Experience error-free AI audio transcription that's faster and cheaper than human transcription and includes speaker recognition by default! (Get started for free)
7 Ways to Identify a Song When You Can Only Remember the Melody
7 Ways to Identify a Song When You Can Only Remember the Melody - Record and Upload Your Humming to AudioTag Database
AudioTag offers a direct way to identify songs based on your humming or a recorded melody. You simply record a short audio clip—the first 60 seconds, to be exact—and upload it to their database. This database is built to compare your recording to its stored music library and hopefully give you a match. Though seemingly easy, it's limited to a 100MB file size for the uploaded audio. This approach is particularly handy if you're struggling with recalling the song's name or lyrics but have a solid grasp of the tune. However, the accuracy of the search hinges on how clear and unique the recorded audio is, and it's not guaranteed to yield a definitive answer each time. While this technique provides a relatively simple approach to song identification, its success is contingent upon the quality of the humming or recording.
One approach to identifying a song when you only recall its tune involves uploading a recording of your humming to a database like AudioTag. This method leverages the advancements in audio fingerprinting technology, where unique characteristics of a song are extracted and stored. While it offers a promising way to pinpoint a song, users need to be aware of its limitations.
AudioTag, for instance, permits recordings up to 100MB, focusing on the first minute of your hum. However, the quality of the recording significantly impacts the identification process. Even slight background noise can interfere, and given the inherent variability in how humans reproduce melodies, accurately capturing pitch is not always straightforward. Studies suggest a significant portion of the population struggles to accurately reproduce a song's pitch when humming, which can create challenges for the system.
The system itself learns through the sheer volume and diversity of uploaded recordings. The more varied the humming samples, the better the algorithms can recognize patterns. However, relying solely on user-generated data can introduce inconsistencies in quality and consistency, which, in turn, can affect the accuracy of the identification process. While impressive in its ability to identify songs based on extracted melodies, the dependence on machine learning makes it a continuous work-in-progress, continually adapting and refining its capabilities. It is important to keep in mind that its effectiveness is related to the breadth and depth of its database. Those systems trained on a broad range of genres and styles typically have a higher rate of success compared to systems trained on a narrower musical focus. It is still important to recognize that the intricacies of human expression, specifically the way a person hums a tune, are often difficult to map to the machine learning models and that occasionally, automated systems miss the mark due to subtle differences in phrasing and timing.
7 Ways to Identify a Song When You Can Only Remember the Melody - Tap the Beat Pattern Into Musipedia Virtual Piano
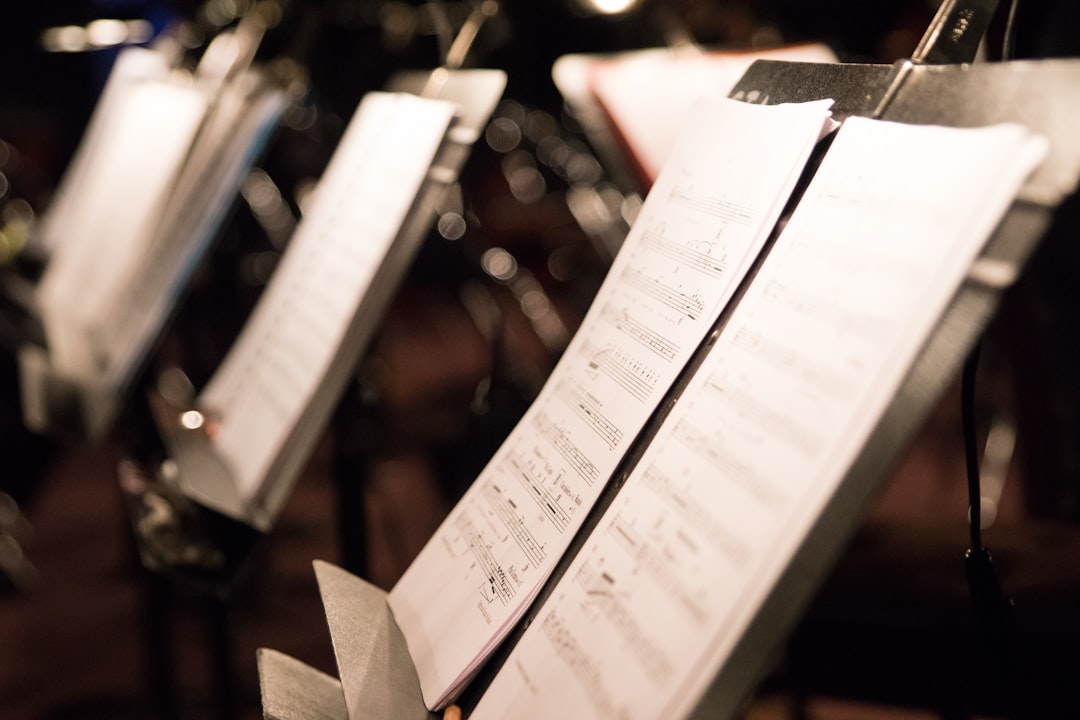
Musipedia offers a different approach to song identification by focusing on the rhythmic structure of a song rather than the melody itself. You can tap the beat of the song into their virtual piano using your keyboard. This approach can be quite helpful if you're struggling to recall the actual notes of a tune but have a strong sense of its rhythm. The availability of a virtual piano on the Musipedia site further expands the options for inputting melodies, potentially improving your chances of finding the song you're looking for. The underlying concept of Musipedia is based on a collaborative model, much like Wikipedia, where everyone can contribute to the ever-growing database of musical pieces. This open approach, while potentially leading to inconsistent data, allows for a continuously developing resource that evolves and expands over time with each contribution. The accuracy of song identification through this method is largely dependent upon the accuracy of your rhythm input and the quality of the stored music data. It is not an exact science, but a potentially useful alternative for individuals who struggle with pitch and rely more on the beat.
Musipedia presents an intriguing approach to song identification, particularly when you only recall a melody. It relies on a blend of music theory and machine learning to recognize melodic patterns. Users can tap out a rhythm on a virtual piano, essentially 'teaching' the system the tune. This interaction helps Musipedia learn and adapt its algorithms, improving the recognition process over time. The ability to input melodies via keyboard taps or mouse clicks makes it accessible to a wider range of users, even those who lack formal piano training.
However, this method is sensitive to how users input the melody. The system attempts to differentiate between staccato and legato playing styles, showcasing a level of detail in its approach. But research has shown that people aren't always accurate in replicating pitch or rhythm when tapping out a tune. This suggests that Musipedia's algorithms need to be quite robust to compensate for human variability.
Musipedia's design also incorporates a community element, allowing users to share their experiences and techniques for tapping melodies. This interactive aspect can lead to more efficient song identification over time. Furthermore, the system tackles the challenge of 'melodic density,' where complex melodies with many notes are input. Algorithms appear to be designed to prioritize significant musical phrases within the melody, which helps with recognition.
Musipedia's online accessibility ensures broad usability across various devices, and the continuous improvement of its algorithms, fueled by user feedback and interaction patterns, makes it a constantly evolving tool for musical discovery. While its success relies on users providing a reasonable facsimile of a song’s melody, Musipedia highlights the potential of tapping and machine learning to help with musical recall. It is also an interesting example of a system that leverages collaborative improvement, where the user-base helps shape the technology itself, making it more capable over time. The accuracy and efficiency of such a system depends on the large-scale, continuous input of users to train and refine the systems. It remains to be seen just how successful it is in terms of practical use, but it certainly presents an interesting approach to dealing with the rather common problem of remembering a song and not being able to find it.
7 Ways to Identify a Song When You Can Only Remember the Melody - Use Google Voice Search While Humming the Tune
If you only recall a song's melody, Google's voice search offers a helpful way to identify it. Through a feature called "Hum to Search," accessible within the Google app or search widget, you can simply hum, whistle, or sing the tune for about 10 to 15 seconds. Google's system then uses sophisticated audio processing and machine learning to analyze the melody and compare it to its vast music database. It presents potential matches, ranked by how likely each song is to correspond to your humming.
This approach is quite convenient since it utilizes a widely available tool. However, the results you get depend on factors such as the clarity of your humming and how accurately you can reproduce the melody. Background noise or inaccuracies in the humming can sometimes affect the system's ability to pinpoint the correct song. In essence, it's a convenient and widely-accessible method but relies on your ability to produce a clear and recognizable rendition of the melody. While Google has invested heavily in this type of technology, it's important to remember that it's a work in progress and the results aren't always perfect. Nonetheless, it stands as a creative approach to rediscovering songs that have eluded your memory.
Using Google's voice search to identify a song by humming is an interesting approach, particularly when you can't recall lyrics or the actual notes of a song. It seems like it should be fairly straightforward. You open the Google app or use the Google Search widget, tap the microphone, and hum the tune for about 10 to 15 seconds. Google then tries to match the hummed melody to its extensive music database using a sophisticated machine learning algorithm.
However, this process highlights a number of interesting challenges in translating human musical expression into a machine-readable format. It seems people generally find it easier to recall melodies when humming than when singing, likely because it's less cognitively demanding. But the accuracy of pitch when humming varies widely, and even small variations in pitch can throw off the recognition algorithms. Google's system, like other systems built on artificial intelligence and machine learning, is trained on vast amounts of data, yet this data likely doesn't capture the range of subtle variations and improvisational aspects inherent in human humming.
It's fascinating to consider that our ears can distinguish between about 20,000 different sound patterns. Capturing those subtle nuances when humming is crucial, but most systems are designed to handle a more limited range of variations. Moreover, external noise can have a significant impact on the process, making it imperative to create a quiet environment for recording.
Another interesting aspect of these systems is that they often utilize a form of community learning. As more people use them, the algorithms learn how people tend to hum different melodies, updating and refining the accuracy of the database. But it's also important to acknowledge that the technology can't fully capture the emotional expression or personal interpretations that we associate with humming. And the way people hum can vary a great deal based on their musical background and cultural context. All these factors, including background noise, user engagement, and the diversity of musical expression across cultures, affect the accuracy and reliability of the system.
Ultimately, the effectiveness of Google's Hum to Search functionality, or any song identification system based on humming, relies on a feedback loop. If users get good results, they're more likely to continue using it, providing more data to train the system. But if they encounter inaccurate matches often, they might lose interest. This illustrates the continuous challenge of balancing machine learning with the inherently complex nature of human musical interaction. While it's a powerful technology, we must remain mindful of its limitations and acknowledge that it's not a perfect solution for remembering every song that gets stuck in our heads.
7 Ways to Identify a Song When You Can Only Remember the Melody - Play Notes on Music Notes Recognition App
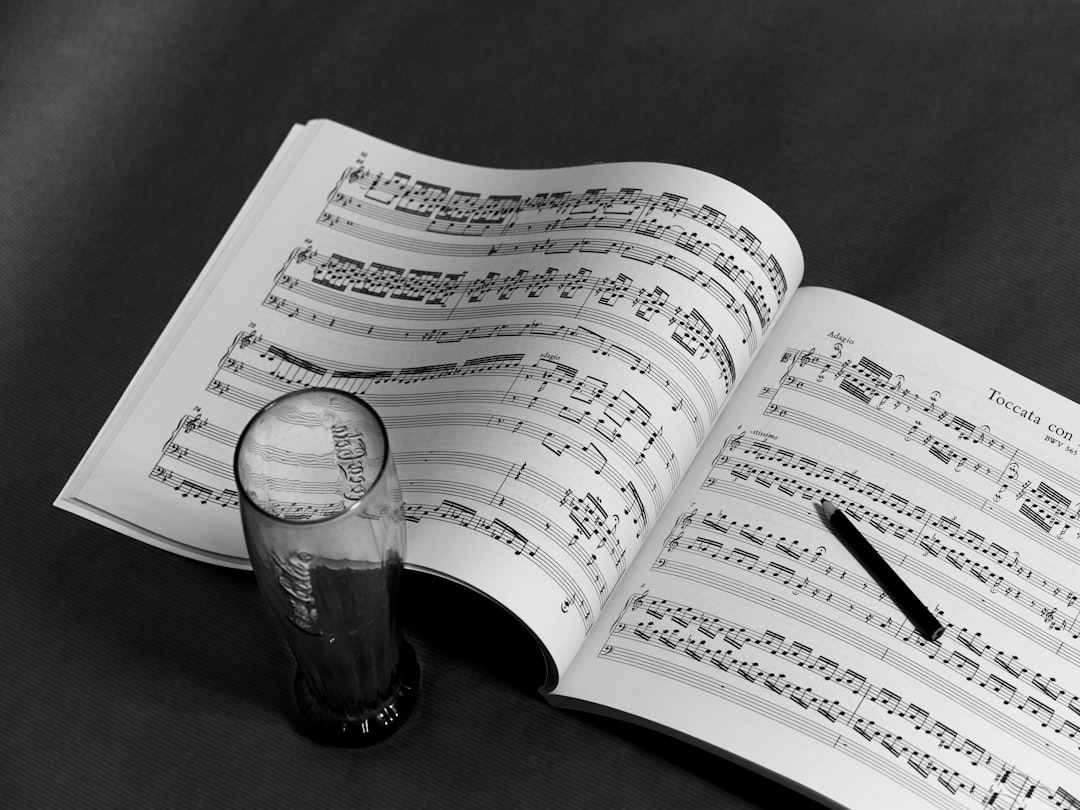
"Play Notes on Music Notes Recognition" apps present a different approach to identifying songs by allowing users to input melodies directly. These apps typically use virtual instruments like keyboards or other input methods to translate played notes into musical notation in real time. This approach can be helpful for learning music theory and recognizing tunes, but it's not without limitations. The accuracy of these apps depends on the precision of the user's input and the complexity of the algorithms powering the software. While they can be beneficial for refining musical skills and learning about note recognition, they sometimes struggle to capture the subtle aspects that make melodies unique. For example, variations in timing or the emotional nuances a musician expresses can be difficult to translate into a digital format. These apps show promise as aids in identifying songs but serve as a reminder that capturing the nuances of human musical expression in a digital format is a complex undertaking.
Music recognition apps that allow you to "play notes" use a fascinating combination of music theory and computer science. They rely on methods like pitch detection and how fast or slow a melody is played to try and interpret a hummed or played tune.
However, studies show that it's often tricky for people to recreate a melody perfectly. This means that variations in how high or low a note is and the rhythm can make it harder for these recognition systems to work well.
Many apps use machine learning. This means they are trained on a huge collection of music to find patterns and relationships between melodies that might not be obvious to our ears. This is really interesting because it allows computers to 'learn' to identify songs.
What's also interesting is that these systems usually improve when people give them feedback. This means they get better at recognizing tunes over time because they can adjust based on whether they're right or wrong.
The way a musical note is written or played matters for recognition. Things like how high or low it is and how long it lasts create unique sound patterns that help algorithms tell the difference between similar-sounding tunes.
Some research indicates that we usually recognize tunes that are familiar to our culture, but music from different parts of the world might be harder for these computer programs to understand. They haven't been trained on enough of this music.
It's crucial that these apps are trained on recordings with many different instruments and singing voices. This is because the sounds created by various things affect how accurately the system can identify a melody.
Background sounds are a big problem for these apps. Even a little bit of noise can mess up the recording and lead to the wrong song being chosen. This is a challenge for improving their performance.
Research in how we think suggests that our feelings and emotions are important for remembering a tune. Maybe including information about emotions in these apps would make them better at identifying songs.
Music recognition technology is changing very quickly. We now have more powerful computers and smarter algorithms, allowing us to process complex musical information in real-time. This was impossible before and opens up new possibilities for how we interact with music and find the tunes we're looking for.
7 Ways to Identify a Song When You Can Only Remember the Melody - Search Online Sheet Music Archives With Basic Melody
If you can only remember a song's melody, searching through online sheet music archives can be a surprisingly effective way to identify it. Several online resources allow you to search using just a basic melody. Musipedia, for example, acts as a sort of online encyclopedia of music, where users can input melodies by humming, whistling, or playing them on a virtual keyboard. Each entry can contain sheet music, MIDI files, and other information about the piece. It is built on a collaborative model, meaning the content is user-edited, similar to Wikipedia. The accuracy of Musipedia's searches can be uneven since it relies on users for data input, making it a resource with potential but not necessarily a foolproof solution. Another well-known option is Musescore, which boasts a massive collection of over a million freely available sheet music scores. It provides a large search space for those who want to search for music based on a melody. The ability to browse sheet music collections online by composer, title, or keywords expands the ways a user can look for a melody that might be stored there. However, these approaches depend on the quality of the input and the nature of the archived melodies. While useful tools, they highlight the inherent difficulty in representing human musical expression, with its unique timing and nuances, within a digital format. While searching with a basic melody can lead to a successful identification, the accuracy of the results relies heavily on how clearly you can recall the melody and how well those melodies are represented within the archive itself.
Online music archives offer a fascinating avenue for identifying songs based on just the melody. However, the process is not always straightforward, and there's a surprising amount of complexity beneath the surface. Here are ten insights that might shed some light on how these systems work:
1. People don't all hum the same way. Research suggests that a significant portion of the population struggles to accurately reproduce a melody when humming, likely due to variations in pitch memory. This variation adds a layer of complexity to the task of recognizing melodies through automated systems.
2. While machine learning has made leaps and bounds, it still has limitations when it comes to recognizing melodies. These algorithms learn from vast amounts of musical data but might stumble when presented with unusual or complex tunes outside of their usual training set. This can result in unexpected errors in identification.
3. We can typically filter out background noise when we hear a melody, but algorithms aren't quite as adept at that. Background noise can severely hinder a system's ability to isolate and accurately analyze the frequency characteristics of a hummed melody. This is a significant challenge for making these systems more robust.
4. The complexity of a melody matters a lot to how easy it is for a system to recognize it. Melodies that are packed with a large number of notes played quickly can create challenges for algorithms as they need to distinguish overlapping sounds effectively.
5. There's a tendency for music recognition systems to be better at recognizing music from certain cultures. Systems typically perform better with music they've been trained on extensively, leading to potential bias against genres or styles that are less common in the data.
6. We often remember songs better if they have emotional significance. The emotional connection we have with a song seems to enhance our ability to recall the melody. This suggests that the way we remember melodies might be deeply intertwined with our emotions, and potentially could inform the way these systems are designed.
7. These systems are often continuously learning based on user feedback. If you use a system to identify a song and it's right, the algorithms get reinforced. If the system is incorrect, the algorithm 'learns' from the mistake, which helps it make fewer errors over time. It's a continuous feedback loop that refines the process of identification.
8. The size and diversity of a database are a key element in determining success. Systems with large, varied libraries of music tend to have higher success rates compared to systems trained on a narrower selection of genres or eras. More data allows for a wider range of melodic patterns to be recognized.
9. Recent advancements in processing power have enabled real-time music processing. This has allowed for a much more seamless experience when interacting with these systems. It is a remarkable technological step forward that was not possible even a few years ago.
10. Variations in musical expression can significantly alter how a melody is perceived. Even slight changes in tempo or subtle articulations can introduce differences that lead to misidentification by a system looking for an exact match. It's a reminder of how expressive and nuanced human musical interpretation can be.
These insights suggest that while using a melody to search for a song online can be a helpful approach, the process is far more sophisticated than it might initially appear. The underlying technology and its limitations are continually evolving, but it's a fascinating field of study in the realm of computer science and music cognition.
7 Ways to Identify a Song When You Can Only Remember the Melody - Write Down Musical Notes Using Online Music Staff
When you want to write down a musical idea, online music staff tools can be a valuable resource, especially if you're trying to capture a song you only remember the melody of. Platforms like Noteflight or MuseScore offer a user-friendly way to create and edit music notation. This can help you better understand the underlying structure of the melody. There are also online platforms where you can collaborate and share your musical compositions with others, like Flat. This aspect can foster learning and improve the transcription process, as you can receive feedback from others.
However, there are some challenges involved. Translating the often complex and nuanced way humans express music into digital notation is still difficult. The quality of the written music can depend on the person's ability to enter the information accurately. The ability of these platforms to capture subtleties in music, such as the emotional quality of a piece, is also a continuing area of development. But despite the limitations, they provide an interesting way to bridge the gap between musical ideas in our minds and their written form. These online platforms highlight the challenges inherent in transferring human creativity into digital representations, showcasing both the potential and the limitations of digital music notation in capturing the full richness of music.
Several online tools allow you to write down musical notes using a virtual staff. These tools range from full-featured music notation software like Noteflight or MuseScore, which are widely used by composers and educators, to simpler platforms like Typatone, which focuses on transforming typed input into musical notes. Other services, like Flat, emphasize collaboration and sharing, allowing musicians to connect and exchange musical ideas. There are also tools, like ScoreCloud Studio, which can analyze audio or MIDI input to create a score. Additionally, some platforms are more game-like, using interactive exercises to help students learn musical notation on the staff.
It's interesting to note that these platforms have fostered a new kind of collaboration within the music community. By creating a shared space for music creation, users can exchange knowledge and musical ideas. While this collective effort can broaden musical understanding, it also reveals some limitations of online tools. For example, while some of these programs offer real-time feedback, helping users learn music notation, the accuracy of capturing a tune ultimately depends on a person's ability to recall the melody accurately and then translate it into notation.
The challenge is that our memory for melodies is not always perfectly translated into a digital format. It appears to be easier to remember a tune than to correctly reproduce the notes on a musical staff. This highlights a potential bottleneck in musical notation, as individuals may struggle to capture complex or nuanced melodies as accurately as they remember them. The cognitive demands of recalling and writing down a melody can make it a challenging task for many people.
Thankfully, the algorithms used in these applications have improved with time and greater processing power. However, they still don't perfectly capture subtle timing differences or stylistic variations in musical phrasing, especially when dealing with variations in instruments, tempos, and the expressive qualities of individual musicians.
The way a user enters notes, whether it is through a virtual keyboard, MIDI interface, or a simple mouse click, also impacts the overall process. External elements, like background noise, can interfere, making it difficult for the algorithm to pick out the intended melody. It's also intriguing to see how emotion and memory are linked. Research shows that our memories for melodies that have emotional significance tend to be stronger.
Furthermore, some platforms use machine learning, continuously improving as more users interact with them. This means that over time, these online staff tools are likely to become even more accurate in their representation of music. However, it is important to acknowledge that the accuracy of music recognition can depend on the cultural background of the music itself, making some tools better suited for certain styles or genres over others.
While it seems like a straightforward task, transcribing music from memory using online music staff tools unveils several unexpected challenges regarding human perception of musical patterns, limitations of software, and the challenges of capturing a nuanced and expressive art form in a structured and digital format. Even with the ever-improving technology and increased collaboration, the field of music notation in the digital age remains a dynamic and fascinating area of research.
7 Ways to Identify a Song When You Can Only Remember the Melody - Ask Music Communities on Reddit Music Forums
**Ask Music Communities on Reddit Music Forums**
Reddit's music-focused communities can be a valuable resource when you're trying to identify a song you only remember the melody of. Subreddits specifically dedicated to helping people find songs, like "r/NameThatSong" and "r/WhatSong", are built for this purpose. You can share what little you remember—maybe a few lyrics, a link to a recording, or even try humming the tune. The idea is that people from all musical backgrounds come together and share their knowledge, improving the odds of someone recognizing the song.
The effectiveness of this approach is uncertain though. It depends on how well you describe the song and whether there are enough active users at the time who are willing to help. While this informal approach can be a quick way to find a song, the quality and consistency of responses can vary. Sometimes people misidentify songs or miss the right answer entirely. Despite the occasional limitations, using these music communities is a novel way to find songs that are stuck in your head.
Reddit's music communities offer a unique approach to identifying songs based solely on a melody. These online spaces essentially function as a massive, constantly evolving database of musical knowledge, with a wide range of users contributing their expertise and insights. The rapid exchange of information within these communities can be incredibly helpful when trying to recall a song you only remember the tune of, often leading to quicker results than traditional methods.
However, the effectiveness of these communities depends on a few factors. The diverse backgrounds and musical tastes of the members contribute to a wide variety of perspectives on melodies, which can be both beneficial and problematic. While it expands the potential for discovering unusual or less common songs, the interpretations and suggestions may vary significantly depending on the cultural context of the community. This suggests that the success of a particular query may depend on the specific community you tap into.
Additionally, relying solely on user input introduces a level of subjectivity into the process. While these communities can overcome some limitations of automated music recognition systems, user engagement plays a significant role in their accuracy and usefulness. For instance, if users aren't actively involved in the discussion and sharing their musical knowledge, the communities become less helpful.
Further complicating matters is a tendency for users to favor songs and musical styles from their own cultural background, leading to a potential 'echo chamber' effect. This can limit the range of suggestions when a person is trying to identify a tune that falls outside those shared musical experiences. Nonetheless, the anonymity of these platforms can encourage users to share obscure or personal song memories, which can lead to the discovery of rare or lesser-known tunes not typically found using standard search methods.
The way users describe a melody can also vary, adding complexity to the process. While some users might employ musical terminology to describe the melody, others might rely on pop culture references or descriptive imagery, which can be both insightful and lead to misinterpretations.
It's also interesting that these communities often contribute a level of historical context that goes beyond the melody itself. Users might reference the song's era, its connections to other musical genres, or its cultural impact. This contextual information can deepen the identification process and provide a more comprehensive understanding of the song's significance.
In summary, Reddit music communities present a valuable alternative to automated systems for identifying songs based on melody, but their success is tied to active community participation and the inherently subjective nature of musical interpretation. They can reveal hidden treasures of music, but also highlight the complexities of translating a subjective experience, like remembering a tune, into a format that can be successfully shared and understood within a digital community. While not a perfect solution, their ability to leverage the diverse knowledge and experiences of music enthusiasts makes them a compelling tool for rediscovering long-forgotten melodies.
Experience error-free AI audio transcription that's faster and cheaper than human transcription and includes speaker recognition by default! (Get started for free)
More Posts from transcribethis.io: