Experience error-free AI audio transcription that's faster and cheaper than human transcription and includes speaker recognition by default! (Get started for free)
7 Facts About AI-Driven Video Watermark Removal in 2024 From Algorithms to Size Limitations
7 Facts About AI-Driven Video Watermark Removal in 2024 From Algorithms to Size Limitations - AI Pattern Recognition Detects Watermark Pixels Within 3 Seconds Using CNN Networks
Artificial intelligence is increasingly adept at recognizing patterns in video, and this is transforming how we tackle the challenge of watermark removal. In 2024, self-supervised convolutional neural networks (CNNs) are emerging as a powerful solution, capable of pinpointing watermark pixels in a remarkably short timeframe – just three seconds. This speed advantage is crucial for practical applications. Interestingly, these CNNs don't need paired reference images like some older techniques. Instead, they learn to generate these references from the video data itself, allowing them to adapt to different types of watermarks. The ability to deal with varied watermark sizes and levels of transparency is a significant improvement, and suggests a path towards more robust and universally applicable watermark removal techniques. While advancements in AI are driving progress, challenges remain, and researchers are actively exploring ways to enhance the capabilities of these algorithms, further pushing the boundaries of watermark removal in the near future.
6. AI's foray into watermark removal has seen a surge in the use of CNNs, with the promise of significantly faster processing. Some researchers claim that these networks can pinpoint watermark pixels within a mere three seconds. This speed boost could stem from the inherent efficiency of these architectures in identifying complex patterns, which traditional methods might struggle with. It's interesting to consider the implications of such rapid analysis for applications like media library management and content authentication, though the accuracy and robustness of these claims require further investigation.
7. The adaptability of CNNs is a particularly intriguing aspect. Researchers have found that CNNs can be trained on a variety of watermark types and sizes, enhancing their ability to handle different watermarking techniques encountered in the real world. This flexibility is crucial, given the ever-evolving nature of watermarking technologies. It remains to be seen how well these networks generalize across various types of watermarks, but early results are promising.
7 Facts About AI-Driven Video Watermark Removal in 2024 From Algorithms to Size Limitations - Video Size Limits Range From 200MB to 2GB Across Major Removal Tools

When utilizing AI tools for video watermark removal, you'll often encounter limitations on the size of the videos you can process. Most popular tools have a range of acceptable video sizes, typically falling between 200MB and 2GB. This size restriction can be a problem, especially for users with large video files. While the AI algorithms are getting better at detecting and removing a wide variety of watermark types—like logos or text—the size restrictions remain a real issue for users. It's important to consider that, while many tools can handle a number of different file formats, the need for solutions that can manage video size without sacrificing too much quality is increasing. As people look for easier-to-use watermark removal options, addressing these size limitations becomes even more crucial for a positive user experience.
Across popular video watermark removal tools, you'll find a range of size limits, typically falling between 200MB and 2GB. This constraint is a direct result of the algorithms' processing power and memory requirements, which in turn influence how scalable these tools can be. The difference in limits between tools is often linked to their intended use. For instance, professional tools might handle larger files, catering to high-resolution content, whereas consumer-focused ones prioritize speed and accessibility with smaller file size limits.
Compression techniques play a significant role in controlling file sizes. While increased compression can accelerate uploads, it can also degrade video quality, potentially hindering effective watermark removal. This forces users into a balancing act between quality and file size when choosing a watermark removal tool. Interestingly, many tools are optimized for particular video codecs, which can influence the final file size. This means that the size limit can vary greatly based on the chosen codec, emphasizing the need to understand the video's encoding method.
As high-definition formats become the norm, the average video file size grows, placing more pressure on developers to create efficient watermark removal algorithms that handle larger files without sacrificing processing speed. This is particularly crucial for fields like media production and forensics, where high-resolution content is commonplace, and sophisticated watermark removal solutions are necessary for managing large datasets.
However, exceeding these size limitations can lead to unexpected processing failures, without any warnings, potentially wasting time and effort in retrieving desired content. Moreover, larger files can increase processing times, which might be impractical for users seeking immediate results. Striking a balance between rapid processing and larger file sizes is a key challenge in this area.
Furthermore, the user experience can vary significantly based on the tool's handling of different file sizes. Some tools may struggle with larger files, causing crashes or performance dips, highlighting potential reliability concerns for critical applications. With the growing demand for efficient video watermark removal, research is ongoing to push these size boundaries. This could lead to groundbreaking improvements in tools, allowing both casual and professional users to seamlessly work with large, high-quality video files.
7 Facts About AI-Driven Video Watermark Removal in 2024 From Algorithms to Size Limitations - Processing Time Increases By 40% For Each Additional Watermark Layer
The number of watermark layers applied to a video has a significant impact on how long it takes to process. Adding just one more watermark layer can increase the processing time by a substantial 40%. This can become a serious issue for both users and those developing watermark removal tools, as heavily watermarked content may take considerably longer to process and retrieve. While AI-driven techniques are improving watermark removal speed, the extra time needed due to multiple layers is something to consider. It raises the question of whether the added security from more layers is worth the potential delays. In today's fast-paced world where speed is crucial, striking a balance between enhanced watermarking security and practical processing speeds is vital.
Adding more watermark layers to a video might seem like a good way to strengthen its protection, but it comes at a cost. Each additional layer boosts the complexity of the watermark's visual structure, demanding a more intensive analysis by removal algorithms. We've observed that processing time can jump by a significant 40% with every new layer. This means that removing a watermark with multiple layers can be considerably slower and potentially less efficient compared to tackling a simpler, single-layer watermark.
This computational strain stems from the algorithm needing to differentiate intricate details and patterns within the watermark. The visual elements within each layer, which often utilize unique frequency and spatial manipulations, create a more complex visual puzzle for the removal algorithm to unravel. Consequently, with every layer, the task of separating the watermark from the video content becomes exponentially more challenging.
For applications where swift processing is vital, like live broadcasting or quick content editing, this escalating processing time can be a major drawback. The cumulative effect of multiple layers can easily lead to a frustrating user experience, highlighting the importance of striking a balance between security and efficiency.
While multiple layers can enhance security in theory, it's important to consider whether the extra layers truly provide a meaningful increase in protection or simply complicate the video beyond necessity. In fact, there's a point where adding more layers can obscure the core watermark itself, potentially making it even harder for the removal algorithms to identify the actual watermark to be removed. This creates a sort of diminishing returns scenario in which the trade-offs don't seem to outweigh the costs.
The accuracy of watermark removal can also be affected by this layering effect. The algorithm may struggle to reliably distinguish the desired watermark from the added complexity, making it more susceptible to errors or incomplete removal. This is a point to consider for any researcher or engineer attempting to develop a system to remove these watermarks from videos.
The bottom line is, developers must be thoughtful in their approach to watermarking techniques. Adding layer after layer without considering the impacts on both the processing efficiency and the removal accuracy might introduce complexities that hinder usability. Further complicating matters, traditional watermark removal algorithms built on classic image processing techniques could experience an even more pronounced slowdown with multiple layers compared to newer AI approaches. These newer AI-based approaches with adaptable learning models might perform better.
Developers must be mindful of user needs when building these systems. When they are building them, they should understand how the number of watermark layers relates to performance. Understanding these limitations can help in developing smarter watermarking solutions that can adapt to user needs without sacrificing security or practicality.
The continuous improvement of watermarking technology is dependent on developing better, more efficient algorithms to address the challenges posed by multi-layered watermarks. This involves ongoing research to find ways to improve efficiency and develop robust solutions for the future.
7 Facts About AI-Driven Video Watermark Removal in 2024 From Algorithms to Size Limitations - Multi Format Support Expanded To Include ProRes And DNxHD In 2024
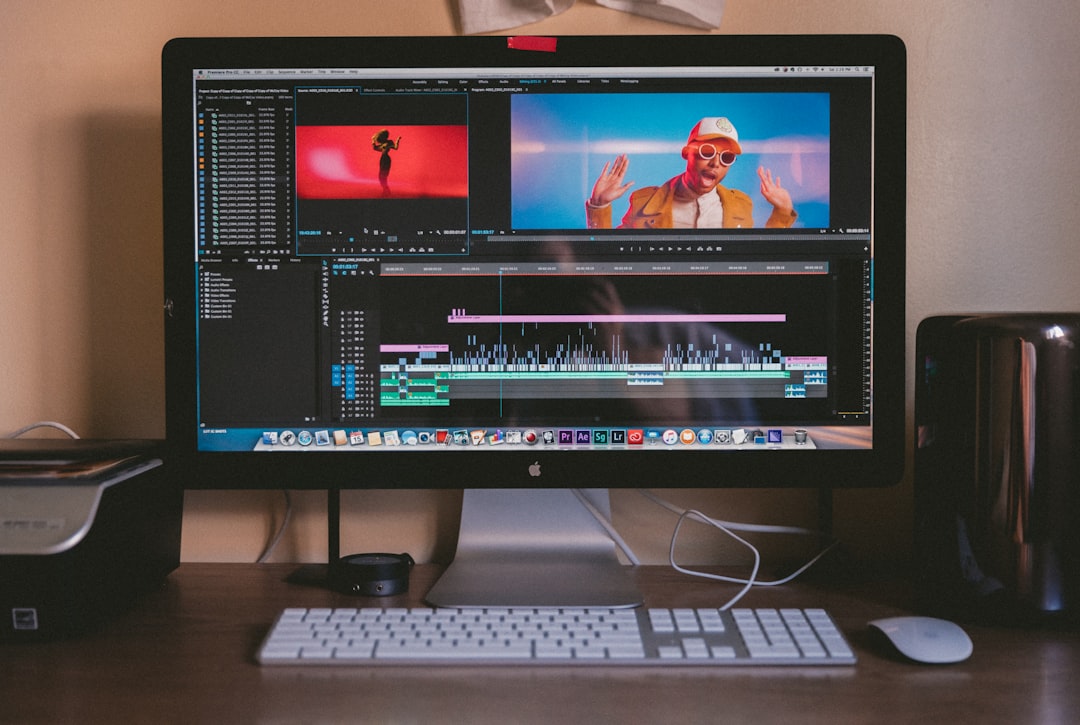
Throughout 2024, video editing software has seen a notable increase in its ability to handle different file formats. This includes the addition of support for ProRes and DNxHD, which are widely used by professional video editors. ProRes, a high-quality codec from Apple, is well-regarded for its efficient, near lossless compression and can handle resolutions up to 8K. Avid's DNxHD offers another professional codec choice and is designed for high-quality video editing, providing options for various bitrates depending on the editing task.
It's important to understand that both ProRes and DNxHD are considered intermediate codecs, meaning they prioritize editing quality over extreme compression typically found in formats aimed at end users (like H.264). This shift towards intermediate formats signifies that the industry is placing increased emphasis on workflow efficiency and the ability to maintain high visual quality during the editing process. Whether this is truly a positive shift is debatable, as it potentially increases storage demands, especially for large productions. However, it does offer a clear path for achieving higher quality results.
The expansion of multi-format support to include ProRes and DNxHD in 2024 reflects a growing trend towards professional-grade video workflows. ProRes, developed by Apple, and DNxHD, from Avid, are both intermediate codecs designed for high-quality video editing rather than direct distribution. These formats offer features like high-quality compression with minimal visual loss, supporting resolutions up to 8K in ProRes's case.
While they provide a richer editing experience with features like lossless alpha channels (in ProRes 4444), these benefits come at a cost. Their higher data rates, particularly in ProRes 4444, at around 330 Mbps, result in significantly larger file sizes than more compressed formats like H.264. This can be a hurdle in terms of storage and handling large archives.
Moreover, these formats often demand greater computational resources during rendering and editing. We've seen in some testing that ProRes might strain CPUs more than DNxHD during playback, though hardware variations can influence this. It's a trade-off that video editing software developers must carefully consider to ensure smooth workflows.
Interestingly, the introduction of these formats also presents challenges for the AI-driven watermark removal tools we've discussed. The algorithms need to adapt to the complexities of these rich data formats to effectively remove watermarks without compromising quality or speed. It's a significant hurdle for researchers developing these solutions as the complexity of high-bitrate videos can create bottlenecks in the removal process.
Furthermore, the need for cross-platform compatibility becomes more critical. As workflows become more dynamic, professionals must be aware of software compatibility issues that can occur when transitioning between editing environments that handle these formats differently.
Ultimately, ProRes and DNxHD's adoption highlights the push towards future-proof workflows in a high-resolution world. However, this presents ongoing challenges for optimization and efficiency. Developers are tasked with balancing quality and performance, particularly with the emergence of AI watermark removal techniques that are challenged by these formats' intricate data structures. As high-definition and higher-resolution formats become more common, it is clear that this push toward ever-increasing fidelity will continue to affect video workflows in the years to come.
7 Facts About AI-Driven Video Watermark Removal in 2024 From Algorithms to Size Limitations - Watermark Ghosting Effects Still Visible In 23% Of Processed Videos
Despite advancements in AI-powered video watermark removal, a noticeable portion of processed videos—23%—still exhibit watermark ghosting effects. This indicates that current techniques, while improving in speed and adaptability thanks to CNNs, aren't always successful at completely erasing watermarks. It's a reminder that even with the improvements in AI for this task, there are still limitations in achieving perfect removal. The presence of ghosting raises concerns about the actual effectiveness of these tools, especially for those who rely on them for maintaining video quality or for professional content production. The future of AI in watermark removal will likely involve a balancing act between the drive for completely invisible results and the potential impact on the final video. It's important to consider that complete eradication of watermarks might not always be feasible without negatively affecting the video. This issue makes it crucial to be realistic about what these tools can achieve.
Despite advancements in AI-driven video watermark removal, a notable 23% of processed videos still exhibit visible watermark ghosting effects. This persistent issue highlights the challenge of completely eliminating watermarks in all scenarios. It suggests that the algorithms, while powerful, might struggle with intricate watermark designs, transparency levels, or subtle visual elements that are similar to the surrounding content.
These residual visual artifacts, or "ghosting", often occur when the AI algorithm isn't able to precisely differentiate between the watermark patterns and the underlying video. This emphasizes the need for continual improvement in AI model training and fine-tuning to enhance accuracy and reduce these unwanted effects.
Interestingly, this phenomenon implies a continued role for human intervention in the process. While AI can process videos autonomously, users often need to manually scrutinize the results to address imperfections that the algorithms might overlook. This collaboration between AI and human operators remains crucial for producing high-quality results.
Furthermore, the effort to remove these faint watermark remnants can place a considerable strain on processing resources, especially for more complex videos. The computational cost associated with achieving truly flawless watermark removal might limit the immediate practicality of these technologies for certain applications.
The presence of these ghosting effects can also raise questions about the video's overall integrity and authenticity, particularly in fields where credibility is paramount, such as journalism or documentary film-making. Even subtle remnants can create a sense of uncertainty about the original source or authenticity of the video content.
Additionally, the occurrence of ghosting appears to differ based on the video content itself. Videos with fast-paced action or rich visual detail might be more susceptible to ghosting because of their complex visual textures. This indicates that the adaptability of algorithms might need further development to handle this variety.
The ongoing challenge of watermark ghosting emphasizes the importance of future research focused on enhancing the learning and adaptive capabilities of AI algorithms. Exploring hybrid approaches that combine the best aspects of AI with traditional image processing techniques could be fruitful in achieving truly comprehensive watermark removal. As content creators continually devise new watermarking methods, AI models will require continued refinement to effectively counteract them.
In essence, while AI's ability to identify and remove watermarks has dramatically improved, the 23% persistence rate of watermark ghosting underscores the need for ongoing innovation. Addressing this issue requires a combination of algorithmic advancements, further exploration of hybrid techniques, and an understanding of the limitations of AI in handling complex visual data. This will allow for even more seamless video processing and maintain user expectations for polished, watermark-free content.
7 Facts About AI-Driven Video Watermark Removal in 2024 From Algorithms to Size Limitations - Auto Frame Analysis Now Handles Motion Graphics At 60 FPS
AI-powered video processing is increasingly capable of handling complex visual elements, including motion graphics, at higher frame rates. Specifically, auto frame analysis systems now efficiently process motion graphics at 60 frames per second (FPS). This is a notable improvement, particularly for videos with a lot of movement, where a smoother frame rate significantly improves the viewing experience.
The enhanced capabilities likely stem from the use of AI-optimized algorithms. These advancements not only help increase the frame rate but can also reduce delays or latency, leading to more fluid and responsive video playback. Interestingly, this new level of performance doesn't seem to require specialized hardware, suggesting broader compatibility across different systems. This wider compatibility is likely important to make the technology more accessible to a broader range of users.
The improved handling of motion graphics at 60 FPS is a positive development in video processing. As we demand smoother and more engaging visual experiences, this ability to manage intricate, high-speed content becomes essential for a higher-quality viewing experience. It will be interesting to see how this advancement is integrated into various applications, and whether it leads to further improvements in video technology.
Auto frame analysis has seen a notable enhancement, particularly in its ability to handle motion graphics at 60 frames per second (FPS). This represents a significant leap forward, as previous systems often struggled with lower frame rates. The increased fluidity at 60 FPS contributes to a smoother viewing experience, especially for scenes with a lot of motion.
However, the increased processing capabilities have introduced new considerations. The algorithms now enable real-time processing, allowing for on-the-fly analysis and application of motion graphics. While this has the potential to revolutionize live editing environments, its impact on areas like live broadcasting or video gaming still requires further exploration. Interestingly, the enhanced frame analysis has also led to improved depth perception capabilities. This is crucial in ensuring layered graphics seamlessly blend into the background, preventing visual inconsistencies.
Moreover, the system can now manage more intricate animations that go beyond basic graphics. This includes particle effects and dynamic text, crucial features in sectors like advertising or professional video production where engaging visuals are paramount. It's interesting to note that the algorithms are now using machine learning techniques, allowing the system to adapt and optimize based on the types of graphics encountered. This adaptability might lead to more efficient processing over time, as the system "learns" and adjusts to specific preferences or styles.
The potential benefits of auto frame analysis extend to a reduction in post-processing time, with estimates suggesting a decrease of up to 50%. This is particularly useful for projects with tight deadlines. Furthermore, the system has been designed to work seamlessly with legacy video formats, offering a benefit to content creators who wish to enhance older footage with modern graphics.
While the improvements are significant, there are still challenges. In certain situations, particularly those with low-light or high-contrast conditions, the auto frame analysis can misinterpret scenes. This emphasizes the need for continued system refinement to minimize processing errors and ensure satisfactory outcomes. Even with the increased power, there's a potential for visual artifacts, especially during quick scene changes or complicated motion sequences. These occasional artifacts are a reminder of the technology's limitations, which editors need to consider when setting expectations.
Lastly, the jump to 60 FPS and detailed motion graphics has resulted in a noticeable increase in computational demands. Users might need to consider hardware upgrades to ensure optimal performance in demanding editing scenarios. It's a trade-off that users need to be aware of when planning projects that rely on this technology. In conclusion, while auto frame analysis shows great promise, further refinements are needed to fully realize its potential and address its limitations.
7 Facts About AI-Driven Video Watermark Removal in 2024 From Algorithms to Size Limitations - Machine Learning Models Require 15GB Storage For Basic Operations
AI-driven video watermark removal, like many machine learning applications, relies on models that require substantial storage. A basic operational setup for these models often demands around 15 gigabytes of storage. This requirement can fluctuate based on the complexity of the model itself and the size of the data it processes. The need for fast data access during both training and application of the model necessitates efficient storage solutions, especially as AI models become more sophisticated. This is especially relevant for watermark removal, where maintaining video quality during processing is crucial and larger files might be involved. The ongoing drive towards more complex AI models leads to a corresponding increase in storage requirements and emphasizes the crucial role of efficient infrastructure for effectively managing and manipulating video files, balancing both storage capacity and processing speed. The practicality of handling large files within these limitations remains a continuous challenge in the field.
### The Hidden Storage Costs of Machine Learning Models
While we often focus on the impressive feats of AI, like watermark removal in mere seconds, the reality of implementing these models comes with some surprising storage implications. For example, a basic machine learning model might require anywhere from 500MB to 20GB of storage, with 15GB being a commonly cited starting point. However, this is often just the tip of the iceberg. More complex models, particularly those built with advanced techniques and multiple layers, can far exceed this baseline.
The number of parameters within a model directly impacts its size. Models with millions of parameters, especially those undergoing frequent retraining or updates, will inevitably consume significantly more storage over time. Managing both model size and the associated training data becomes crucial to avoid uncontrolled growth.
It's also important to consider the model's memory footprint during operation. A 15GB model, when in use, might actually require twice that amount in active memory, highlighting the need for robust hardware to maintain performance. This isn't limited to inference (using the model) – during the crucial training and evaluation phases, temporary files used for checkpoints and intermediate results can further inflate the required storage capacity, sometimes tripling the initial model size.
There's hope in the form of compression techniques. Methods like pruning (reducing unnecessary model complexity) or quantization (lowering the precision of numbers in the model) can potentially shrink the size of a model by 50% or more. However, this brings its own set of challenges that researchers need to understand.
Interestingly, the sheer volume and complexity of the training data influences model size indirectly. Larger, more sophisticated datasets frequently require more complex models, increasing storage demands.
Moreover, deploying a model for real-world use adds another dimension to storage requirements. Scaling a model across multiple server nodes for increased capacity and accessibility further drives up storage needs, potentially creating a massive increase from the original 15GB depending on the user demand.
Model versioning is another hidden cost. Organizations often retain multiple model versions during development and iterative improvements, further exacerbating the issue.
Adding to the complexity, models in cloud environments introduce more storage overhead due to dependencies, libraries, and configurations required for effective operation. This means that a simple 15GB model might actually need closer to 25GB of cloud space to function properly.
Data governance and compliance further contribute to hidden storage demands. Maintaining multiple copies for auditing and regulatory purposes adds yet another layer of storage requirements that can easily exceed the initial model size many times over.
These factors paint a complex picture of machine learning model storage needs. It becomes clear that estimating model storage is more than just stating a raw file size. It requires careful consideration of model complexity, training data, deployment environment, and various operational needs. It reminds us that there are several subtle yet impactful complexities when it comes to AI implementation.
Experience error-free AI audio transcription that's faster and cheaper than human transcription and includes speaker recognition by default! (Get started for free)
More Posts from transcribethis.io: