Experience error-free AI audio transcription that's faster and cheaper than human transcription and includes speaker recognition by default! (Get started for free)
7 Critical Steps to Pass Rev's 2024 Transcription Test A Data-Driven Analysis
7 Critical Steps to Pass Rev's 2024 Transcription Test A Data-Driven Analysis - New Grammar Guidelines Focus on Verbatim Rules Over Clean Verbatim
Recent changes in grammar guidelines have altered the landscape of transcription, prioritizing verbatim accuracy over the previously popular clean verbatim style. This shift demands transcribers to meticulously capture every uttered word, including hesitations, fillers, and other speech imperfections. This increased emphasis on detail might make preparing for Rev's 2024 transcription test more challenging, as a thorough understanding of these new rules is crucial for success. The move towards a stricter verbatim approach could create a situation where different transcription service providers adopt slightly varying interpretations of these guidelines. This potential for inconsistency underscores the importance of carefully studying the updated guidelines to ensure transcribers meet the evolving expectations of the field and ace upcoming tests.
It seems the new transcription guidelines are pushing for a more rigorous adherence to verbatim transcription, prioritizing capturing every spoken word and sound. This shift emphasizes that even slight alterations, previously commonplace in "clean verbatim" styles, can potentially alter the original meaning or intent.
Linguistic research suggests that individual transcribers' familiarity with specific dialects can lead to inconsistencies when applying "clean verbatim" principles. By demanding strict adherence to what's actually spoken, these new rules might mitigate this bias. Studies also highlight verbatim's role in preserving subtle cues like hesitations and pauses, leading to richer data for qualitative research.
This trend towards verbatim reflects a growing awareness that the nuances of spoken language often carry important information, going beyond the literal words. This focus is beneficial for automated systems, as machine learning models trained on precise transcriptions become more capable of understanding speech patterns.
Furthermore, the intricacies of sentence structure and how humans interpret language suggest that accurate, verbatim transcripts are crucial for maintaining the speaker's original message. By minimizing the "cleaning up" of language, we can avoid unintentionally introducing bias or distortion, especially when dealing with complex or sensitive topics. This is especially important in fields like law and medicine, where misinterpretations can have severe consequences.
Finally, verbatim transcription's importance becomes clear in situations involving multiple languages. Even seemingly small differences in word choices or context can change the meaning dramatically, highlighting the need for utmost precision. The guidelines, therefore, seem to urge transcribers to develop a more comprehensive understanding of speech, not just the words themselves, but also the underlying rhythm and emotional elements often overlooked by "clean verbatim" approaches, thus creating transcripts with more depth and accuracy.
7 Critical Steps to Pass Rev's 2024 Transcription Test A Data-Driven Analysis - Raw Audio Test Now Features Regional US Accents From Texas to Maine
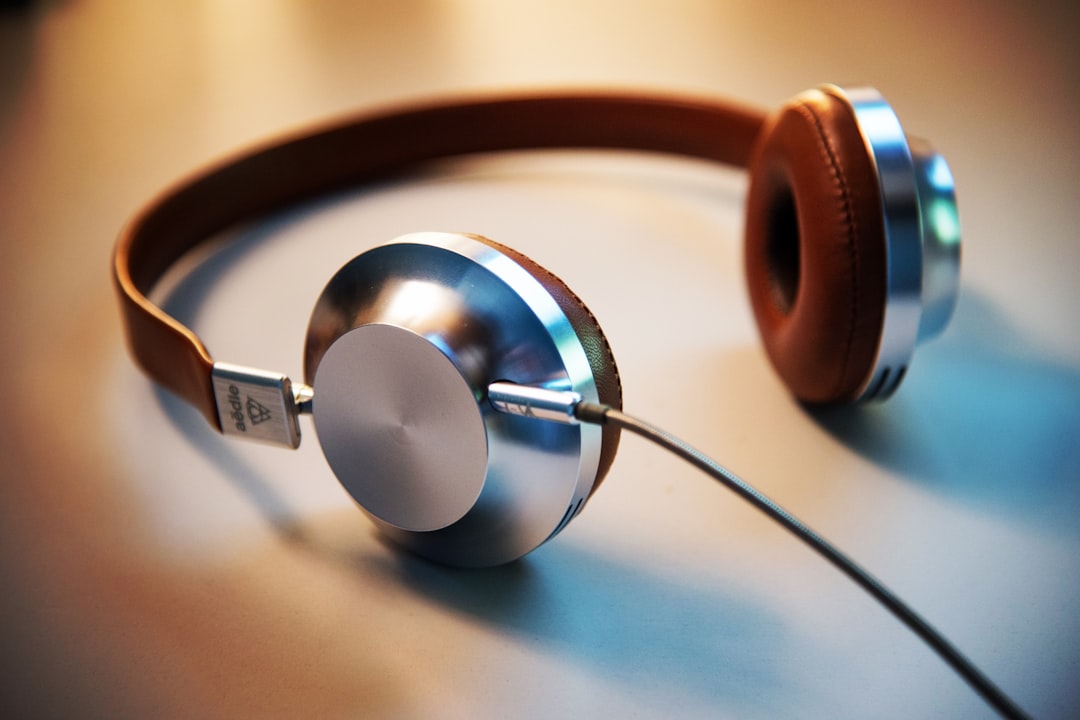
Rev's 2024 Transcription Test now incorporates a wider range of American accents, from the Southern drawl of Texas to the distinct speech patterns of Maine. This means that the test audio now includes a more diverse set of dialects, pushing transcribers to sharpen their skills in accurately capturing various speech patterns. This change is significant because it recognizes the vast linguistic landscape of the US and highlights the importance of understanding diverse dialects in order to create accurate verbatim transcriptions. However, this shift also presents a challenge, as some accents, like those found in Minnesota, are notoriously difficult for language recognition systems to decipher. Transcribers, therefore, need to be more adept at recognizing and capturing the subtle sounds that define these regional dialects. The inclusion of such accents likely reflects a growing movement towards inclusivity and an appreciation for the rich diversity of American English. At the same time, it presents a hurdle for transcription professionals, as the test now necessitates a heightened ability to translate these diverse sounds into accurate written formats.
Rev's 2024 transcription test now includes a wider range of US accents, spanning from the Texas twang to the Maine dialect. This expansion reflects the reality of diverse speech patterns across the country. It seems like a good idea to ensure transcribers can handle the nuances of regional language.
However, capturing these different accents presents new challenges. There are specific pronunciation differences, like vowel shifts and changes in consonants. For instance, the "Northern Cities Vowel Shift" is a distinct pattern that alters how some words are spoken, potentially making them trickier to transcribe accurately.
Beyond pronunciation, each region has its own vocabulary and expressions. Words common in one area might be unknown elsewhere. This means transcribers need to be familiar with a wide array of colloquialisms to avoid errors.
It's not just about geography though. Social groups also have distinct speech patterns based on factors like socioeconomic class or ethnicity. Understanding these variations, called "sociolects," is important for capturing the speaker's full context.
Interestingly, this shift towards including more accents is relevant for AI transcription. For machine learning systems to accurately transcribe speech, they need to be trained on a wide array of accents. This helps these systems recognize and interpret language across a variety of backgrounds.
One potential problem is that words that sound similar can have different meanings depending on where you are. These regional variations can easily lead to mistranscriptions if not understood correctly. Accuracy really hinges on correctly interpreting the context of the words spoken.
Accent also impacts the way people speak – not just the words, but the rhythm and pace. Some regions are characterized by longer pauses which transcribers should note. Such subtle aspects of speech can carry important meaning, and accurately representing them is crucial.
Culturally specific references or idioms, often linked to an accent, can further complicate the process. Transcribers must be sensitive to this, ensuring they capture the cultural meaning.
With the surge in online communication, like video conferencing, we're being exposed to a greater range of accents. This leads to subtle shifts in how people speak, constantly evolving regional pronunciations and vocabulary. Transcribers need to keep up with this shifting landscape.
Ultimately, this understanding of regional speech goes beyond transcription accuracy. It has real-world applications for fields like marketing and customer service. In these areas, recognizing and adapting to variations in language is key for effective communication, fostering customer engagement and satisfaction.
7 Critical Steps to Pass Rev's 2024 Transcription Test A Data-Driven Analysis - Timestamping Requirements Changed to 30 Second Intervals
Rev's transcription test has introduced a change to timestamping, now requiring 30-second intervals instead of the previous 2, 5, or 10-minute markers. This means transcribers must now insert time codes every 30 seconds, creating a much more granular link between the audio and the written transcript. The rationale behind this shift is likely a heightened focus on precision and the need for readers to easily pinpoint specific sections within audio files. This approach strengthens the integrity and reliability of transcripts, making them more valuable for diverse uses like preserving the authenticity of digital documents.
While the increased frequency of timestamping may add a layer of complexity to the transcription process, the goal is ultimately to create more useful and reliable transcripts. This change may require some adaptation and practice for transcribers accustomed to the previous system, ensuring they can maintain accuracy while meeting the new requirements. However, the move towards more frequent timestamping underscores a growing emphasis on detail and data integrity in the field of transcription.
1. **The 30-Second Timestamp Shift**: Rev's decision to mandate 30-second intervals for timestamps represents a notable change in the transcription landscape. It effectively moves away from a more paragraph-focused approach towards a finer-grained, time-based segmentation of audio. This shift necessitates a different way of thinking about how dialogue unfolds within these 30-second chunks, potentially altering the standard transcription workflow.
2. **Efficiency Concerns and Timestamp Insertion**: While the goal of timestamping is undoubtedly to improve the usability of transcripts, the new 30-second rule might inadvertently slow down the transcription process. Frequent timestamp insertions could become a distraction, potentially interfering with a transcriber's focus on ensuring clarity and capturing the broader contextual meaning of the audio.
3. **The Evolving Role of Timestamps**: Timestamping's journey has been one of continuous evolution. Originally designed for video editing and production, its integration into transcription services reflects how the field has constantly adapted to technological innovations.
4. **Challenges for Voice Recognition Systems**: The transition to 30-second intervals presents a new set of challenges for voice recognition software. These systems typically perform best when processing continuous speech. The more fragmented nature of transcriptions with more frequent timestamping might negatively impact their accuracy. This highlights the ongoing need for researchers and engineers to improve the capabilities of AI in handling such segmented data.
5. **Cognitive Load and Transcriber Fatigue**: The implementation of stricter timestamping standards might place a greater cognitive burden on transcribers. Juggling the simultaneous tasks of ensuring accuracy in transcription and adhering to precise timing could increase the chances of fatigue or burnout. This in turn could impact both job satisfaction and the overall quality of the final transcript.
6. **Timestamping as a Tool for Deeper Analysis**: Beyond its core purpose of marking time, the frequent 30-second timestamping might actually be beneficial for more in-depth qualitative analysis. Researchers could leverage these timestamps to more readily analyze correlations between changes in dialogue and shifts in topics or emotional tone, potentially making transcriptions more valuable for uncovering insights.
7. **Importance in Legal and Medical Domains**: In areas like law and medicine, accurate timestamping holds immense value. The precise chronological record of dialogue that timestamps provide becomes crucial in legal proceedings or medical reviews. The exact timing of statements can have legal weight, making timestamp accuracy a critical aspect of maintaining integrity and accountability.
8. **Dialect Challenges in Shorter Segments**: The shift to 30-second intervals requires transcribers to be hyper-aware of subtle dialectal variations within those segments. The shorter time frames could cause nuanced phonetic features or regionally specific expressions to be missed or misrepresented if not carefully transcribed.
9. **The Risk of Contextual Misinterpretations**: When dialogue is broken into smaller segments via frequent timestamps, there's an increased risk of misinterpreting the context of what is being said. Shorter, less cohesive sections of dialogue might make it difficult for a transcriber to grasp a speaker's full intended meaning. This is especially important in cases where the transcription relates to complex or sensitive topics.
10. **Towards New Standards in Transcription**: The adoption of more frequent timestamping could signify the development of new standards in transcription practices. The industry may see the emergence of benchmark criteria related to both timing and content accuracy. These standards could influence the way transcribers are trained and shape the expectations of the field at a global level.
7 Critical Steps to Pass Rev's 2024 Transcription Test A Data-Driven Analysis - Rev Introduces Background Noise Rating System for Audio Files
Rev has implemented a new system for rating background noise in audio files. This system aims to help transcribers gauge the level of noise in audio they receive. Understanding this noise level is important because it can impact the accuracy of a transcription, especially as Rev emphasizes verbatim transcription and a wider variety of accents in the 2024 transcription test. This new noise rating system is a step towards improving transcription accuracy overall. However, it's still crucial for transcribers to carefully select audio files with minimal background noise to improve their success rate on the test. The transcription landscape at Rev is consistently evolving, and choosing audio wisely remains a key factor for anyone looking to pass their test.
Rev has introduced a new system for rating the background noise in audio files. This change marks a significant shift in how audio quality is assessed, moving from a more subjective approach to a more quantifiable method. By assigning a rating to the noise level present in each audio file, transcribers can now better anticipate potential challenges and adjust their strategies accordingly.
It's known that background noise can make it hard to understand what's being said in a recording, particularly when there are other sounds overlapping with speech. Rev's new system acknowledges this and aims to help avoid mistakes that might occur due to distracting noise.
Research in sound and speech understanding shows that it becomes much harder to understand speech when there's noise in the background, especially if it's louder than a typical conversation. This new noise rating system can guide transcribers towards selecting audio that's easier to work with, which should improve their transcription accuracy.
It's interesting to think about how this new system could be incorporated into AI transcription. For example, training AI systems on audio with different noise ratings could help them get better at filtering out noise and understanding speech in a variety of conditions. This could lead to more efficient and accurate transcriptions in the future.
This rating system has the potential to bring some standardization to audio quality assessment within the field of transcription. This means we could see more consistency in how audio quality is evaluated across different platforms and services, which could lead to more reliable transcripts.
We can also expect this new system to impact how future transcribers are trained. Training programs might need to place more emphasis on teaching techniques for dealing with different levels of background noise while maintaining accurate transcriptions.
Of course, one challenge with any noise rating system is that what's considered "acceptable" noise can be different for each person. It'll be interesting to see how effective this new system is in overcoming this inherent subjectivity.
It's also plausible that this new system could help transcribers be more productive. With a clear noise rating, transcribers could choose to prioritize tasks with clearer audio or decline those with excessive noise, making their workflow more efficient.
It's also important to consider how this system could be useful beyond transcription. The concept of evaluating and categorizing background noise has applications in fields like audio editing, sound production, and any area where clarity of speech is critical.
Ultimately, the introduction of this noise rating system points to ongoing advancements in audio technology. We're seeing improvements in microphone design, noise cancellation software, and techniques for enhancing audio quality. This rating system is another step in this process and could lead to even higher-quality audio and significantly better transcriptions in the future.
7 Critical Steps to Pass Rev's 2024 Transcription Test A Data-Driven Analysis - Quality Metrics Updated to Include Speaker Label Accuracy
Rev's latest update to its transcription quality standards now includes speaker label accuracy as a key metric. This signifies a growing emphasis on ensuring that the words spoken are correctly linked to the individuals who said them. This is especially important when knowing who is speaking adds to the meaning of the transcript. While speaker identification is important, other factors like overall transcription accuracy, completeness, and consistency continue to matter. Businesses that use transcription services are being urged to track and improve quality through the use of various metrics. This move highlights the field's increasing demand for detail and precision in transcriptions. It's a sign that the industry is striving for higher quality in all aspects of the transcription process, which is crucial for maintaining accuracy and reliability.
Rev's updated quality metrics now include speaker label accuracy, which offers a more structured way of evaluating the quality of transcriptions. This means that we can now measure how often a transcriber mistakenly attributes dialogue to the wrong speaker. This new metric should help us pinpoint areas where transcribers might need to hone their skills in recognizing cues that indicate who's speaking.
It appears that ensuring accurate speaker labels is closely linked to improving the overall quality of transcripts, especially when multiple individuals are talking. If we consistently assign dialogue to the right person, it reduces the potential for confusion and misinterpretation. This is really important in fields like law and medicine, where the meaning of what's said is crucial.
There's been progress in developing automated systems that can help identify speakers based on their voice characteristics. While these systems can be useful, they still haven't quite caught up to the level of detail that trained transcribers can pick up. Humans are better at understanding the more subtle nuances in people's voices.
Keeping track of who is speaking while simultaneously transcribing everything they say seems to add a bit more complexity for transcribers. It requires a different kind of focus and could possibly contribute to transcriber fatigue, especially if they have to maintain a high level of accuracy for longer periods.
The need for accurate speaker labels becomes more significant when transcripts are shared with different groups of people. If a transcriber mixes up who said what, there's a risk of causing misinterpretations. This is a point to consider in educational and professional settings, where clear attribution of statements is important.
People have a range of vocal characteristics depending on their background, making speaker identification sometimes challenging. Transcribers should be trained to recognize these variations in speech, ensuring accurate labels while avoiding any biases that might stem from limited experience with diverse speakers.
When it comes to legal situations, incorrectly assigning dialogue to a person can have major implications, possibly influencing the outcome of a case. This emphasizes the vital role of precise speaker labels, as understanding who said what can heavily impact how conversations are understood.
Poor audio quality and background noise can have a negative impact on how accurately we can determine who's speaking. Ensuring that the audio is clear is important, as it helps avoid making errors when trying to correctly identify speakers.
It's not just about figuring out who is speaking, but also understanding the feeling or emotion behind their words. This emotional subtext can provide valuable context that enriches the transcript, helping those listening to better understand the subtle nuances within the conversation.
Efforts to improve speaker label accuracy are driving a shift towards integrating more machine learning into transcription. By training systems on large datasets that focus on speaker identification, we can hope to create models that are more proficient in processing speech. This could lead to breakthroughs in how we transcribe speech in the future.
7 Critical Steps to Pass Rev's 2024 Transcription Test A Data-Driven Analysis - Audio Preview Length Extended From 30 to 45 Seconds
Rev has increased the length of the audio preview available for their transcription tests, extending it from 30 to 45 seconds. This change aims to improve the user experience by giving potential transcribers a longer sample of the audio to evaluate before committing to the full transcription. A longer preview can provide a better sense of the overall context and nuances of the recording, which is valuable for making informed decisions about whether to accept a specific transcription job.
However, any change in workflow can present new challenges. While the longer preview could potentially be helpful, it also introduces the risk of becoming more easily distracted or finding it harder to focus on specific details critical for transcription success. This extended audio preview is one of several changes implemented by Rev to refine their testing processes and push for higher quality and efficiency within their transcription service. While helpful in some ways, transcribers should be prepared for the potential downsides of a longer preview.
1. **Impact on Workflow**: Extending the audio preview from 30 to 45 seconds could potentially alter the typical workflow for transcribers. It might require a shift in how they approach the initial assessment of the audio, potentially increasing the time needed to familiarize themselves with the content.
2. **Enhanced Contextual Understanding**: The added 15 seconds offer a chance for transcribers to gain a richer understanding of the context within the audio. This could prove helpful in capturing subtle nuances in speech, like changes in tone and emotional cues, which are sometimes missed in shorter snippets.
3. **Implications for AI Training**: Longer audio previews could be a valuable resource for training machine learning models involved in automated transcription. By exposing these models to more extensive audio samples, they could potentially become more adept at recognizing diverse speech patterns and dialects.
4. **Human Attention Span**: It's worth considering the potential impact on human attention. Research suggests that most people can effectively focus for around 20-30 seconds. A 45-second preview might be at the edge of that optimal range, potentially leading to a decline in focus towards the end of the preview.
5. **Capturing Diverse Speech**: Longer clips could inherently encompass a greater variety of speech styles and dialectal variations. This offers transcribers more opportunities to practice recognizing and interpreting various accents or regional speech patterns, ultimately refining their transcription skills.
6. **Risk of Misinterpretation**: With increased audio length, the potential for misinterpreting the context of the spoken content also increases. Maintaining a clear understanding of the conversational flow within longer excerpts might be a greater challenge for transcribers, possibly leading to inaccuracies.
7. **Cognitive Demand**: Processing longer audio previews demands more from transcribers cognitively. This could potentially result in quicker fatigue, which might impact the consistency of their transcriptions and their overall performance.
8. **Importance in Specific Domains**: In fields that demand extreme accuracy, like legal or medical transcription, the longer audio preview is crucial. It allows transcribers to gather a more thorough understanding of the context surrounding key statements, helping them make more informed decisions about the importance of those statements in the larger conversation.
9. **Emotional Nuances**: The additional seconds in the preview allow transcribers to better grasp the emotional tone of the speaker, which can play a vital role in correctly interpreting the intent behind the words. This is particularly significant in scenarios where emotional context heavily influences the overall meaning of the discussion.
10. **Adapting Training Programs**: With the shift to a longer audio preview, training materials for new transcribers should be adjusted. There should be more emphasis on strategies for effectively handling longer audio segments, ensuring they can maintain accuracy and a deep understanding of the content while working with the extended previews.
7 Critical Steps to Pass Rev's 2024 Transcription Test A Data-Driven Analysis - Style Guide Updates Target Medical and Legal Terminology
Rev has recently updated its Style Guide to pay closer attention to how medical and legal terms are handled in transcriptions. This reflects a growing need for highly accurate and clear transcripts, especially in areas like healthcare and law where small mistakes can have big impacts. The changes are designed to make sure transcripts are more accurate, especially when dealing with specialized language.
Transcribers are being told to keep up with any new rules in legal and medical terminology, highlighting the importance of knowing the specific language used in these fields. This renewed focus on accuracy and detail shows a wider trend towards improving the overall standards of transcription, particularly for professionals dealing with complex topics. These guide updates aim to improve the quality of transcripts and meet the demands of a wide range of clients who require precise information in their transcriptions. In short, these changes help ensure that transcripts are as accurate and reliable as possible.
Rev's style guide updates, particularly those concerning medical and legal language, reveal a growing emphasis on precision and accuracy in transcription. It seems they recognize that mistakes in these fields can have significant consequences, like misdiagnosis in healthcare or misrepresentation in legal proceedings.
This push for accuracy means transcribers need to constantly update their knowledge. Medical and legal terminology is constantly evolving, and staying current with the latest terms and practices is now a crucial aspect of the job. Simply knowing a term isn't enough; they must also grasp the nuances of its usage within a given context. Similar terms can have wildly different meanings depending on the surrounding conversation.
The result is a higher demand for transcribers with specialized expertise. It's reasonable to think that relying on professionals who understand the intricacies of these domains can mitigate the risks associated with errors in transcription. And, as machine learning models increasingly process these types of transcripts, clear and consistent use of terminology becomes even more crucial for improving AI's ability to handle these complex areas.
One interesting outcome could be improvements in communication within the healthcare sector. If transcription consistently uses standardized medical language, it could improve clarity for doctors, nurses, administrators, and patients alike.
However, this increased focus on accuracy might also lead to a more demanding job for transcribers. It could mean spending more time checking terminology against evolving standards. The legal field adds another layer of complexity: language can vary significantly depending on the jurisdiction. This creates a need for transcribers to be familiar with regionally specific terms to avoid errors in legal transcriptions.
There's a potential drawback though: a focus on extreme precision might hinder a transcriber's ability to capture the subtle meaning intended by a speaker. Finding a balance between standardization and capturing the essence of the speaker's voice becomes a challenge.
These updates indicate that a strong foundation in both medical and legal concepts is becoming a necessity. Transcribers are increasingly expected to work across disciplines, meaning they'll need interdisciplinary training to handle the increasing complexities of these kinds of transcripts. It seems like the transcription field is evolving to become more specialized, placing more importance on context and potentially requiring a deeper understanding of the subject matter being transcribed, beyond simply recording words.
Experience error-free AI audio transcription that's faster and cheaper than human transcription and includes speaker recognition by default! (Get started for free)
More Posts from transcribethis.io: