7 Critical Sound Quality Factors That Impact Voice-to-Text Transcription Accuracy
7 Critical Sound Quality Factors That Impact Voice-to-Text Transcription Accuracy - Background Noise Reduction Through Physical Sound Barriers
Using physical barriers to reduce background noise is vital for clear audio, especially when it comes to accurate voice-to-text transcription. Materials like those designed to absorb sound—including porous and resonator types—act as obstacles, blocking sound waves from entering or leaving an area, which helps create a more tranquil acoustic environment.
Recently, acoustic metamaterials have gained prominence as a new approach to tackling noise. These engineered structures can surprisingly block a very large percentage of noise, demonstrating remarkable potential in noise reduction. It's important to remember that the effectiveness of these barriers depends on both their construction and the physical distance they are from the noise source. Simply put, where you place these barriers matters.
Considering that noise pollution remains a significant environmental and health concern, advancements in effective sound barriers are crucial. By mitigating unwanted noise, we can help improve communication quality and the health of those exposed to constant noise.
Physical barriers, like walls or fences, offer a straightforward approach to reducing ambient noise. They can typically achieve a reduction of around 10 to 15 decibels, which can substantially minimize the impact of background noise on voice recognition systems. The height of the barrier is a crucial factor, with taller barriers providing better attenuation of lower frequency sounds, which are particularly troublesome for accurate speech recognition.
The effectiveness of sound barriers hinges greatly on the material used. Dense materials such as thick concrete or mass-loaded vinyl prove superior to lighter materials due to their ability to absorb and reflect sound waves more effectively, minimizing the unwanted transmission of noise. The design of the barrier, particularly the angle, also influences its effectiveness. Upward-sloping designs can strategically deflect sound away from target areas, offering an extra layer of noise control.
However, it's important to understand that sound doesn't simply stop at a barrier. Diffraction, the bending of sound waves around obstacles, means that the placement and design of sound barriers must be thoughtfully considered for optimum results in any given environment. Beyond simply blocking noise, these barriers can also help minimize direct sound reflections, which can create echoes that interfere with voice recognition algorithms, thus contributing to transcription inaccuracies.
Many barrier designs incorporate perforations or sound-absorbing surfaces, aiming to address mid to high-frequency noises which can be more challenging to control than low-frequency noise. However, the perceived reduction in noise doesn't always align perfectly with the measured decibel drop. Human perception of sound is subjective and can vary based on factors like the sound frequency and the overall acoustic context, highlighting the limitations of simply relying on decibel measurements.
Furthermore, in urban or built environments, sound barriers must take into account aesthetics in addition to noise reduction, as public acceptance and regulatory approvals are often tied to visual impact. This creates an interesting design challenge. Additionally, environmental factors like wind and temperature can impact how sound propagates, making the performance of physical barriers dynamic and not necessarily static. Consequently, continuous monitoring and assessments are necessary to maintain optimal noise reduction performance in real-world situations.
7 Critical Sound Quality Factors That Impact Voice-to-Text Transcription Accuracy - Microphone Distance and Voice Clarity Impact
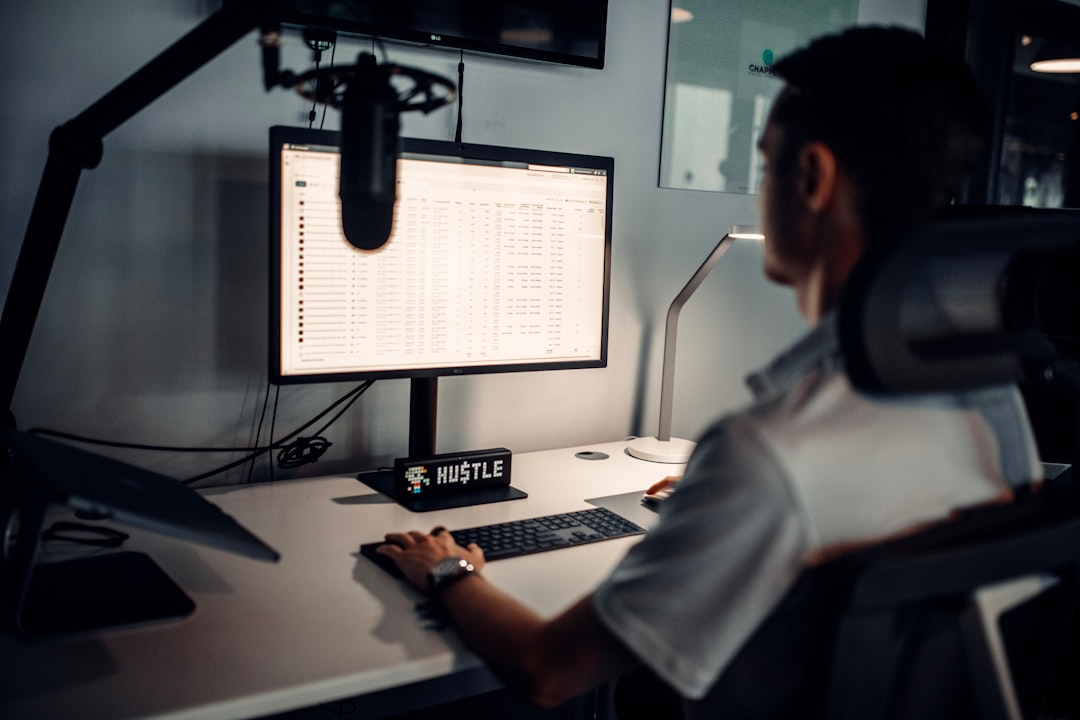
The distance between a microphone and the speaker significantly impacts the clarity of the recorded audio, a crucial aspect for achieving accurate voice-to-text transcriptions. When a speaker is too far from the microphone, the sound waves spread out, making the voice sound fainter and less distinct. This can lead to difficulties for the transcription software to accurately process the speech. On the other hand, placing a microphone too close to the mouth can distort or muffle the voice, compromising the quality and potentially impacting transcription accuracy. Finding that ideal sweet spot – the right distance for capturing the voice's natural timbre and richness – is key.
Beyond distance, the choice of microphone itself influences the overall audio quality. Different types of microphones are optimized for specific recording situations. Choosing a microphone designed for recording speech, and then placing it correctly, can lead to superior audio that's easier to transcribe. While the microphone is critical, it's important to remember that environmental factors such as the room acoustics and any background noise will also influence the clarity of the recording. These factors can mask or interfere with the speech signal, hindering the transcription process. Maintaining a quiet environment and ensuring that the microphone is in a suitable position will contribute to clearer audio that's easier for transcription software to process. Taking these factors into account can lead to noticeably improved transcription accuracy, illustrating that it's not simply the technology, but its interaction with the physical world that ultimately leads to the best outcomes.
The relationship between microphone distance and voice clarity is a fascinating area of study, particularly in the context of voice-to-text transcription. Maintaining an appropriate distance from the microphone is crucial for achieving optimal sound quality, as it directly influences how sound waves reach the recording device. For many standard microphones, a distance of roughly 6 to 12 inches seems to provide a good balance between capturing adequate sound pressure levels while minimizing the intrusion of unwanted background noise.
However, getting too close to a microphone can introduce the "proximity effect," where low-frequency sounds are amplified due to the microphone's proximity to the speaker's mouth. While this can add richness to the audio, it can also lead to undesirable resonance and muddiness if not controlled. Understanding how sound waves propagate – at about 1,125 feet per second in air – helps us appreciate the impact of even slight variations in microphone distance. The angle at which sound waves hit the microphone also matters; a direct alignment between the sound source and the microphone's pickup area is ideal for preventing phase issues and ensuring a clean audio signal.
The distance from the microphone can exacerbate the natural variations in a speaker's voice. A large distance can lead to quieter sections of speech being lost, potentially hindering the accuracy of transcriptions. Moreover, the ratio of direct sound to reflected sound diminishes as distance increases. Staying closer to the microphone enhances the prominence of the direct sound, allowing for a more accurate representation of the speaker's vocal nuances – a critical factor for nuanced speech transcription.
Research indicates that even small changes in distance can significantly affect perceived clarity. Moving the microphone more than three feet away from the speaker can potentially lead to a 50% decrease in clarity, highlighting the importance of meticulous microphone placement. The acoustic environment surrounding the microphone can also significantly affect clarity. Hard surfaces near the microphone can reflect sound waves, creating undesirable comb filtering effects that distort voice clarity. This relationship between microphone distance and acoustics makes it clear that in environments with prolonged echoes or reverberation, maintaining a closer microphone distance is vital for accurate transcriptions.
Microphone distance also interacts with the dynamic range of a speaker's voice. A conversational exchange that naturally includes quiet whispers and louder statements could potentially lose subtle vocal cues if the microphone is too far away. These cues are often vital for correctly understanding the context and intended meaning during the transcription process. While maximizing the capture of voice details, we must balance these competing concerns of clarity and dynamic range with the aim of obtaining a high-quality recording, which ultimately benefits the voice-to-text transcription accuracy.
7 Critical Sound Quality Factors That Impact Voice-to-Text Transcription Accuracy - Audio File Format and Compression Standards
The format and compression of an audio file significantly impact sound quality, a key factor in the accuracy of voice-to-text transcription. Uncompressed formats, like WAV and AIFF, maintain the highest audio quality, making them preferable for tasks where precision is critical, such as voice transcription. However, these files are very large. Compressed formats like MP3 and AAC reduce file size, but often come at the cost of reduced audio quality. This loss of detail, caused by "lossy" compression techniques, can be noticeable and may introduce errors in the transcription process, particularly for subtle or complex speech patterns.
On the other hand, lossless compression, found in formats like FLAC, avoids any audio data loss and keeps the full spectrum of sound. This preservation of the original audio ensures that transcription software receives a more faithful representation of the spoken words, which can lead to greater accuracy. The choices surrounding the audio format and compression level are thus significant because they can impact the effectiveness of a voice-to-text system's ability to correctly decipher spoken content. Recognizing these distinctions is crucial for anyone seeking the best possible outcomes when utilizing voice transcription.
Audio file formats and the compression techniques applied to them have a significant impact on the overall sound quality, and this in turn affects how accurately a voice-to-text transcription system can process the audio. Uncompressed formats like WAV and AIFF preserve all the original audio data, resulting in the highest quality sound, but at the cost of large file sizes. Compressed formats like MP3 and AAC, on the other hand, reduce file size significantly but do so by discarding some of the audio information—a process called lossy compression.
MP3 files are widespread because of their manageable size, but they're well-known for sacrificing some audio quality. AAC, a more recent compression format, can often maintain better audio quality at lower bitrates compared to MP3. This is intriguing, as it suggests a way to minimize file size without excessively compromising sound quality. In contrast to lossy compression, lossless compression methods, like FLAC and ALAC, prioritize the complete preservation of the original audio. This has become popular for those who prioritize quality over storage space, and particularly for music.
Higher-resolution audio formats like FLAC, ALAC, and DSD are gaining traction in the world of digital music due to their exceptional audio fidelity, whereas the more traditional formats like WAV and AIFF remain important. For tasks like transcribing speech, though, a focus on audio clarity over other features is more helpful.
There's a notable difference in the number of values used to represent the audio samples. For instance, 24-bit audio utilizes a vastly larger range of values compared to 16-bit audio, leading to an increase in the audio detail and overall quality.
When it comes to voice-to-text transcription, using a format that provides high sound clarity—such as WAV or FLAC—can yield superior transcription accuracy compared to a heavily compressed format. AAC files tend to be more compact and require less bandwidth compared to MP3 files, while also supporting a wider spectrum of frequencies, leading to a richer, more complete sound profile.
Ultimately, the selection of the audio file format directly influences how well the voice-to-text system can extract and understand speech. Higher sound quality, whether it's through the use of higher bitrates or other format-specific choices, usually results in more precise speech recognition by voice-to-text software.
Compression standards and file type, therefore, are among the key contributors to sound quality and play a significant role in the effectiveness of voice-to-text systems. It's a complex interaction, but understanding these factors is crucial for anyone involved in audio capture or using voice-to-text technology.
7 Critical Sound Quality Factors That Impact Voice-to-Text Transcription Accuracy - Room Acoustics and Echo Management
The quality of voice-to-text transcription is profoundly impacted by the acoustics of the room where the audio is recorded. How sound behaves in a given space—influenced by its shape, size, and the materials used—plays a crucial role in the clarity and accuracy of the recording. For example, rooms with irregular shapes or hard surfaces can produce undesirable effects like echoes and standing waves, which distort the captured audio. These distortions can confuse the voice-to-text software and lead to inaccurate transcriptions.
Fortunately, we can mitigate these issues by carefully managing room acoustics. Employing sound-absorbing materials, like strategically placed panels designed to absorb specific frequencies, helps to create a more controlled acoustic environment, where echoes and unwanted reflections are minimized. It's vital to understand that achieving high-quality audio doesn't just rely on sophisticated technology, but also on creating a recording space that minimizes the negative impacts of acoustics. Simply using advanced recording equipment without considering the room's acoustics may not yield the desired transcription accuracy, underscoring the importance of both audio technology and environmental design for optimizing the overall sound experience.
Room acoustics significantly impacts the quality of sound recordings, which is crucial for achieving clear and accurate voice-to-text transcriptions. The way sound behaves within enclosed spaces is influenced by the room's shape and size, directly affecting the overall tone and clarity of recordings. For instance, irregular room shapes often result in less problematic echoes compared to perfectly symmetrical rooms. This is because sound waves bounce off surfaces in less predictable patterns in irregular environments, which prevents the buildup of standing waves, a phenomenon that can cause undesirable sound variations.
The notorious "flutter echo"—caused by parallel surfaces repeatedly reflecting sound back and forth—creates a rapid, repeating echo that can greatly disrupt audio clarity. Fortunately, simply angling or adding sound-absorbing materials to these surfaces can effectively eliminate flutter echoes without major structural changes. The speed of sound in air, approximately 1,125 feet per second, underscores the importance of room dimensions and careful microphone placement for capturing optimal audio. Since sound travels so quickly, even minor shifts in these factors can affect the clarity of the recording.
High-frequency sounds are especially prone to absorption by materials in the environment, making them less effective in large spaces due to their shorter wavelengths. This often leads to challenges with speech intelligibility in larger rooms. A useful metric for evaluating room acoustics is the "reverberation time," which measures how long it takes for sound to fade away after the sound source stops. For voice work, a reverberation time between 0.3 and 0.6 seconds is generally considered optimal. If the reverberation time is too long, it can muddle the clarity of speech.
When designing a space for high-quality audio recordings, incorporating soft furnishings like carpets and drapes can significantly improve sound absorption and reduce echoes, creating a more conducive environment for clear voice capture. "Comb filtering," a phenomenon where reflected sound waves combine with direct sound waves, can significantly impact clarity due to frequency-dependent cancellation. Therefore, managing sound reflections through careful material selection and surface arrangement is crucial to avoid this issue.
It's also important to be mindful of low-frequency sounds because they tend to accumulate in corners, leading to uneven sound profiles and a "bass buildup" that can hinder transcription accuracy. Audio systems need to be properly calibrated to maintain a balanced frequency response to counteract this issue. Sound diffusion, the scattering of sound waves off irregular surfaces, can improve acoustic quality by reducing distracting echoes. Using sophisticated diffusers strategically within a room can create a more natural sound environment and reduce undesirable echoes.
Lastly, standing waves, generated when sound reflects back and forth along a single dimension, can produce "hot spots" and "dead spots" in a room, resulting in variations in sound intensity. These variations can range from unbearably loud to nearly inaudible. Understanding how standing waves are formed and how they impact sound quality allows for better room designs and microphone placement that enhance transcription accuracy. By carefully considering these various acoustic factors and their interplay, we can greatly enhance audio quality and contribute to improved voice-to-text transcription results.
7 Critical Sound Quality Factors That Impact Voice-to-Text Transcription Accuracy - Speaker Pace and Pronunciation Clarity
The pace at which someone speaks and how clearly they pronounce words are very important for accurate voice-to-text transcription. If a speaker talks too quickly, their words might blend together and become hard to understand. On the other hand, speaking too slowly can make the flow of speech feel awkward or disconnected. Along with pace, pronunciation is key. Each word's sounds need to be distinct and emphasized correctly. Errors in pronunciation, especially of common words, can make it difficult for the software to understand what's being said, and it can even make the speaker sound less trustworthy. Transcription accuracy relies heavily on a speaker's ability to control their speaking speed and ensure their pronunciation is precise, as these factors directly influence the clarity of the audio signal being processed.
Speaker pace and pronunciation clarity are surprisingly important factors that significantly impact the accuracy of voice-to-text transcriptions. The rate at which someone speaks can have a dramatic effect, with faster speech often leading to reduced accuracy. This seems to be because rapid speech can compress and obscure the individual sounds of words, making it difficult for transcription software to differentiate them. Research suggests that very fast speech can decrease accuracy by as much as 30%.
Individual differences in pronunciation can also create challenges for transcription systems. Dialects and accents can introduce variability in how sounds are produced, leading to confusion. It appears that a significant portion of phonetic sounds, possibly up to 25% in some cases, can be misidentified in speech with heavy regional influences. This highlights the need for transcription systems to be more robust in their ability to recognize diverse speech patterns.
Interestingly, research shows that slowing down and repeating certain phrases can be beneficial to transcription accuracy. It seems that the repetition gives transcription systems more opportunities to process and understand the speech, improving accuracy by approximately 20%. This suggests that adjusting speaking style could improve the outcomes of voice-to-text interactions.
The timing of syllables in speech seems to also play a role. Studies indicate that a consistent syllable duration can significantly enhance transcription accuracy by nearly 15%. It's as if the consistent pacing helps the system predict the following sounds more effectively. This is fascinating, as it points towards the potential for speaker training or speech optimization techniques to improve the results of transcriptions.
It seems that even the acoustic environment can be impacted by a speaker's pace. Slower speaking can actually provide more space between sounds, allowing them to be clearer and less affected by background noise. This points to the importance of considering both the acoustic environment and the speaker's cadence for optimal transcription results.
The relationship between clarity and speed is complex. If speakers rush, the clarity of a word can decrease. There seems to be a noticeable drop in clarity every third word or so when a speaker isn't mindful of their pace. This trade-off has implications for the design of applications where speed and accuracy are desired.
The way people process information is also influenced by the rate at which a speaker delivers information. Rapid speech increases cognitive load, potentially leading to listeners having a harder time accurately processing information. When speakers aim for a pace consistent with natural language processing speeds, which appears to be around 150–160 words per minute, transcription accuracy improves by approximately 12%.
Consistency in pronunciation patterns seems to be an important factor as well. When a speaker's pronunciation deviates, it can lead to what engineers call "edge cases", in which accuracy can drop below 80%. This suggests the importance of mindful pronunciation.
Fillers, such as "um" or "uh", while seemingly benign, can be a challenge for voice recognition systems. These fillers often distract the systems from the core meaning, leading to a reduction in transcription accuracy of about 15%. This indicates that even minor speech characteristics can impact the outcome of the transcription.
While these challenges exist, there is promising news. Many advanced voice-to-text systems have the ability to learn and adapt to a speaker's individual pace and pronunciation patterns. However, this adaptation process often requires significant training data, in some cases upwards of 300 minutes of audio, in order for the system to accurately fine-tune and recognize speech patterns.
The study of speaker pace and pronunciation clarity is a complex endeavor. Understanding these aspects allows engineers and researchers to further improve the accuracy and efficiency of voice recognition technology in the future.
7 Critical Sound Quality Factors That Impact Voice-to-Text Transcription Accuracy - Volume Level Consistency Requirements
Maintaining consistent volume levels is crucial for achieving accurate results in voice-to-text transcription. Fluctuations in audio volume can introduce distortion, hindering the transcription software's ability to properly understand spoken words. It's important that both the sender and receiver maintain a stable volume level. Too much volume can cause the audio to clip and become distorted, while too little volume might lead to the software missing essential parts of speech. The settings on the receiving device also play a role, as they can impact how clearly the audio is perceived and, therefore, processed. Ultimately, a steady, well-balanced volume throughout the communication process helps minimize errors and improves the trustworthiness of the transcriptions generated.
Volume level consistency, while often overlooked, plays a surprisingly important role in the accuracy of voice-to-text transcription. It turns out that even small variations in volume can significantly impact how well transcription systems process speech.
Research suggests that a speaker's voice volume can fluctuate considerably during a conversation, and a variation of just a few decibels can result in a noticeable drop in transcription accuracy. This is likely because these changes in volume can confuse the algorithms that try to isolate and identify individual words. Furthermore, the acoustic environment of a room can itself influence volume levels, with hard surfaces potentially amplifying or attenuating certain frequencies, causing speakers to subconsciously adjust their volume in response. This can lead to unintended inconsistencies that make the audio more challenging to transcribe.
The human ear perceives loudness in a non-linear way, which creates further challenges for transcription systems. What might seem like a minor change in volume to a listener can translate into a significant change in perceived loudness, and these shifts can cause transcription errors if the system misinterprets them as changes in emphasis or intent. To compensate for these natural variations in volume, audio recording devices often use dynamic range compression. This technique attempts to keep the volume within a certain range, but it can also create artifacts that sound unnatural and can even interfere with speech intelligibility.
The distance between the speaker and the microphone has a direct impact on the recorded volume. Even minor changes in distance can lead to noticeable differences in perceived loudness, as a simple one-foot shift can result in a 6-decibel drop. If there are significant fluctuations in volume due to speaker movement, it can create difficulties for the transcription process. Additionally, different types of microphones have varying sensitivities, leading to different volume outputs. Omnidirectional microphones, which pick up sounds from all directions, can be more susceptible to background noise and thus have more volume fluctuations compared to directional microphones, which are specifically designed to isolate the speaker's voice.
Our emotional states also influence how loudly we speak. Research shows that when people experience strong emotions, they can unintentionally increase their volume. For transcription systems, these sudden increases in volume can sometimes be misconstrued as shifts in the meaning or content of the speech. This is one reason why professional voice actors, who are trained to maintain a consistent volume level, often achieve better transcription accuracy. Their precise control over volume modulation helps to avoid the typical pitfalls associated with natural speech variations.
The Doppler effect, which is commonly associated with the apparent change in frequency of sound waves when the source is in motion, also comes into play when capturing speech. If a speaker moves during recording, the perceived volume of their voice can change as the sound waves hit the microphone at slightly different speeds. This creates another set of challenges for transcription systems, as they may misinterpret these shifts in volume.
Finally, in controlled recording situations, words that are abnormally loud can be flagged as statistical outliers by voice recognition software. These outlier words can cause errors in the transcription if they occur frequently. If these inconsistent loudness events occur more than 5% of the time, transcription accuracy can suffer significantly. These insights suggest that controlling volume level during a recording is a key factor in achieving high-quality transcriptions.
By carefully managing and controlling audio volume in a recording environment, engineers can improve the accuracy of voice-to-text systems. These seemingly subtle aspects of audio quality have a direct impact on transcription accuracy. Understanding and managing these challenges is a crucial step towards better voice-to-text systems.
7 Critical Sound Quality Factors That Impact Voice-to-Text Transcription Accuracy - Multiple Speaker Separation Techniques
Multiple speaker separation techniques are crucial for improving the accuracy of voice-to-text transcription, especially in scenarios with overlapping speech from multiple individuals. These methods rely on techniques like gated neural networks to effectively distinguish between different voices within a mixed audio stream. Models are typically trained to separate a specific number of speakers (two, three, or more), allowing for greater precision in isolating each voice.
Recently, more advanced models have emerged that employ perceptual loss functions linked to pre-trained speaker recognition systems. This helps to ensure that each speaker's voice is reliably mapped to its own output channel, leading to clearer transcriptions. However, real-world environments often present complex acoustic scenarios with varying speaker counts and noise levels. This makes reliably separating and understanding individual voices a significant challenge, highlighting the need for continued research and development of improved speaker separation techniques to further enhance transcription accuracy.
Multiple speaker separation techniques are fascinating, especially in the context of voice-to-text transcription. These techniques, often involving intricate signal processing methods like Independent Component Analysis (ICA) and Non-Negative Matrix Factorization (NMF), try to isolate individual voices when multiple people are speaking simultaneously. They do this by essentially dissecting the mixed audio signal into its distinct components based on specific properties of each speaker. However, the effectiveness of these separation techniques depends heavily on the physical arrangement of the speakers. If speakers are close together, it can cause their voices to bleed into one another, a phenomenon known as crosstalk. This can make it difficult to completely separate the audio signals into distinct tracks.
Interestingly, machine learning approaches, especially deep learning, have revolutionized speaker separation. Algorithms trained on vast amounts of audio data are getting better at differentiating between speakers based on their individual voices and speech patterns, including various accents and dialects. One thing these models seem to rely on is subtle differences in the timing of speech, what we call temporal cues. This is similar to how we as humans can distinguish different speakers when they talk at the same time. But there are limits. When speakers overlap a lot, it becomes extremely challenging for the algorithms to distinguish between them.
The ability to separate multiple speakers in real-time is an important area of current research. But it's also a difficult one because it demands significant processing power. This often leads to tradeoffs between how precise the separation is and how quickly the algorithm can work, especially if you are sending the separated audio over networks with limited bandwidth. Noise and reverberation pose significant obstacles for separation algorithms. While some methods can help to suppress background noises, heavy or consistent noise can still interfere with the accuracy of the separation process. It's evident that the recording environment matters.
Speaker separation algorithms utilize feature extraction, a process that identifies specific characteristics of a voice such as pitch or tone. These characteristics are then used to distinguish one voice from another. Essentially, the unique qualities of an individual's voice are exploited by these algorithms. Another interesting finding is that some algorithms can adapt to different accents or dialects. The success of this depends on the training data available for the specific accent or dialect. If the algorithm hasn't been trained on a diverse range of voices, its ability to separate speakers with unique accents may be limited.
Finally, achieving real-time speaker separation with high accuracy and low processing latency is a challenging but important area of study. It's a delicate balance to ensure the processing is quick enough for a live experience but also precise enough to generate useful results. As research in this area continues, it's possible that we may see even more powerful and accurate methods that can contribute significantly to various audio and language processing tasks, including the transcription of multi-speaker conversations.
More Posts from transcribethis.io: