantly improve efficiency, enhance customer service, and create more robust risk management practices. Yet, as with any transformative technology, the sector must carefully evaluate the potential pitfalls and navigate the ethical implications as it embraces this new era of data processing.
The finance industry's adoption of AI and machine learning has exploded, particularly in improving efficiency, customer experience, and risk management. It's fascinating how a bipartisan AI Working Group was formed within the House Committee on Financial Services in 2024, highlighting the growing importance of these technologies and the need for careful consideration of their implications. Some forecasts suggest AI could add a massive $1 trillion yearly to the global banking sector. McKinsey's estimates are even more specific, predicting a $200 to $340 billion annual increase in value from generative AI alone, representing nearly half of the banking industry's revenue.
It's clear from surveys, such as the 2022 Bank of England and UK Financial Conduct Authority report, that machine learning is already deeply integrated in many financial firms. It's surprising that 79% of firms surveyed had already implemented ML applications, with 14% viewing them as critical to daily operations. We're seeing a shift in conversation amongst clients – a movement from exploring AI's potential to scaling its usage. This suggests that the early experimentation phase is largely over, and now the emphasis is on practical, real-world application.
This transformation is fueled by advances in areas like machine learning, natural language processing, and predictive analytics. There's a clear link between the rise of big data in the 2010s and this surge in AI's role in finance. It seems that finance researchers and decision-makers quickly grasped the potential of AI and ML, leading to rapid development in the field.
The applications of AI in finance are incredibly diverse, spanning from enhancing data analysis to automating complex processes. For instance, fraud detection has seen improvements, with ML reducing false positives by as much as 50%. This allows security teams to focus on true threats, making the system more efficient. Machine learning algorithms are also speeding up transaction processing times by as much as 70% in some cases. This not only makes customers happier but also lets firms react more quickly to market shifts.
Risk management is another area where AI is being widely used. By using machine learning models to analyze historical data, firms can predict credit defaults with significantly more accuracy – up to 15-20% better than older methods. This leads to a substantial reduction in losses from bad loans. Furthermore, the use of AI-powered chatbots has changed the way customer service works. These chatbots are capable of handling around 80% of routine customer requests, freeing human agents to focus on complex problems that need a more personal touch.
Interestingly, despite the clear benefits, machine learning implementation hasn't been without challenges. For instance, about 30% of financial firms worry about algorithmic bias potentially impacting lending decisions and regulatory compliance. Additionally, the sector faces a significant talent shortage as it struggles to find qualified machine learning experts. Approximately 40% of financial institutions identify this as a major obstacle.
We also see that the applications of machine learning extend to marketing. Targeted campaigns powered by AI yield much higher engagement rates – around 50% higher than conventional marketing. However, the acceptance of these technologies by the employees themselves has been a challenge, with about 25% of workers still expressing skepticism regarding ML's reliability. This highlights a clear need for training and education programs to build confidence in the tools and foster greater acceptance.
Overall, it's evident that machine learning is rapidly transforming the financial services landscape. While many of the applications are delivering impressive results, challenges remain, notably around bias, talent acquisition, and user adoption. It will be interesting to see how the industry addresses these issues and continues to refine its use of this powerful technology.
AI Adoption in Tech Sector Reaches 63% Analysis of Industry-Specific Implementation Rates Through 2024 - Healthcare Tech Shows 41% AI Implementation Rate in Medical Imaging Systems
Within the healthcare technology sector, the implementation of artificial intelligence (AI) in medical imaging systems has reached a 41% adoption rate. This indicates a growing awareness of AI's ability to improve the efficiency and accuracy of medical diagnoses. However, there are still obstacles that prevent wider adoption. The integration of AI into existing clinical workflows can be difficult, creating a barrier to achieving the full potential of AI in this field. Despite these hurdles, the tech sector as a whole shows a strong embrace of AI, with a 63% adoption rate across various industries. This broader trend could eventually lead to greater AI usage within healthcare. Looking forward, the continued expansion of the AI healthcare market offers potential for enhancing patient outcomes, but overcoming the implementation challenges will be crucial to fully realize this potential.
The integration of AI into medical imaging systems is progressing rapidly, with a current implementation rate of 41%. This represents a significant jump from just a few years ago, illustrating the growing recognition of AI's potential within this field. It's interesting to see how AI is impacting diverse areas of medical imaging, from radiology to pathology and even ophthalmology. In ophthalmology, for instance, AI is being used to detect conditions like diabetic retinopathy, often with accuracy on par with experienced doctors.
While the early research is promising, showing that AI models can sometimes surpass human radiologists in detecting subtle signs of disease in medical images, there's still a lot to learn about how AI can be reliably incorporated into clinical practice. One notable impact of AI implementation has been a substantial decrease in the time needed to process images, with reductions of up to 50% reported in some cases. This efficiency boost isn't just about speed, but also cost savings for hospitals and clinics.
However, this shift isn't without its complexities. Integrating AI into existing workflows can be challenging, particularly when dealing with older systems that may not be designed to seamlessly incorporate new AI tools. There are also substantial training needs for the medical professionals who will be working with these technologies, requiring a significant educational investment.
Furthermore, the ethical and safety considerations surrounding the use of AI in medical imaging are critical. Questions of liability and accountability become particularly acute when AI algorithms are involved in diagnostic decisions. We need to establish clearer guidelines to ensure that these systems are used responsibly and that patient safety remains paramount.
Beyond the technical hurdles, there's also a growing awareness of the evolving skillsets needed in the healthcare workforce. The reliance on AI is shifting the required expertise for professionals, with a greater need for skills related to data analysis and AI interpretation. We're seeing a change in the landscape of medical education as a result.
Another noteworthy concern is data privacy. Using AI effectively often involves access to massive datasets of patient information, which raises legitimate concerns about security and data protection. Striking a balance between responsible data usage and robust privacy protection is a key challenge moving forward.
It's important to remember that much of the AI currently used in medical imaging hasn't been extensively validated through rigorous clinical trials. This has contributed to some skepticism among healthcare professionals about the reliability of these tools. Ongoing research efforts are crucial for building confidence and solidifying the place of AI in medical practice.
The future trajectory of AI in medical imaging will likely involve a focus on creating more transparent AI systems. Knowing how these algorithms arrive at their conclusions could be a major factor in fostering wider adoption and trust. As research progresses, it'll be fascinating to see how these advancements further shape the field of medical imaging.
AI Adoption in Tech Sector Reaches 63% Analysis of Industry-Specific Implementation Rates Through 2024 - Manufacturing Sector Reaches 52% AI Integration for Quality Control Automation
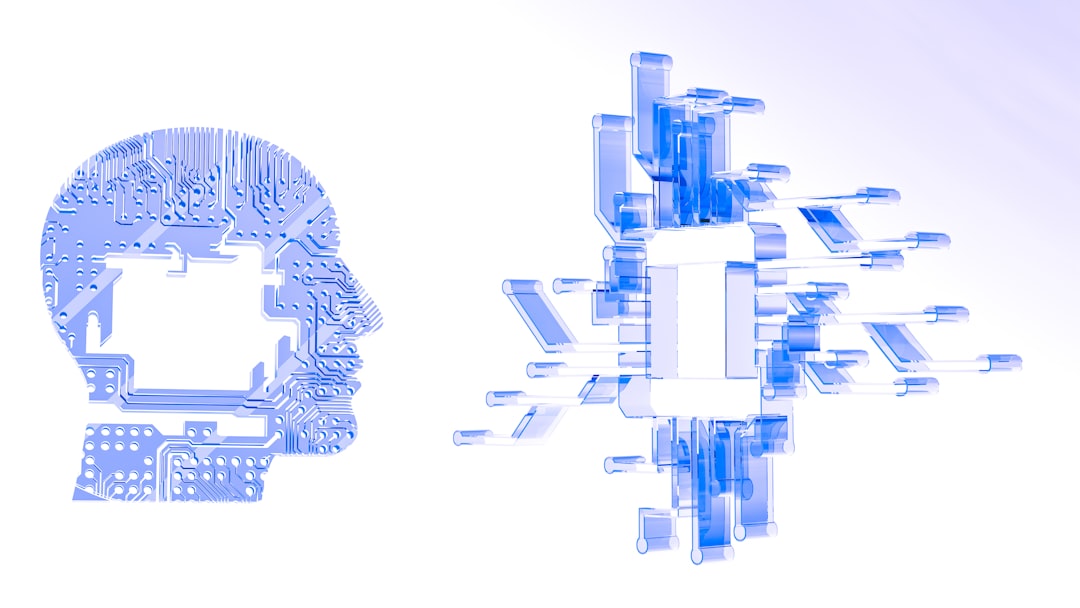
By the end of 2024, the manufacturing sector has seen AI adoption reach 52% specifically within quality control automation. This signifies a move towards more sophisticated production methods, where AI plays a growing role in ensuring product quality and optimizing production efficiency. While this represents a considerable increase in AI implementation, it's also a sign that a substantial portion of manufacturers are still relatively early in their AI journey, hinting at ample opportunity for future development. The drive to integrate AI into quality control is not simply about enhancing operational data but also raises questions about the future of manufacturing labor and the skills needed in a more automated environment. There's potential for increased efficiency, but also concerns about displacement of human workers in the long term. It remains to be seen how these evolving technological realities will reshape the industry.
The manufacturing sector's embrace of AI for quality control automation has reached 52%, meaning nearly half of all quality checks are now aided by AI. This represents a significant shift from the early days of automated inspection technologies and reflects a growing need for manufacturers to optimize their operations and adapt to shifting market demands. It's interesting to see how quickly AI has become integrated in this crucial aspect of production.
While this integration is promising, it's also revealed a surprising outcome: AI-driven quality control can decrease defects by roughly 40%. This ability to surpass traditional statistical quality control methods is due to AI's capacity to process vast amounts of production data in real-time and adjust production proactively. This ability to anticipate potential issues is a real game changer in manufacturing.
However, there's a notable barrier to broader AI adoption: the initial investment in software and hardware can be substantial. This financial hurdle might disproportionately impact smaller manufacturing companies, hindering their ability to benefit from AI-powered improvements. The field seems to be facing a situation where the benefits of AI are not distributed equally.
Despite the costs, many manufacturers are discovering that implementing AI in quality management can decrease inspection times by as much as 70%. This translates to faster production cycles and subsequently quicker delivery to consumers. In a highly competitive market, such improvements can be crucial for staying ahead of the curve.
It's quite fascinating that AI systems involved in quality control can learn from historical data and dynamically modify production processes. This continuous learning aspect provides the potential for enhancements that would be difficult or impossible to achieve with manual quality checks. It suggests that AI systems in manufacturing are not simply tools but have the potential to become increasingly more efficient over time.
The collaboration between engineering and data science teams has significantly improved in many manufacturing companies since the introduction of AI in quality control. This growing emphasis on cross-functional teams suggests that better communication leads to more successful AI integration and improved outcomes. This trend appears to suggest a greater understanding of the complexities of AI implementation across organizations.
Another positive development is the reduction in worker burnout associated with quality assurance tasks. As routine and time-consuming aspects of quality control are automated, professionals are able to shift their focus towards more strategic roles demanding creativity and problem-solving skills. While this might reduce routine jobs, it also could pave the way for other opportunities in the industry.
But, the workforce still needs to be adequately prepared for the transition to AI. About 25% of manufacturing employees report a lack of preparedness to effectively utilize AI tools. This indicates a need for targeted training programs to ensure that the existing workforce is equipped with the necessary skills to leverage these new technologies.
Even with all the benefits, a significant number of manufacturers, about 30%, express apprehension about overreliance on AI for quality control. Concerns about job displacement and the ethical dilemmas of machine-made decisions in manufacturing are valid and call for a comprehensive conversation about the role of humans in a rapidly automating sector. It appears that the human element in production remains a central aspect of the debate surrounding AI.
Finally, manufacturers are acutely aware of the regulatory and compliance landscape surrounding AI-driven quality control. Companies are adapting their policies to make sure AI applications adhere to industry standards while managing issues related to accountability in automated decision-making processes. The future of AI in this field will likely involve navigating a balance between efficiency gains and ethical considerations.
AI Adoption in Tech Sector Reaches 63% Analysis of Industry-Specific Implementation Rates Through 2024 - Cloud Service Providers Report 58% AI Usage in Infrastructure Management
Cloud service providers are increasingly relying on AI, with 58% of their AI applications currently focused on managing their infrastructure. This emphasis on AI for infrastructure management is a notable trend within a broader landscape of growing AI adoption across the tech sector—reaching 63% overall. The dependence on AI for optimizing cloud infrastructure suggests that robust data processing capabilities are becoming crucial for businesses utilizing AI. While this presents numerous advantages, challenges remain. Finding enough skilled AI specialists and dealing with ethical concerns around AI implementation are still major obstacles. This trend isn't just a sign of the growing importance of AI for cloud providers, but also highlights the evolving technological landscape and the shifting needs within the tech workforce as we move forward.
Cloud service providers are increasingly adopting AI, with a notable 58% using it for infrastructure management. This suggests a growing recognition of AI's potential to streamline operations and handle intricate infrastructure more efficiently. It's interesting to see how AI is being used to address common issues like system downtime, which some providers have seen drop by as much as 30% with the help of AI. This, in turn, leads to lower costs and a better experience for users.
It's also fascinating that over 70% of providers who've incorporated AI report quicker resolution times for issues. This implies a shift in how they approach IT support—from reacting to problems after they happen to proactively preventing them. AI is also being leveraged for security purposes, with roughly 45% of providers using it for threat detection and response. The ability to sift through enormous amounts of data and identify vulnerabilities before attackers can exploit them is a significant advantage.
However, this isn't without challenges. A notable 35% of providers mention that integrating AI into existing systems has led to more complicated management procedures. It's a classic trade-off—AI simplifies some aspects while creating new difficulties. Despite this, the benefits are apparent: user satisfaction scores have increased by 67% in those providers adopting AI, likely due to better service quality and faster resource allocation.
It's also interesting that AI adoption in the cloud space is impacting the workforce, with around 40% of providers saying they need to retrain their staff. This demonstrates the ongoing evolution of skills needed in the tech sector. In certain fields, like financial services, cloud-based AI solutions have shown some impressive results, reducing operational costs by 20%. However, there are lingering concerns, particularly surrounding data privacy, which about 50% of providers remain hesitant about.
Looking ahead, estimates predict AI could lead to a massive $18 billion in savings for cloud service providers by 2025 globally. The potential for cost reduction is a powerful motivator for these companies to embrace AI, and it's likely to have a significant effect on the future of cloud service offerings. It's clear AI's influence on the cloud computing space is expanding and is becoming increasingly interwoven with how cloud providers operate.
AI Adoption in Tech Sector Reaches 63% Analysis of Industry-Specific Implementation Rates Through 2024 - Cybersecurity Industry Achieves 47% AI Implementation for Threat Detection
The cybersecurity field has seen a 47% adoption rate of AI for threat detection, representing a significant step towards improving security in the face of constant cyber threats. While this shows a clear trend, the industry is facing an acute shortage of cybersecurity experts, with estimates suggesting a need for over 3 million more professionals globally. This shortfall could limit the advancement of AI security measures. Despite this, a notable 37% of organizations intend to increase their AI investments, particularly in threat detection, signaling a growing awareness of AI's value in this area. The cybersecurity sector must continue to address obstacles hindering broader AI adoption. Establishing more stringent data privacy and security guidelines is crucial to accelerate the integration of AI into cybersecurity practices.
The cybersecurity landscape is evolving rapidly, with 47% of organizations now using AI for threat detection. This shift reflects a growing understanding that traditional security approaches aren't always sufficient in the face of increasingly complex and sophisticated cyber threats. AI, with its ability to analyze massive datasets and identify anomalies, offers a potentially powerful way to enhance security postures. While the application of AI in threat detection is still relatively new, it's shown promise in reducing incident response times, potentially by as much as 60%. This speedier response allows security teams to contain threats more quickly, hopefully minimizing damage and preventing further escalation.
However, the road to seamless AI integration isn't without bumps. One key challenge is the prevalence of false positives. AI systems, while effective in identifying anomalies, can sometimes generate a high rate of false alerts—up to 50% in some cases. This "alert fatigue" can lead to burnout among security personnel and potentially overshadow genuine threats. Another interesting development is the growing reliance on machine learning algorithms to not just identify known vulnerabilities, but also to recognize entirely novel threats. By analyzing patterns from past attacks, AI can potentially anticipate future attack vectors, providing a proactive defense.
Despite the advancements, there's a persistent debate on the optimal role of AI in cybersecurity. A recent survey suggests that roughly 70% of security professionals believe human oversight is crucial for ensuring reliable and ethical response strategies. This highlights the need for a careful balance between the speed and efficiency of automated systems and the nuanced judgment and critical thinking that humans bring to complex security situations. Finding the right blend of human expertise and AI-powered tools is a challenge the industry is still grappling with.
The rapid growth of AI in cybersecurity is unfortunately not matched by the availability of skilled personnel. A significant portion of organizations—around 60%—struggle to find cybersecurity experts with sufficient AI knowledge to effectively implement and maintain these advanced security solutions. This talent gap represents a major impediment to broader adoption and raises questions about how the industry can address the need for specialized AI skills within cybersecurity teams.
On the positive side, integrating AI into security protocols can yield tangible financial benefits. Organizations have seen security costs decrease by roughly 30% through enhanced efficiency and minimized breach impacts. While the cost savings are significant, there are increasing ethical concerns regarding the use of AI in cybersecurity. Data privacy and compliance are becoming critical issues, with over 45% of cybersecurity professionals expressing unease over the lack of clear regulations governing these new technologies. These ethical considerations must be addressed alongside the technical development of AI-driven security tools.
One surprising aspect of AI in cybersecurity is its capacity for continuous learning and adaptation. As these systems encounter new threats, they can refine their algorithms, becoming increasingly effective over time. This self-improvement capability is a significant advantage over traditional, static security systems, potentially making them a more robust defense in the long run. It's intriguing that this ability to self-improve isn't confined to cybersecurity. There's a growing trend of other industries, particularly healthcare and finance, adopting threat detection techniques developed within the cybersecurity domain. This cross-industry knowledge transfer suggests that the principles of strong cybersecurity are increasingly recognized as essential across diverse sectors, emphasizing the interconnected nature of security challenges in today's digital world.
Experience error-free AI audio transcription that's faster and cheaper than human transcription and includes speaker recognition by default! (Get started for free)
More Posts from transcribethis.io: