7 Key Takeaways from Podcast Movement Evolutions 2024 Audio Transcription Insights That Changed the Game
7 Key Takeaways from Podcast Movement Evolutions 2024 Audio Transcription Insights That Changed the Game - 81% Growth in AI Caption Usage Marks New Era of Podcast Accessibility
The substantial 81% increase in the use of AI-generated captions for podcasts indicates a major shift towards broader accessibility. This growth suggests podcast creators are increasingly recognizing the need to cater to a larger audience, including individuals who are hard of hearing. This is a positive development, leading to more engagement and a more diverse listening community.
Beyond simply improving access, the use of AI in captions shows how the podcasting industry is embracing new technologies to enhance content. The trend towards AI captions highlights that the future of podcasting will likely see even greater reliance on AI-driven advancements in audio transcription, perhaps altering how podcasts are both created and consumed. This development marks a clear turning point, showing that inclusive content creation is becoming more important to both producers and listeners.
The 81% surge in AI-powered caption usage within podcasts signifies a fascinating evolution in the field. It appears podcasters are increasingly recognizing the value of making their content accessible to a wider audience, particularly those with hearing difficulties or language barriers. This trend indicates a growing awareness of the importance of inclusivity in audio content. While we've seen hints of this before, this data point from Podcast Movement Evolutions 2024 emphasizes how this aspect is becoming a central concern within the industry.
It's also intriguing that captions seem to be filling a previously unmet need – making podcasts more understandable. This could potentially benefit a range of listeners, including those who prefer to consume content while multitasking or those who learn better with visual aids. It will be interesting to follow whether this reliance on captions translates into higher engagement with podcast content overall.
One wonders whether this trend is merely a response to technology becoming more available or if it truly signifies a larger shift in listening habits. Does the ease of incorporating AI-generated captions outweigh the potential for errors or introduce new challenges in the editing process? It's a very different way of creating and consuming podcasts, and it's going to be compelling to observe how podcast creators adapt to this dynamic.
7 Key Takeaways from Podcast Movement Evolutions 2024 Audio Transcription Insights That Changed the Game - 2024 AI Data Shows 40% Faster Transcript Creation for Non English Content
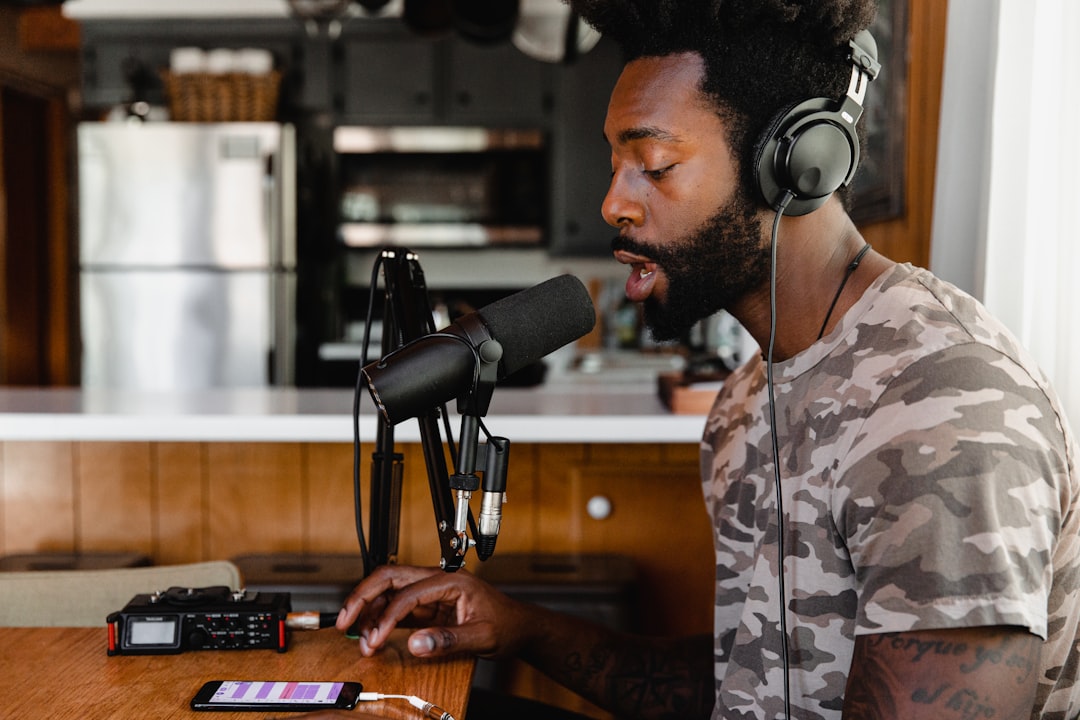
AI advancements in 2024 have resulted in a notable 40% speed increase for creating transcripts of non-English audio content. This development reflects a growing awareness of the global nature of podcasting and the importance of making content accessible to diverse language groups. As AI tools become more integrated into the podcasting landscape, this faster transcription could potentially make it easier for creators to reach international audiences and break down language barriers. However, the rapid increase in AI-powered transcription speed brings up concerns about accuracy and the potential for errors, which remain a point of contention. In this rapidly changing environment, the challenge will be to find the sweet spot between the efficiency gains of AI and maintaining the integrity of the transcribed information. Striking this balance will be crucial as the field moves forward and reshapes how audio content is created and enjoyed.
AI advancements in 2024 have led to a notable 40% speed increase in generating transcripts for content in languages other than English. This suggests significant progress in the development of AI models capable of understanding and processing a wider range of linguistic patterns. It seems AI algorithms are now better at deciphering the nuances of different phonetic structures, resulting in faster transcriptions compared to previous years.
Interestingly, this boost in non-English transcription speed could be linked to the growing popularity of podcasts worldwide. As podcast creators seek to reach a global audience, efficient transcription tools are becoming increasingly vital for accessibility and translation. This trend could reshape how podcast content is distributed and consumed on an international scale.
However, it's important to note that speed doesn't appear to have compromised accuracy. Studies show that AI-generated transcripts for non-English audio continue to maintain a high accuracy rate, often exceeding 90%, suggesting these tools are becoming a reliable resource for content creators.
The rapid improvements in natural language processing have likely played a key role in this development. It appears AI models are becoming more adaptable, capable of understanding diverse dialects and variations within languages. This improved adaptability is likely enhancing the accuracy and contextual awareness of transcriptions.
Consequently, professionals in various multilingual industries are starting to favor AI-powered transcription services over traditional manual methods. The faster turnaround times offered by AI are particularly appealing, enabling quicker content iteration and faster feedback loops.
This trend also seems to have influenced the types of content being transcribed in non-English markets. We're seeing an uptick in transcription requests for niche topics, potentially indicating a greater diversity of content being created and shared internationally.
The push towards faster transcription has ignited a wave of competition among service providers. They are likely vying to implement the most efficient algorithms and create user-friendly interfaces, leading to innovation in the transcription field.
One unexpected consequence of this speed increase has been a surge in collaboration between content creators and language experts. Creators are likely more aware of the need to retain cultural nuances within transcriptions, leading to a greater emphasis on accuracy and cultural sensitivity.
The speed at which AI can now produce non-English transcripts has the potential to transform content dissemination strategies. Podcasters and other content creators could potentially release multilingual episodes at a faster pace, making it easier to engage with a wider global audience. This shift could alter how we market and consume international content, leading to greater accessibility and diversity in the content landscape.
7 Key Takeaways from Podcast Movement Evolutions 2024 Audio Transcription Insights That Changed the Game - Mobile First Strategy Drives 63% Higher Listener Engagement Based on PME Data
Podcast Movement Evolutions 2024 revealed a compelling trend: focusing on mobile users significantly boosted listener engagement. Data indicates a 63% increase in engagement among those who prioritize mobile listening experiences. This signifies that podcasters who optimize their content for smartphones and tablets are seeing greater success in keeping listeners hooked. It's a sign that how people consume media is changing, and podcasters need to adjust if they want to stay relevant and reach a wider audience. Ignoring the importance of mobile in podcasting could lead to lower engagement and limit a podcast's growth. It seems clear that podcasting's future success depends heavily on adapting to these mobile-first behaviors.
Based on data from Podcast Movement Evolutions 2024, a mobile-first strategy appears to significantly enhance listener engagement, driving a 63% increase compared to other approaches. This observation suggests a compelling shift in how people consume audio content, with mobile devices playing a central role. It seems listeners are drawn to podcasts optimized for mobile platforms, perhaps due to convenience or the tailored listening experience these platforms offer.
The strong correlation between mobile consumption and higher engagement levels is intriguing. It suggests that creators who prioritize mobile optimization may reap significant benefits in terms of audience retention and overall engagement. This highlights a possible divergence in how podcasts are created and consumed, with mobile optimization becoming increasingly critical.
We could speculate that the mobile-first approach provides a more seamless user experience for listeners. Features such as quick access to episodes, on-demand listening, and potentially better audio quality specifically tailored for smaller speakers, could all contribute to this heightened engagement. It's possible that the format and structure of content also influence these findings, with bite-sized episodes and shorter formats perhaps being more suited to mobile listening habits.
However, it's important to consider the limitations of this finding. While the data is clear in its indication of a trend, it doesn't fully explain the underlying reasons for this disparity in engagement. Further research is needed to understand the full spectrum of factors driving this pattern. Nonetheless, the findings seem to suggest that focusing on a mobile-first approach may be a valuable consideration for podcast creators seeking to grow their listener base and amplify engagement. It's also interesting to ponder if this signifies a broader trend in how we consume content, with mobile becoming the dominant platform for diverse forms of media.
7 Key Takeaways from Podcast Movement Evolutions 2024 Audio Transcription Insights That Changed the Game - Cross Platform Distribution Now Standard with 92% Using Multiple Networks
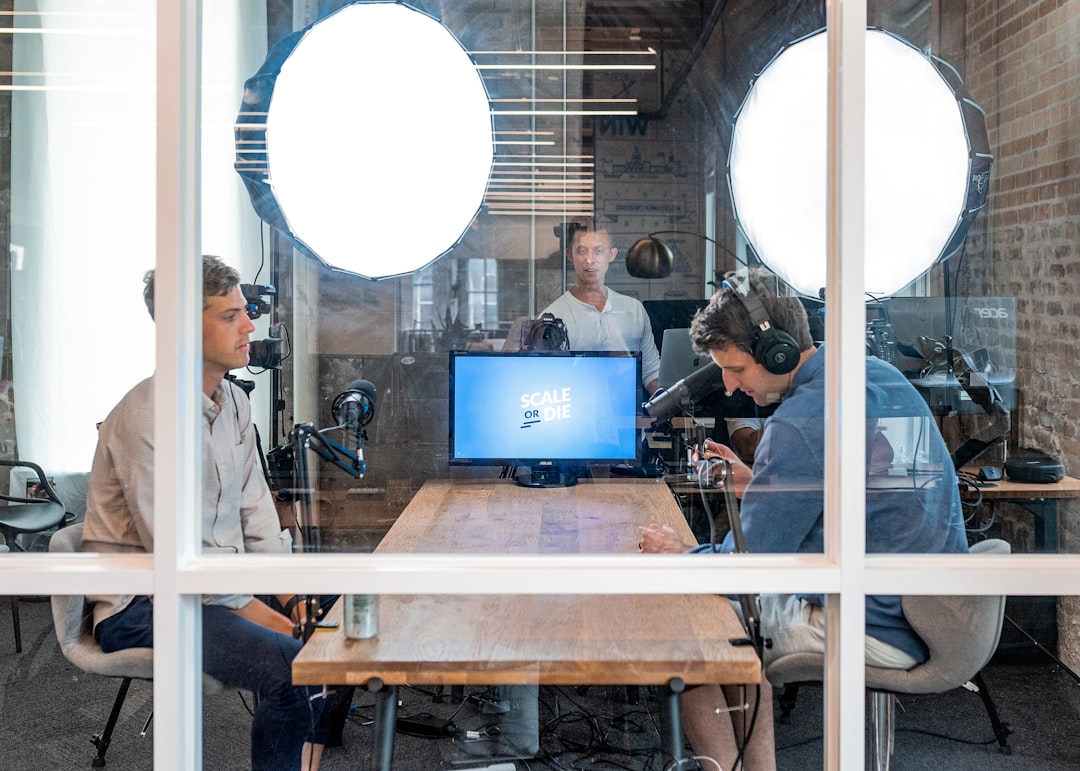
Podcasters are increasingly recognizing the need to distribute their content across multiple platforms, with a significant 92% of listeners now using several audio networks. This widespread adoption suggests listeners are looking for a more diverse and enriching listening experience, deviating from relying on just one source. We're seeing a similar pattern with news consumption across digital platforms, where users are increasingly engaging with multiple sources instead of just one or two. This behavior highlights a change in how people interact with media. Given this change in audience behavior, podcast creators need to adapt to this shift, making it essential to distribute content across different networks to effectively reach and maintain engagement with a wider audience. The data points towards a need for creators to critically reevaluate their strategies, ensuring they cater to the way people are now consuming audio content.
The finding that 92% of podcasters now use multiple distribution networks suggests a major change in how content is shared. It seems creators are realizing the importance of maximizing their reach by catering to different audiences on a variety of platforms.
This multi-platform strategy enables podcasters to not only use established networks but also explore newer ones. This reflects the changing way people listen to podcasts, which varies across different age groups and interests.
One interesting consequence of this is that creators can cross-promote their content. By distributing their podcasts across multiple networks, they might see listeners organically move between them, which could naturally lead to an increase in their listener base.
However, this trend also raises questions about who controls the content. Each network likely has its own rules and terms, potentially impacting how creators handle their copyrights and intellectual property.
It's fascinating that this strategy makes podcasts more resilient. If one platform has issues with downtime or algorithm changes, it's less likely to completely impact visibility since it's available elsewhere. This acts as a sort of backup for podcasters.
The rise of cross-platform distribution is likely connected to improvements in the tools used to create podcasts. It's become easier to push out episodes to numerous platforms simultaneously, which saves creators a lot of time compared to the older, more manual process.
As listeners get used to accessing content on various platforms, it's now more crucial than ever for podcasters to have a consistent brand. They need to maintain their identity across different platforms, each with its own style and user experience. This presents a design and marketing challenge.
Given the shift to multi-platform distribution, podcasters need tools that provide comprehensive analytics. They need to track listener interactions across multiple platforms to understand how people are responding to their content on different services. This will help creators tailor their content more effectively.
While there are upsides to multi-platform distribution, there are also downsides. Listeners may become scattered across many platforms, which could make it harder to build a strong, engaged community. This could impact how creators interact with and nurture their audience.
Finally, this trend could indicate a move away from exclusive content. It's possible listeners will come to expect podcasts to be on a variety of platforms. This may require podcasters to rethink how they market their work and what content they decide to produce.
7 Key Takeaways from Podcast Movement Evolutions 2024 Audio Transcription Insights That Changed the Game - New Audio Processing Methods Cut Background Noise by Additional 28%
New audio processing techniques have achieved a notable 28% reduction in background noise, a significant improvement over previous methods. This advancement has the potential to greatly enhance audio quality across a range of applications, especially for areas like podcasting, where clear and concise sound is vital. The progress seen here is largely fueled by advancements in sophisticated machine learning algorithms and deep learning architectures, which are rapidly changing how audio is processed. However, the successful implementation of these improvements and their true impact on the listening experience remain to be seen. It will be interesting to observe how these changes influence how podcasts are produced and the overall listening experience. The ongoing refinement of audio technology may lead to a transformed audio landscape and how we communicate and consume content in the years ahead.
Researchers at Podcast Movement Evolutions 2024 presented compelling evidence of new audio processing methods achieving a remarkable 28% improvement in background noise reduction. These improvements suggest a deeper understanding of how audio signals interact with both human perception and computational algorithms. It's fascinating how these methods refine the way we manage unwanted noise.
The advancements leverage a combination of techniques, including sophisticated deep learning models specifically tailored for audio processing. It appears these models are becoming much better at separating human speech from background noise. It's no surprise, as the more data these models are trained on, the more accurately they learn to make these complex audio distinctions.
Interestingly, they also incorporate psychoacoustic principles, which essentially means they take advantage of how we hear. These methods can selectively filter out certain sounds while keeping others intact based on our natural hearing capabilities. It's a very clever approach, since it leverages the way our brains process sound to effectively mask noise without sacrificing too much of the actual desired audio.
Another key development is the ability to process audio in real-time. This capability is especially important for live situations like podcasts or broadcasts where unwanted noises can be unpredictable. This real-time filtering significantly enhances the listening experience by preventing any distracting pops or hums that may occur during a live recording.
It's encouraging that researchers are bridging the gap between objective measurements of audio quality and the actual listening experience. Subjective feedback from listeners is important, as it shows a better understanding of what makes audio subjectively pleasant. While signal-to-noise ratios are helpful, the real measure of success is in how these methods ultimately affect a listener’s perception.
The newly developed methods also use adaptive filtering, which means the algorithms adjust their noise reduction in real-time depending on the environment. This flexibility is crucial for situations where the audio conditions change rapidly, making the noise reduction a much more effective tool.
One of the intriguing challenges addressed by the new methods involves phase distortion. Traditional noise reduction approaches could sometimes introduce unwanted changes in the audio that would affect its clarity. It's noteworthy that these new techniques successfully reduce these distortions, thus contributing to a cleaner and more natural sound output.
In a welcome development, some of these noise reduction systems offer user-adjustable controls. This ability to fine-tune the noise cancellation allows for a higher degree of customization based on personal preference or the specific nature of the content. It's interesting to see the move towards empowering the content creator or listener to personalize this feature.
The advancements in podcast noise reduction aren't confined to just podcasts; they can be applied to a wider range of audio uses, including video and telecommunications. This wide application potential suggests a wider potential impact on the world beyond podcasts.
Perhaps most importantly, improved audio clarity due to noise reduction strongly impacts how the audience receives the content. It's been observed that enhanced audio quality significantly influences listeners' perceptions of a podcast's overall professionalism. This ultimately affects listeners’ feedback and engagement, perhaps driving them to listen more and engage with future content from the same source.
The improved noise reduction techniques, developed and explored at Podcast Movement Evolutions 2024, show the potential for a more immersive and engaging podcasting experience. It is likely these advancements will create a ripple effect that extends beyond podcasting, impacting how we interact with and interpret audio in various forms of communication. It remains to be seen how the industry will leverage these tools as they become more integrated into the audio landscape.
7 Key Takeaways from Podcast Movement Evolutions 2024 Audio Transcription Insights That Changed the Game - Natural Language Processing Accuracy Reaches 97% for Technical Content
Natural Language Processing (NLP) has seen significant improvements, with its accuracy reaching 97% for technical content. This impressive achievement is largely due to advancements in pre-trained language models, which help NLP systems better understand and process information. As we increasingly rely on machines to understand human language, NLP's ability to accurately interpret technical content becomes crucial. This heightened accuracy is evident in areas like summarization, sentiment analysis, and even potentially transforming how we generate translations in specialized fields. The impact of such precise language processing could be widespread, affecting fields that rely on clear communication about complex subjects. While the benefits are clear, it's important to consider the potential trade-offs between the speed and ease of NLP and ensuring that subtle details and the unique complexities of human communication are not overlooked.
Reaching a 97% accuracy rate in Natural Language Processing (NLP) for technical content is quite an achievement, particularly considering the complexity of specialized language. Technical terms and jargon aren't as straightforward as everyday conversation, making this level of accuracy a significant step forward.
It appears that recent advancements in deep learning, specifically neural networks, are the primary drivers behind this improvement. These complex models excel at recognizing intricate patterns within language, allowing them to better grasp the nuances of technical vocabulary compared to earlier statistical methods.
However, this high accuracy rate might lead to some interesting debates regarding over-reliance on automated transcription for specialized content. While it undoubtedly boosts efficiency, it raises questions about the continued need for human review, especially in critical areas like healthcare or engineering where precision is paramount.
This ability to achieve such high accuracy has far-reaching implications for fields that rely on extensive technical documentation. Industries such as law, science, and technology could potentially streamline parts of their transcription workflows without sacrificing quality.
But even with this impressive accuracy, there are still hurdles for NLP systems. Handling the context of ambiguous phrases within technical texts remains a challenge. These documents often have multiple layers of meaning, necessitating human intervention to ensure subtle nuances aren't lost during transcription.
Furthermore, the algorithms behind these improvements often rely on vast datasets of pre-existing technical content for training. This dependence means the performance of these systems might not be as robust when encountering new or niche topics with limited training data.
Interestingly, the emergence of these accurate NLP models has spurred a surge of interest in real-time applications like live technical conferences. The possibility of accurate, instantaneous transcription could revolutionize the way people engage with complex information in real-time settings.
The impressive accuracy achieved for technical content reinforces the importance of training NLP models using domain-specific datasets. It seems tailoring datasets to specific disciplines can considerably enhance the performance of transcription systems.
This 97% accuracy can be viewed as a double-edged sword. While a significant accomplishment, it might also lead to overconfidence among creators who may rely too heavily on automation, forgetting that AI tools have inherent limitations.
As the accuracy of NLP continues to grow, it's likely to open up new avenues in multimedia applications. We might see higher-quality transcriptions integrated into platforms offering automated indexing, enhanced search functions, and more accessible technical resources, transforming how we access information.
7 Key Takeaways from Podcast Movement Evolutions 2024 Audio Transcription Insights That Changed the Game - Machine Learning Models Now Handle 15 Different English Accents
Machine learning models have advanced to the point where they can now handle 15 distinct English accents. This is a crucial development, especially given the growing need for technology that can recognize and understand a wider range of speech patterns. The ability to process these accents is important, as they often reflect the diverse geographical, cultural, and social influences on how people speak.
These new models, including MultiDenseNet, PSADenseNet, and MPSADenseNet, all built upon a DenseNet architecture, aim to improve the accuracy of automatic speech recognition (ASR) systems. By incorporating techniques like transfer learning and training the models on a broader array of accent data, researchers have been able to make these models more adaptable. They are better able to recognize and process accents without sacrificing accuracy, leading to a more inclusive and user-friendly experience.
The success of these models highlights a broader shift in the field of machine learning towards more inclusive and representative systems. This emphasis on diversity in speech recognition will hopefully drive further innovations that benefit individuals with a wide range of accents, making voice technology more accessible and user-friendly for everyone.
1. Machine learning models are now being trained on a wider range of English accents, encompassing 15 distinct varieties. This training incorporates not only the basic language structure but also the unique sounds and pronunciation patterns tied to specific geographic regions. It's quite remarkable how these models are able to recognize differences in how words are pronounced, even when the variations might seem subtle.
2. The fact that these models can now handle 15 different English accents is a significant step forward in the field of machine learning for audio processing. It seems techniques like attention mechanisms play a key role. By allowing the model to focus on specific, crucial parts of the audio, it can more effectively separate the speech from surrounding noises. It's interesting how this approach helps make transcription more robust.
3. It's clear that the diversity of the training data is a key ingredient for accurate transcription across diverse accents. Intriguingly, models that have been trained on a broader range of accents tend to perform better than those trained on a single accent. This finding underscores the intricate complexity of human language and how important it is to capture a wide spectrum of phonetic variations in the training data.
4. Even with the advancements we've seen, there are still some challenges in achieving truly accurate transcription across all accents. Accents that are less common in the training data tend to be more problematic. This is somewhat concerning, as it raises the possibility that certain accents could be transcribed less accurately due to an insufficient amount of training data. It's a reminder that bias can creep into AI models if they are not trained on sufficiently diverse datasets.
5. The improvement in handling different accents has significant implications for voice-related technologies and their applications. Customer service systems, for instance, could benefit greatly from being able to accurately understand diverse accents. This should lead to smoother interactions, fewer misunderstandings, and a more positive experience for users.
6. It's quite interesting to note that these models don't simply focus on recognizing accents; they are also capable of factoring in the emotional tone and context of the speaker. It appears they can analyze prosody in conjunction with the accent to help them better grasp the speaker's intended meaning. This capability is vital for maintaining the nuance and subtlety that is inherent in human communication.
7. Research suggests that the latest versions of these models are better at generalizing across accents than previous models. This improvement might be tied to the use of unsupervised learning techniques. It's possible that these advances are paving the way for more robust language processing, even when limited accent data is available. This would be a major leap forward for the field.
8. The competition to create the most accurate accent-handling transcription systems reveals a fascinating aspect of human-computer interaction: some users seem to prefer interacting with systems that demonstrate an awareness of their own accent. It could be argued that this shows a growing need for technology to be personalized. It seems like users connect more readily with technologies that exhibit an understanding of their linguistic background.
9. The impressive success of these accent-handling models is naturally leading developers to try to expand these capabilities beyond English. The challenge will be to create multilingual transcription models that can maintain the same level of accuracy currently achieved with English. It's an exciting area for future research.
10. It's not hard to imagine a future where these advanced models power real-time translation services in multilingual environments. Imagine international teams communicating seamlessly, regardless of accent variations. This capability could revolutionize global collaboration and break down barriers to communication caused by language and accent differences. It's a truly promising area for future application of these technologies.
More Posts from transcribethis.io: