7 Proven Methods for Transcribing Long-Form Podcast Episodes with 99% Accuracy
7 Proven Methods for Transcribing Long-Form Podcast Episodes with 99% Accuracy - Manual Audio Preprocessing Using Audacity For Crystal Clear Sound Quality
Manual audio preprocessing within Audacity offers a powerful way to refine podcast audio for better transcription results. A key element is noise reduction. Audacity's Noise Profile and Noise Remover tools effectively target and remove unwanted background sounds, leading to a cleaner audio signal. Furthermore, adjusting the overall volume, along with the meticulous use of the equalizer to tailor vocal frequencies, can greatly improve the clarity of the recording. Proper setup is also important. Checking microphone levels and setting the right sample rate in Audacity's preferences are crucial for initial recording quality. Mastering these aspects not only elevates the perceived audio quality, but also ensures the foundation is laid for a more accurate transcription process. While Audacity offers many tools, understanding their purposes and proper application, such as splitting audio clips or navigating the toolbars, is key to effectively manipulating audio files and achieving higher-quality results. These steps, while time consuming, can contribute to achieving more polished audio output that greatly benefits the clarity and accuracy of automatic transcription tools.
When working with Audacity to improve audio quality, a helpful tool is the "Spectrogram View." This visual representation of sound frequencies reveals noise patterns that can be targeted for removal, making audio much cleaner.
Understanding the Nyquist frequency is essential. Essentially, to capture a sound accurately, the sampling rate must be at least double the sound's highest frequency. Adjusting the sample rate in Audacity, following this principle, is important for accurate audio reproduction.
Audacity's noise reduction tools can be quite effective, capable of significantly lowering background noise levels, potentially by 90% with skillful application. This capability aids in isolating vocal tracks and boosts overall sound quality.
Audacity's "Compressor" feature provides control over the dynamic range of audio files. It helps create a more uniform sound by raising quieter audio portions and lowering louder peaks, smoothing the audio experience for listeners.
While Ctrl+Z is the standard undo, Audacity goes beyond this basic functionality. Its extensive edit history allows for reversing to a specific, non-sequential past edit. This can be a timesaver, especially during complex projects with multiple edits.
Audacity allows for batch processing, enabling simultaneous editing of numerous files using the same effects or adjustments. This can drastically accelerate the workflow for podcasters managing a substantial number of episodes.
Audacity also allows users to add a variety of plugins, like VST and LADSPA, increasing its functionality. These plugins provide avenues to customize sound and tailor audio enhancements for achieving the desired outcomes in professional-level audio productions.
Gaining precise control over audio levels within Audacity is achievable through a feature that normalizes audio tracks to a specific amplitude. This helps to maintain consistent volume across multiple podcast episodes, avoiding sudden volume shifts that can be distracting for listeners.
Manual audio adjustments, particularly equalization, play a significant role in improving audio quality. By adjusting frequencies, such as boosting midrange frequencies, one can enhance the clarity of vocal recordings, which directly benefits the intelligibility of speech.
Audacity's "Label Track" offers an interesting opportunity for transcribers. It gives the ability to add timestamps and notes to the audio, creating a kind of annotated timeline. This can simplify the transcription process, as specific portions of a lengthy recording can be more easily accessed.
7 Proven Methods for Transcribing Long-Form Podcast Episodes with 99% Accuracy - Building Custom Language Models Through AI Training Sets
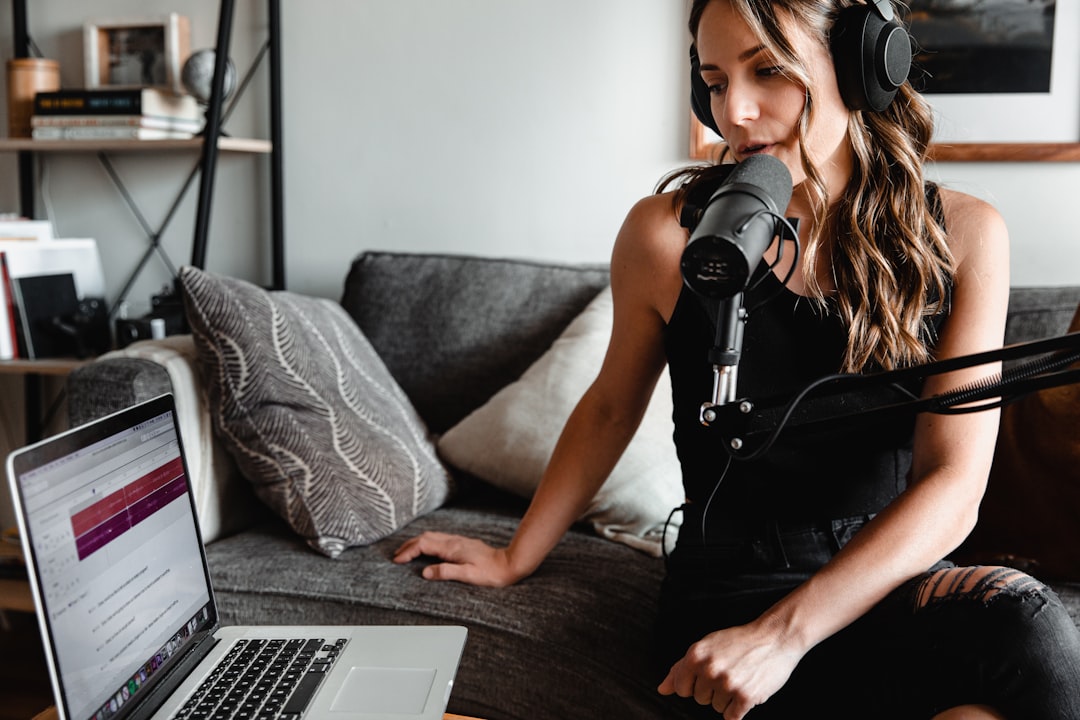
Creating custom language models using AI training sets is a powerful method to enhance the accuracy of speech-to-text, particularly in specialized areas. These models can be fine-tuned to understand the unique vocabulary and language patterns of a particular niche, surpassing the limitations of generic AI transcription engines. The foundation of these models lies in meticulously crafted datasets that provide the training data needed for optimal performance. Interestingly, techniques are emerging to efficiently "distill" knowledge from larger, more complex models into smaller, faster ones. This allows for the benefits of sophisticated language processing to be applied in more resource-constrained environments while maintaining a high degree of accuracy. These custom AI models hold immense potential for improving transcription accuracy, especially for lengthy podcast episodes, where precise capture of intricate conversations is crucial. With continued advancement in large language model capabilities, leveraging customized approaches is likely to become increasingly important in the quest for achieving near-perfect transcriptions. While there are challenges, the ability to build models specifically for podcasts with technical jargon or distinct speaking styles could lead to a new level of precision.
Custom language models, even when trained on relatively small datasets—perhaps as few as a thousand high-quality transcripts—can develop a remarkable ability to grasp the nuances of specialized terminology and context within specific fields. This leads to a substantial boost in the accuracy of transcriptions within that domain.
Leveraging established language models through transfer learning allows developers to significantly reduce both the time and computational power needed for creating specialized models. By essentially "fine-tuning" a pre-existing model on smaller, custom datasets, the development process becomes more efficient.
The quality and relevance of the training data is of paramount importance. Using training sets that richly reflect the language used in a particular industry can yield much better understanding compared to models trained on generic language data. In some cases, it can boost performance by over 30%.
A technique known as "domain adaptation" focuses the language model on the distinctive language patterns found within a given domain, like legal proceedings or medical discussions. This allows for a more targeted approach, effectively tailoring the language model to the specific linguistic quirks of a niche field.
Introducing audio disturbances during the training process, known as noise-aware training, makes language models more robust to real-world conditions. Effectively, this allows them to learn how to filter out various background sounds that are commonly encountered during recordings, leading to cleaner transcripts.
Integrating prosodic features, such as tone and emphasis, into the training data can significantly enhance a model's ability to capture the subtleties of spoken language. These features are often overlooked by simpler transcription methods.
Intriguing research suggests that extending the context window, or the amount of preceding text considered during processing, during training helps the model grasp the broader flow and coherence of sentences. This can improve the resulting transcriptions, making them more accurate in representing the speaker's intended meaning, especially in longer conversations.
There is promising progress in multimodal training. The concept involves combining audio with visual cues to give the model a richer understanding of context. This is particularly beneficial in situations where visual elements provide further insight into the spoken words. However, these approaches require significantly more complex training setups.
Active learning offers an adaptive approach to language model training. Users' feedback in real-time can be used to refine the model's performance, helping it to constantly adjust to new industry trends or emerging terminology.
Careful tuning of training hyperparameters, which are essentially the knobs and levers that govern the training process, can have a huge impact on a model's performance. By meticulously adjusting these settings, developers can fine-tune the model's behavior for specific acoustic settings or speech styles. This process requires significant experimentation and a deep understanding of machine learning algorithms.
7 Proven Methods for Transcribing Long-Form Podcast Episodes with 99% Accuracy - Synchronized Multi Track Recording With Timestamps
Synchronized multi-track recording, where each speaker or audio element is captured on its own track, coupled with precise timestamps embedded within the recording, provides a significant advantage in achieving high-accuracy podcast transcriptions. This approach allows for improved audio clarity, as individual audio sources can be separated and analyzed more effectively, simplifying the task for both human and AI-powered transcription. The timestamps act like a roadmap for the transcription process, enabling easy access to specific moments in the conversation, particularly helpful for lengthy episodes. While these features make the workflow more efficient, modern speech recognition technologies also play a crucial role by converting audio to text with impressive accuracy. It's important to note that the best outcomes often require a blend of technological tools and human oversight. While AI can process audio with remarkable speed and precision, careful review and attention to detail can further elevate the accuracy of the final transcription.
Synchronized multi-track recording, with its ability to capture audio from multiple sources simultaneously and precisely, offers a powerful approach for enhancing transcription accuracy. This technique, where each audio stream is recorded with timestamps down to the microsecond, enables highly accurate audio alignment and synchronization, proving especially helpful during mixing and editing.
By utilizing individual microphone placements for each track, engineers gain access to a richer, more nuanced set of sound properties. This offers greater control and flexibility when adjusting audio levels during post-production, especially when dealing with complex audio environments with multiple speakers. Dynamic range compression can be applied independently to each track, effectively smoothing out the audio across the entire mix. It is crucial to use such techniques with care; over-compression can reduce the dynamic range too much, making the recording sound lifeless.
The layered nature of synchronized multi-track recording grants remarkable flexibility. If a particular section of one track contains unwanted noise or interference, it can be isolated and refined without impacting the other tracks. This ability to "surgical-edit" within a mix allows for targeted fixes without disrupting the entire recording, an important quality for maintaining a high quality recording for the task of transcription.
Furthermore, synchronized multi-track recording enables collaboration across distances. For instance, multiple speakers can participate in an interview while located in different studios, with their individual audio streams seamlessly integrated into a single project timeline. This approach could greatly improve the flow of complex recording projects and provide greater access to remote professionals.
Modern digital audio workstations offer visually intuitive interfaces that leverage timestamps. These tools help engineers to readily manage and manipulate tracks, simplifying the process of aligning and adjusting audio that may have become misaligned due to variations in hardware. However, the volume of audio data generated by multi-track recordings can be massive. Engineers need to employ robust storage and management strategies to avoid logistical nightmares. This also has implications in terms of bandwidth, as sharing and transmitting large projects can be costly and time consuming.
Synchronized recording has particular benefits in live environments, such as capturing a concert or theatrical performance. By capturing each instrument or vocal part individually, engineers can fine-tune the mix in post-production, allowing for adjustments to deal with any unpredictable background noise or stage feedback that often occurs in live settings. Timestamping is not just useful for syncing the recording; it's also instrumental in the editing process. Transcribers can jump quickly to a specific point in the recording rather than tediously scrubbing through hours of audio. This kind of fast navigation speeds up the overall workflow, which is invaluable in dealing with long podcast episodes.
Historically, multi-track recording was limited due to hardware and software constraints. Thanks to advancements in digital audio technologies, creating high-quality multi-channel recordings has become more readily available. While still not without its challenges, multi-track recordings represent a powerful technique that has dramatically shifted paradigms in audio production and is rapidly becoming an essential tool for those wanting to maintain and process large scale audio projects.
7 Proven Methods for Transcribing Long-Form Podcast Episodes with 99% Accuracy - Live Error Detection Using Natural Language Processing
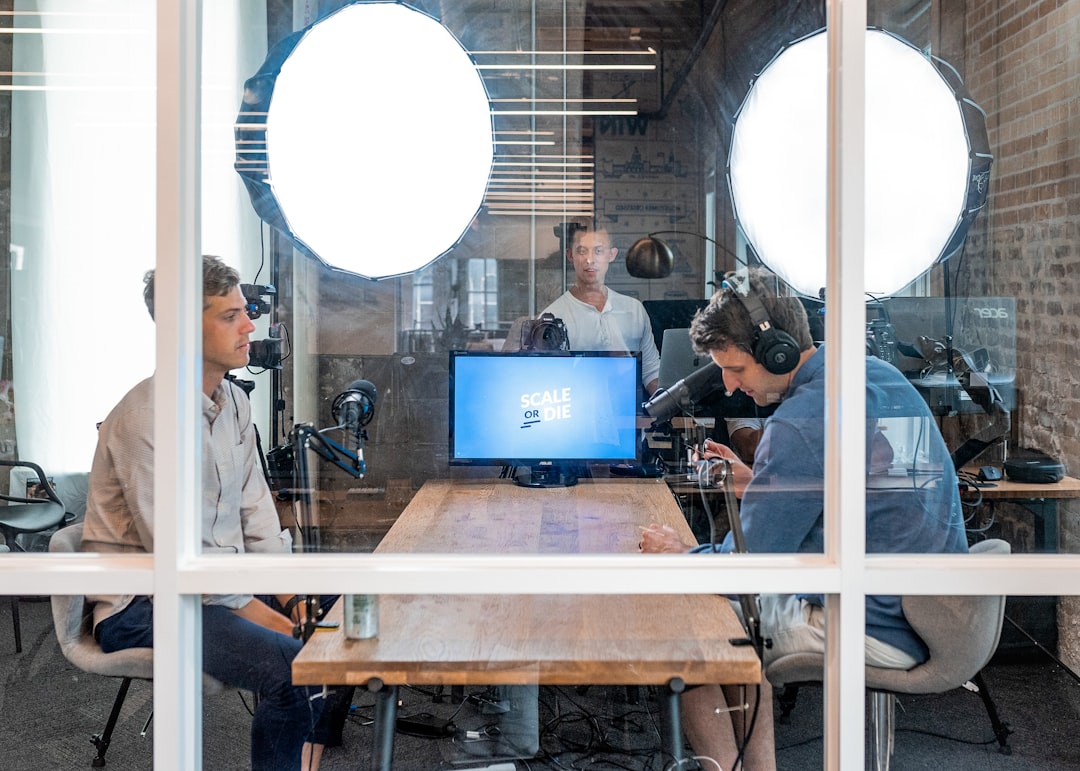
Live error detection, powered by Natural Language Processing (NLP), offers a new way to boost the accuracy of transcriptions. NLP uses clever algorithms to spot and fix mistakes as they happen, allowing for immediate corrections during podcast recordings. Techniques like machine learning and the use of n-grams help NLP continuously refine its performance, potentially leading to transcription accuracy close to 99%. While the technology is promising, there are hurdles. Training NLP models for various languages and accents can be complex and time-consuming. As NLP continues to mature, its capacity to improve the quality of real-time transcriptions is very promising, especially for tasks like creating high-quality transcripts of long podcast recordings.
Live error detection, powered by natural language processing (NLP), is revealing some truly fascinating capabilities. It's not just about catching typos; it's about understanding language in real-time. NLP systems can now adapt to the unique speech patterns of individuals, instantly correcting deviations from typical language structures. Imagine a system that can recognize your specific way of speaking and adjust accordingly – that's the level of personalization we're talking about.
Beyond simply recognizing individual quirks, these systems are getting smarter about context. They don't just rely on dictionaries to spot errors. They look at the surrounding words and the flow of the conversation to make informed decisions about whether something was a mistake. This helps them discern if someone misspoke due to the context of the conversation, rather than just making a basic grammar error.
Interestingly, some systems can now handle multiple dialects and accents. This is a significant step forward in making NLP more accessible in a globalized world where people speak with diverse linguistic styles. It's remarkable that a system can understand the nuances of regional accents and identify errors within those unique ways of speaking.
One intriguing feature is the real-time feedback loop. These systems can learn as they go. During a conversation, when they detect and correct errors, they use that feedback to refine their understanding for future interactions. So, the more you use the system, the better it gets at understanding your individual communication patterns.
Speaker identification is also being explored as a way to improve accuracy. By recognizing the different voices within a conversation, the system can apply tailored language models for each person, leading to more precise and personalized corrections. This capability is further enhanced by the ability to examine speech at the phoneme level. Systems can now analyze individual sounds, offering a more granular approach to error detection, particularly crucial in the fast-paced nature of live speech.
We're even seeing systems starting to integrate emotional cues. Analyzing tone can give a system a better understanding of the context of the spoken words. If someone sounds frustrated, it might make sense for the system to focus on fixing potentially critical errors right away.
The most obvious benefit of live error detection is the drastic reduction in post-transcription editing. By handling errors on the fly, the system dramatically speeds up the whole transcription process, resulting in near-instantaneous output.
Furthermore, these capabilities are being enhanced by transfer learning. Existing models trained on massive datasets are being adapted to specific niches without needing an entirely new training cycle. This allows the development of tailored systems for specific fields or contexts, like legal transcription or medical discussions. And, by collecting data on common errors encountered during live sessions, researchers are gaining insights that can drive targeted improvements in the technology. It's a dynamic process where continuous evaluation leads to steady progress.
While it's early days, the potential of live error detection in NLP is undeniable. These capabilities hold immense promise for refining and streamlining the transcription process and, frankly, it will be fascinating to watch how this area evolves in the coming years.
7 Proven Methods for Transcribing Long-Form Podcast Episodes with 99% Accuracy - Automated Background Noise Reduction Through Audio Filtering
Improving podcast audio quality, particularly in environments with a lot of background noise, is becoming increasingly important. This is especially true when aiming for highly accurate transcriptions. Older methods for noise reduction often struggled with audio that changes rapidly, sometimes introducing unwanted distortions. Newer methods using artificial intelligence (AI) and advanced algorithms have emerged that are specifically designed for handling these kinds of complex, fluctuating noise patterns. This means we can get much clearer speech against a backdrop of various noises, ultimately improving the quality of the recording. This, in turn, makes it easier for anyone trying to create a transcription of the podcast because the speech is easier to hear and understand. We can expect to see more automated audio filtering and noise detection in the future, as this kind of technology will be essential for getting great audio that makes high-quality transcriptions more feasible.
Background noise reduction is a critical aspect of audio processing, especially for podcasts recorded in environments with diverse and changing sound patterns. Traditional methods often fall short, particularly when dealing with dynamic noises that overlap with the speaker's voice, sometimes causing distortion in the process of trying to remove the noise. Newer noise reduction algorithms have emerged that are better equipped to handle rapidly changing and complex sound environments. These advancements help maintain the clarity of the speaker's voice against a backdrop of other sounds.
Effective background noise reduction is a multi-faceted approach. It combines the best recording practices with the sophisticated tools of audio post-processing, aiming for the best possible audio quality, which ultimately leads to better transcription results. There's a growing reliance on AI-powered solutions, like Auphonic and Cleanvoice, that can automatically clean up podcast audio through noise reduction, equalization, and level adjustments. This automation streamlines podcast production, leading to faster workflows.
Interestingly, deep learning techniques have proven their worth in the area of noise reduction, specifically within applications like speech recognition and telecommunications. Deep learning models are showing improved intelligibility in audio by carefully analyzing the audio and identifying patterns. Auphonic, a web-based post-production tool, also offers functionalities like batch processing and loudness standardization, adding to its versatility for creators working with a large number of episodes.
The field of background noise removal is certainly not new. We've seen constant innovation and advancements in audio processing technologies since the very invention of the microphone. However, the field is constantly evolving and, it seems, will continue to be a critical aspect of audio engineering. Noise reduction workflows often require a collaborative approach—a blend of traditional methods combined with the advancements in AI—to successfully handle the wide variety of noise conditions typically found in audio recordings. One might ask, how will audio engineers handle increasingly complex audio environments in the coming years?
While automated noise reduction offers significant benefits, it's also important to acknowledge potential limitations. Some techniques might introduce unnatural artifacts, which can compromise the quality of the resulting audio. A thoughtful balance is needed between the removal of background noise and the potential for altering the natural sound of the recording. Researchers and engineers will continue to push the boundaries in noise reduction, attempting to strike this balance even more effectively.
7 Proven Methods for Transcribing Long-Form Podcast Episodes with 99% Accuracy - Implementing Advanced Speaker Diarization Techniques
Within the realm of podcast transcription, accurately capturing who spoke when is paramount, especially for longer recordings with multiple speakers. This is where advanced speaker diarization steps in. By analyzing audio and labeling segments with the corresponding speaker, it significantly improves transcription accuracy. Different approaches to speaker diarization exist, including various software models. Some, like Pyannotespeaker, have shown promise in minimizing transcription errors related to speaker identification.
However, the task is not without its challenges. Accurately differentiating speakers when their voices overlap can be a difficult problem. Furthermore, as the number of speakers increases, the complexity of the task grows. Fortunately, progress in areas like deep learning and unsupervised machine learning continue to refine the ability of these techniques to sort through complex audio and reliably distinguish between speakers. This progress is crucial for achieving the high-accuracy transcription goals so important for many users of automated transcription systems.
7 Proven Methods for Transcribing Long-Form Podcast Episodes with 99% Accuracy - Real Time Quality Control Through Parallel Human Review
Having multiple people review the transcriptions while they're being generated offers a way to improve the accuracy of long-form podcast transcriptions. This "parallel review" means that errors can be caught and fixed right away. By having several people listening and comparing what they hear to the automated text, mistakes made by the automated system can be identified and corrected, resulting in a more reliable written version of the audio.
Beyond simply correcting errors, this approach helps capture subtle meanings and the context of the conversation that automated systems might miss. This is particularly useful in cases where audio quality is poor, speech overlaps, or there are different accents. These situations can be tricky for automated systems to handle, so having humans working in real time helps keep the transcribed text faithful to the actual podcast. While this method adds some overhead, the benefits of improved accuracy and the preservation of the nuances of a podcast are often worth the extra effort.
Having human reviewers work alongside automated transcription systems in real time offers a way to increase the accuracy of the final product. This approach recognizes that human reviewers can often pick up on subtle nuances and context that even advanced AI systems may miss. For instance, humans can use tone of voice and the rhythm of speech to understand meaning more effectively, something AI still struggles with.
This process can create a valuable feedback loop. Errors identified by human reviewers can help refine and improve the AI models used in the transcription process over time, leading to more accurate transcripts in the future. Additionally, it seems to make the overall task of creating transcripts less demanding by splitting the work more effectively between humans and machines. Human reviewers can focus on the aspects that require deeper understanding, while the AI can handle more repetitive aspects of the process.
This type of human oversight also lets the transcription process adapt more easily to evolving speech patterns and unique vocabulary, particularly useful in situations like podcast transcriptions, where conversation topics can be unpredictable and vary widely. This capability to make rapid adjustments based on context is something that solely automated methods cannot easily achieve.
Of course, human reviewers also excel at recognizing aspects of human language that still challenge AI, such as figurative language, humor, and cultural references. This highlights the limitations of purely machine-driven systems when it comes to understanding the full range of linguistic complexities, especially in unscripted settings.
While the initial implementation of human review can add time to a process, the potential to significantly reduce post-transcription editing can lead to a faster overall workflow. By catching mistakes as they happen, time isn't wasted on extensive edits later. Interestingly, using reviewers with diverse linguistic backgrounds seems to further enhance accuracy, especially for transcriptions that involve multiple speakers with different accents or dialects.
It's worth noting that integrating human and AI approaches raises questions about privacy and who is responsible for the final product. Using human reviewers effectively requires thoughtful attention to these ethical concerns, to make sure the process is both accurate and responsible.
Essentially, this parallel human review approach represents a hybrid solution that attempts to harness the strengths of both human understanding and machine efficiency. This synergistic approach could lead to a more refined and ultimately more accurate transcription process for all kinds of audio content, including long podcast episodes. It's an area ripe with ongoing research and refinement as the capabilities of AI evolve.
More Posts from transcribethis.io: