Experience error-free AI audio transcription that's faster and cheaper than human transcription and includes speaker recognition by default! (Get started for free)
7 Speech-to-Text Apps That Excel at Transcribing Song Lyrics in 2024
7 Speech-to-Text Apps That Excel at Transcribing Song Lyrics in 2024 - Descript AI Engine Captures Complex Rhyme Patterns From Rap Songs
Descript's AI engine has shown promise in handling the challenges of rap music transcription, particularly its ability to recognize intricate rhyme schemes. It appears to go beyond simply capturing words, and is designed to understand the complex interplay of rhyme and rhythm that defines much of the genre. This is a noteworthy achievement in speech-to-text, as many AI systems struggle with the rapid delivery and intricate wordplay common in rap. The accuracy of the transcription becomes more critical as the desire for precise lyric capture within musical contexts grows. Descript's AI, along with others, could be a step towards more refined and usable speech-to-text tools for musicians and music fans alike. It's still early days in this area, but these tools could help advance understanding of music beyond just what we hear, and open up new possibilities in how lyrics are studied, analyzed, and even used creatively in future projects.
Descript's AI engine has a knack for dissecting the sounds of words in rap, going beyond basic transcription to uncover the intricate ways rappers weave rhymes. It leverages machine learning to study how rhyme patterns occur across different rap styles, revealing patterns and how styles have evolved. This isn't just about simple rhyme identification; it can distinguish between different types of rhymes – like internal, end, or near rhymes – by focusing on how syllables are stressed, which is key for understanding the rhythm and flow in rap.
The engine can also grasp the way artists manipulate the beat and delivery, helping researchers analyze how this impacts lyric writing and performance. Interestingly, it incorporates elements of cultural understanding by picking up on regional differences in rap language, providing insight into how location affects lyrical expression. The Descript engine relies on a vast archive of transcribed rap songs, allowing it to refine its capabilities by learning from a wide range of lyrical styles from diverse artists.
Descript also provides visual displays of rhyme density and lyrical flow, giving a powerful tool for music production. By allowing the comparison of lyrics and their underlying patterns, it offers a resource for aspiring songwriters to dissect the craft of rap. Descript differentiates itself from basic transcription software by incorporating the emotional tone behind the lyrics, offering avenues for exploring listener reactions and engagement with the music.
As this technology continues to mature, it's conceivable that it could find a place in diverse fields, such as music therapy and linguistic research, furthering our understanding of how humans use language creatively. It is a technology that continues to develop and could be applied in numerous fields outside of music creation.
7 Speech-to-Text Apps That Excel at Transcribing Song Lyrics in 2024 - Dragon Professional Adds Music Recognition Mode Plus Beat Detection
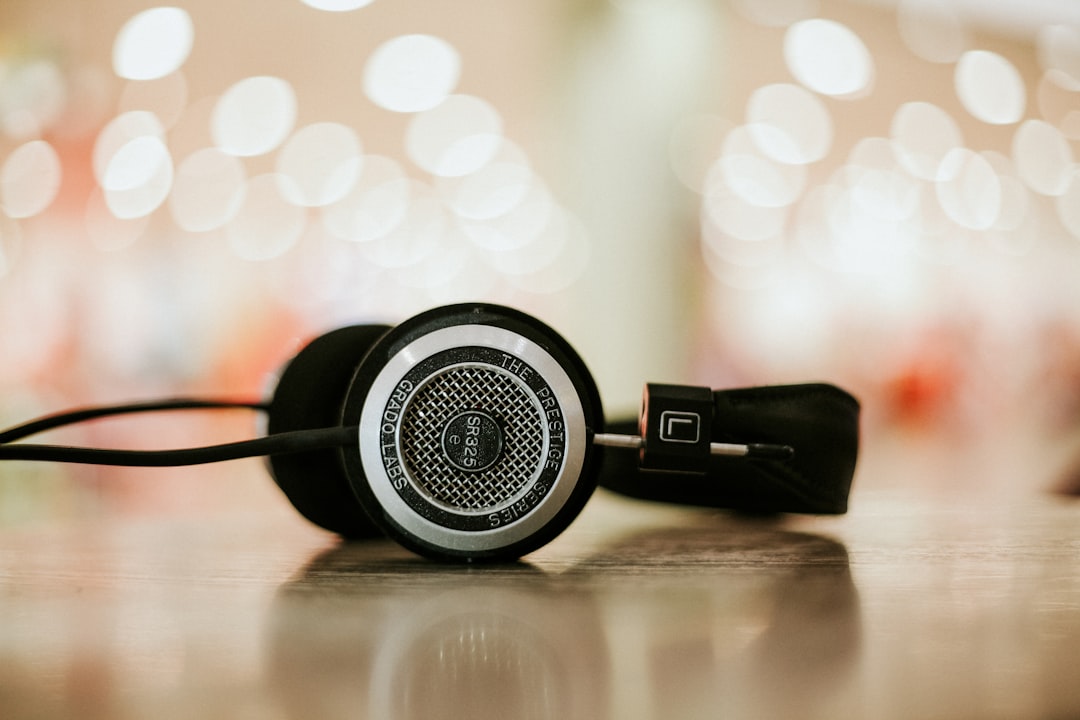
Dragon Professional's latest version, v16, includes a new "Music Recognition Mode" along with "Beat Detection." This update is aimed at improving its ability to handle music-related transcription tasks, particularly capturing song lyrics. The goal is to enhance accuracy by better recognizing the rhythm and phrasing common in music. This is in addition to Dragon's existing features, like adapting to individual voices and letting users create custom commands for a personalized experience. Whether it surpasses other programs that are already specifically focused on music transcription remains to be seen. While promoted as a productivity enhancer, judging its practical effectiveness in the realm of musical transcription still requires testing. Regardless, it's a step towards better speech-to-text software that can be helpful for musicians and those who work with lyrics.
Dragon Professional's latest version, v16, has introduced a Music Recognition Mode along with Beat Detection, aiming to improve its capabilities for transcribing musical content, particularly song lyrics. This addition signifies a focused effort to refine speech-to-text for the nuances of music. It’s fascinating to see how they've incorporated algorithms to try and pinpoint specific musical elements and patterns, leading to a higher degree of accuracy in song transcriptions.
Beyond just recognizing rhythm, the beat detection component dives into analyzing tempo changes and variations within musical tracks, which can be particularly helpful for genres with complex rhythmic structures. This granular understanding of the rhythmic relationship between the music and the lyrics can elevate the quality of the transcription process.
Dragon v16 is trained on a wide array of musical genres and styles, hoping to ensure it adapts to various musical structures, instrumentation, and lyrical styles. This adaptability is important in a field as diverse as music. It also uses frequency analysis to separate the vocal elements from the accompanying instrumental tracks, aiming for cleaner transcriptions of lyrics by filtering out background noise. This is particularly beneficial when transcribing live music performances where sound quality might not be consistent.
The Music Recognition Mode has also adopted a feedback system where users can correct transcriptions in real-time. This dynamic interaction allows users to continually refine transcriptions while also contributing to the overall improvement of the software. It's an example of how the software can learn and improve over time. It's even designed to detect and understand different vocal techniques like vibrato and staccato, hoping to capture the nuances in lyrical delivery that give a song emotional depth.
Dragon has taken things a step further by implementing a feature to suggest lyrical alterations based on the rhythmic and melodic patterns. It’s an innovative addition that potentially assists songwriters seeking to create or adapt lyrical content. This version is also geared toward handling musical pieces with fluctuating tempos, a common feature in many contemporary music styles. This adaptive capability underscores the engineering that's gone into this upgrade.
Furthermore, it incorporates a phonetic recognition system designed specifically for musical contexts. This should help address slang, colloquialisms, and even specific language unique to different musical genres—aspects that often stump traditional speech-to-text software. The coupling of rhythm analysis with lyrical transcription opens up the possibility of broader applications within academic contexts like studying songwriting trends or tracing the evolution of genres through lyric analysis. It could be a valuable asset in musicology research. Whether or not it will become a tool used frequently by researchers remains to be seen. It's just another fascinating piece in the puzzle of ever-improving speech-to-text technology.
7 Speech-to-Text Apps That Excel at Transcribing Song Lyrics in 2024 - Google Cloud Speech API Translates Multiple Singer Vocals Simultaneously
Google Cloud's Speech API offers a unique capability: it can differentiate between multiple singers in a song and transcribe their individual parts. This is done through a feature called speaker diarization, which tags each word with the speaker who uttered it. This is quite useful for accurately capturing lyrics in songs with multiple vocalists or harmonies. The API works best with an audio sampling rate of 16,000 Hz, leading to high-quality transcriptions. Furthermore, this API allows for real-time audio streaming and is designed to handle multiple voices, making it a compelling option for transcribing musical performances. While the technology isn't perfect and is still under development, its abilities in handling multiple speakers suggest it could be a valuable tool for music transcription and analysis.
Google's Cloud Speech API utilizes sophisticated machine learning, particularly deep neural networks, to tackle the challenge of transcribing multiple singers at once, even within complex musical arrangements. This capability stems from a process called speaker diarization, which tags each word in the transcription with the corresponding speaker, allowing for the separation and transcription of individual vocal tracks.
Unlike some other speech recognition tools, Google Cloud Speech API distinguishes overlapping vocals using a technique called voice activity detection. This method effectively isolates active speech segments from background noise and other sounds, enabling a more accurate transcription. The API also incorporates a language model that's adaptable to musical contexts, helping to capture specific genre-related nuances and slang that might trip up more conventional transcription systems. This adaptivity is quite impressive.
The Google Cloud Speech API boasts support for over 120 languages and dialects, making it an exceptional tool for transcribing a wide array of musical styles and genres from various cultures and regions. Its flexible approach handles a diverse range of linguistic features common in musical performances. The technological foundation leverages both phonetic recognition and acoustic modeling to improve accuracy, particularly in situations where voices have similar styles or pitch ranges.
It's worth noting that the API's cloud-based design facilitates the efficient processing of large audio datasets. This is especially valuable for transcription tasks that involve live music with multiple vocalists, a challenging environment for speech-to-text systems. Furthermore, Google continues to refine the Speech API through ongoing updates that incorporate user feedback and data from real-world scenarios. These improvements are key to improving the API's ability to recognize both well-known and lesser-known artists' voices.
It appears Google Cloud Speech API goes beyond simply transcribing words. The technology delves into analyzing the rhythm and melody within the audio and incorporates this musical information into its algorithms. This approach allows the system to understand the intricate interplay between lyrics and musical elements in a way that previous generations of speech recognition models often struggled with. Google Cloud Speech API utilizes voice clustering techniques to organize overlapping voices based on attributes like pitch and tone. This aspect further improves the transcription process, especially in vocal arrangements with multiple, interwoven tracks.
As artificial intelligence in music transcription progresses, Google Cloud Speech API serves as a good example of how technology is moving beyond just capturing the music. The API strives to understand and interpret musical components, creating possibilities for innovative applications in areas like music research and composition. It will be interesting to see how these types of tools are applied in the future.
7 Speech-to-Text Apps That Excel at Transcribing Song Lyrics in 2024 - MacWhisper Uses Neural Networks to Separate Vocals From Instruments
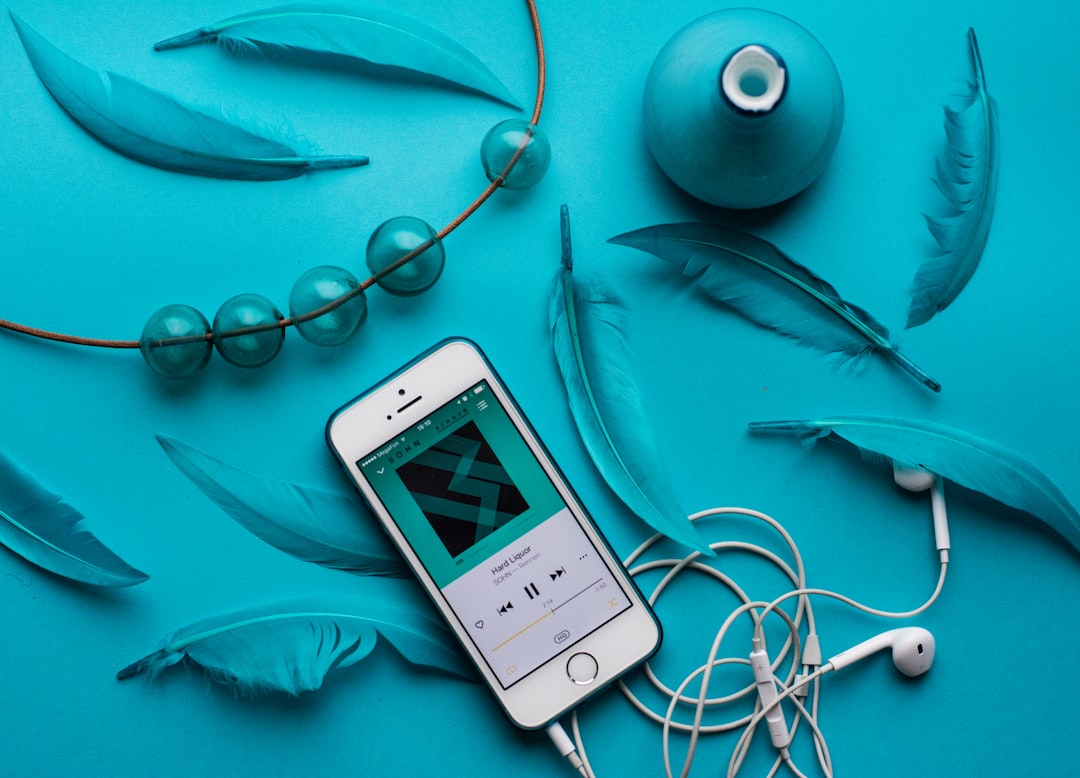
MacWhisper is an interesting tool that uses neural networks to separate vocals from the music in a sound file. This is done using OpenAI's Whisper technology. It's useful for music transcription, as isolating the vocal track can be tricky. The app makes it easy to upload files and is fast, capable of working with many languages and audio types. One thing that sets it apart is that it works locally on your computer, without needing an internet connection. This makes it appealing for people focused on music, such as songwriters and musicians. However, how well it works with complex musical pieces, where there might be lots of instruments or multiple singers, is an area to consider if precise lyric capture is the goal.
MacWhisper employs sophisticated neural networks, specifically tailored for audio source separation, which allows it to effectively distinguish vocal tracks from intricate instrumental arrangements in musical recordings. It combines spectrogram analysis with deep learning algorithms, helping it understand the distribution of frequencies and time-based audio signals, leading to more accurate vocal isolation. Intriguingly, MacWhisper draws on concepts from both music information retrieval and signal processing, allowing it to identify vocal characteristics and patterns that set them apart from overlapping instrumentals.
Rather than using basic filtering techniques, MacWhisper utilizes advanced convolutional neural networks (CNNs) trained on extensive audio datasets. This approach significantly enhances its ability to pick up on subtle audio characteristics. During the training phase, MacWhisper is exposed to a wide range of audio examples. This not only teaches it to separate sounds, but it also learns to recognize variations in vocal dynamics, pitch, and modulation, resulting in refined and nuanced vocal extractions.
However, MacWhisper's performance in separating vocals can differ based on the musical genre. Songs with a strong vocal presence or clearly distinguishable instrumental timbres usually produce better results compared to complex, layered arrangements where audio separation becomes much more difficult. Interestingly, it includes real-time processing capabilities, which allows for live music separation and transcription. This makes it potentially useful for both on-stage performance situations and post-production audio tasks.
The software's capacity to accommodate varying audio qualities, whether from concert settings, studio recordings, or even recordings from portable devices, underscores its adaptability across different audio environments. MacWhisper gives users control over the refinement of the isolation process, offering manual adjustments based on the specific characteristics of the audio. This feature can be particularly beneficial for producers who need more customized audio outputs.
While a lot of tools focus on transcription accuracy, MacWhisper emphasizes the quality of vocal extraction. This dedication to preserving the subtle emotional nuances of vocal performance adds an interesting dimension to lyrical analysis. This is a noteworthy approach, as preserving these elements adds a richness that might otherwise be lost using other techniques.
7 Speech-to-Text Apps That Excel at Transcribing Song Lyrics in 2024 - Otter Launches Musicians Studio With Advanced Chord Recognition
Otter has introduced a new feature called Musicians Studio, which focuses on helping musicians by incorporating advanced chord recognition. This feature combines speech-to-text with an understanding of musical structure, allowing users to transcribe lyrics and musical ideas more efficiently. Essentially, Otter is trying to improve the transcription process for those who create music by adding artificial intelligence capabilities. While potentially useful for songwriters who need to capture their ideas, it remains to be seen how well the chord recognition works in practice for a wide variety of musical styles. This new addition to Otter's suite of features highlights the ever-evolving world of transcription tools and how they are impacting the way musicians record and examine their work. Whether it proves to be a truly useful tool for all musicians will require testing and feedback over time.
Otter has introduced a new feature called Musicians Studio, which incorporates advanced chord recognition. This addition essentially allows musicians to use their speech-to-text capabilities to transcribe lyrics and musical ideas, simultaneously capturing chords in real-time. It's an intriguing development in the broader landscape of speech-to-text applications specifically designed for music creation. Otter's AI-driven Meeting Assistant, previously known for transcribing meetings and generating summaries, now seems to be getting more sophisticated.
The Musicians Studio uses machine learning to continuously improve its chord recognition. It's able to identify chord structures across a range of instruments, which is useful as many musicians don't solely rely on guitars or keyboards. Interestingly, the software gives users immediate feedback during musical creation, helping them hear how their chord progressions and lyrics sound together. This immediate feedback should help foster a faster workflow.
While the ability to recognize basic chords isn't unheard of, Otter's system is specifically designed to handle complex chord structures—including alterations and inversions. This could be useful for musicians working in genres with complex harmonic structures. It also boasts collaborative capabilities, allowing musicians to work together remotely. This aspect highlights a growing trend in creative processes, as more and more musicians work across geographical boundaries.
Otter has attempted to include some music theory instruction as well, potentially helpful for musicians interested in deepening their understanding of music theory as they work. This could become more effective over time as the system refines its capabilities. One particular element they are leveraging is MIDI data, enabling it to recognize chords played through virtual instruments, especially useful for electronic music producers who work extensively with synthesizers. Finally, the Studio allows the export of chords and lyrics in common formats, catering to musicians needing a consistent, industry-standard method of sharing or publishing their compositions.
It's a bit early to declare whether this is a significant advancement for music production. It's yet to be seen how widely adopted and effectively it will be in the studio. However, it demonstrates a move toward more musically aware AI tools that offer the possibility of making transcriptions more useful to musicians. If the system continues to develop, it could help reduce manual labor during the creative process, potentially speeding up songwriting and leading to new musical discoveries.
7 Speech-to-Text Apps That Excel at Transcribing Song Lyrics in 2024 - Microsoft Azure Cognitive Service Detects Song Structure and Tempo Changes
Microsoft Azure's Cognitive Services has recently introduced capabilities within its Speech service that can identify song structure and tempo shifts. This means that in addition to converting audio to text, it can now analyze the musical framework of a song, providing more accurate and contextually rich transcriptions. Developers can leverage the Azure AI Speech SDK, which supports various programming languages, to build apps that benefit from this real-time analysis. The potential is there for useful tools for musicians and audio producers, but it's unclear how well these features can capture the subtleties of different music genres in practical use. The need for more precise lyric capturing is increasing, and tools like this one from Azure could have a significant impact on how songwriters and researchers deal with music transcription. It remains to be seen how widely it will be adopted in different applications and if it will meet the expectations of music professionals.
Microsoft's Azure Cognitive Service offers a unique approach to music analysis, going beyond simply transcribing lyrics. It delves into the structure of a song, identifying sections like verses and choruses, a feature potentially helpful to researchers and composers. The ability to handle real-time audio makes it suitable for use in live settings, potentially offering instant feedback and transcription during performances or recording sessions. This capability relies on a significant amount of machine learning, as the model is trained on an extensive collection of musical audio, allowing it to adapt to a variety of styles from pop to classical.
Interestingly, it can not only pinpoint tempo changes but also subtle variations like accelerando and ritardando that musicians often use to add expressiveness. It also tries to account for how music is created across cultures globally, suggesting a possible application to musical styles beyond what's considered typical in Western music. The service seamlessly integrates with other software, providing flexibility for music producers. It also benefits from user input, encouraging feedback to improve its transcription accuracy. This creates a system that learns and adapts to real-world use over time.
Azure also offers input flexibility, handling everything from high-quality studio recordings to live audio streams, catering to a wide range of users and recording environments. In addition to the core functionality of identifying musical elements, it uses advanced methods to try and isolate vocals from the instrumental accompaniment, an important feature when transcription accuracy of lyrics in dense musical pieces is the goal.
These capabilities hint at exciting possibilities for the future. As technology develops, tools like Azure's Cognitive Service could eventually be used in novel applications like automated music generation, where a deep understanding of structure and tempo could help in creating music within a specified style. It's intriguing to see how this tech is applied in the coming years.
7 Speech-to-Text Apps That Excel at Transcribing Song Lyrics in 2024 - Rev Creates New Music Library With 50,000 Pre-Trained Song Templates
Rev has introduced a substantial music library containing 50,000 pre-trained song templates, aiming to make music creation more accessible. It's a move that suggests a growing trend of AI assistance in the songwriting process, offering a foundation for users to build upon. This is paired with Rev's existing transcription services, known for their 99% accuracy and rapid turnaround times. While pre-trained templates can simplify starting a song, the question remains whether these templates are versatile enough for a broad range of musical genres and individual styles. The future of music technology may hinge on finding a balance between readily available tools and safeguarding the unique artistic expression of individual musicians.
Rev has introduced a substantial music library containing 50,000 pre-trained song templates, a significant resource for music creation. This extensive collection, potentially spanning numerous genres, could provide a substantial boost to the songwriting process. It's intriguing that these templates are pre-trained on a wide range of musical structures. They seemingly come equipped with pre-defined chord progressions, lyrical themes, and even melodic patterns. By providing a somewhat structured starting point, these templates might help to reduce the usual trial-and-error phase many musicians encounter during the early stages of composition.
The approach Rev took is rooted in a data-centric approach. They've apparently analyzed trends in current music to guide the template development. Whether this leads to music that's more commercially viable is yet to be determined. This strategy suggests that it isn't just intuition or random creativity that's at play here, but some attempt at leveraging what is statistically considered more popular.
Underlying this project are machine learning algorithms. They've likely used AI to learn from the patterns found in successful songs, in an effort to extrapolate common elements in musical composition that lead to popular songs. One can only speculate how far-reaching this method could be, but the potential for a different way to build music is significant.
It's important to consider that these templates don't constrain artists; users can certainly adjust the lyrics provided within each template. It's not like the templates dictate a singular composition, but rather a foundation that can be altered to suit individual expression. Having an initial structure in place could be useful, but there is still a demand for originality in musical creation, and this seems to be accounted for in the overall design.
Rev has established a feedback loop into the design, making adjustments over time based on how the templates are used. This signifies that the system should get better with continued use. It’s hard to predict if the feedback will lead to templates that are more relevant or representative of future trends in popular music, but it does signal an adaptable system.
Musicians have the capability to blend elements from many different genres in their projects, making this library quite flexible in terms of genre. This is interesting because it indicates that the creators intended to have a diverse set of templates to appeal to a wider range of music makers. The potential for producing fusion-style music, or simply incorporating stylistic influences from various musical cultures, could be a notable benefit.
Behind the scenes, the platform analyzes users’ listening habits to identify the templates that garner the most engagement. Knowing this might enable the creators to tailor future template offerings, possibly leading to a more tailored experience for the community of music creators that will use this library. The developers appear to be attempting to create a symbiotic relationship between user experience and template optimization, where the templates themselves are influenced by user behaviour.
Real-time adjustments are part of the platform. This allows artists to make immediate changes while hearing the musical output. This capacity for rapid, dynamic changes makes the compositional process more fluid, and allows users to quickly integrate any creative ideas that might arise spontaneously.
The templates offer not just a way to create new songs, but could be considered an educational resource as well. It could serve as an informative tool, illustrating various songwriting techniques and musical theories embedded within the templates. This implies that it could be a tool for those wanting to further refine their knowledge of music creation, in addition to its main function of accelerating creative production. The long-term impact of Rev's template library on the music landscape remains unknown, but it holds the promise of transforming how music is created and learned.
Experience error-free AI audio transcription that's faster and cheaper than human transcription and includes speaker recognition by default! (Get started for free)
More Posts from transcribethis.io: