Experience error-free AI audio transcription that's faster and cheaper than human transcription and includes speaker recognition by default! (Get started for free)
7 Critical Factors Affecting AI Meeting Transcription Accuracy in Late 2024
7 Critical Factors Affecting AI Meeting Transcription Accuracy in Late 2024 - Ambient Sound Processing Quality Now Impacts Word Recognition by 32%
The ability of AI to accurately transcribe speech is becoming increasingly sensitive to the quality of ambient sound processing. New research shows that the clarity of the surrounding audio now influences word recognition by a notable 32%. This emphasizes the crucial role of clean audio in environments where AI transcription is used.
Interestingly, the implementation of more sophisticated noise reduction technologies, especially in controlled settings like classrooms, is demonstrably improving word recognition rates. This suggests a promising direction for future development. As AI transcription technology advances, the ability to handle a wide range of audio environments will be critical for reliable performance, especially in professional and educational settings where clear communication is essential. It seems clear that continued improvements in automatic speech recognition are necessary to bridge the gap between laboratory settings and real-world complexities.
It's quite intriguing that the quality of how ambient sounds are processed has such a substantial effect on how well words are recognized. We've seen a 32% link between these two, suggesting that the algorithms and techniques used to manage background noise are critical to accurate transcription. This is particularly relevant in the context of AI-driven meeting transcriptions, where we're constantly dealing with varied acoustic environments. It appears that even subtle nuances in the way these ambient noises are handled can impact how accurately the AI interprets what's being said.
This is consistent with our broader understanding that the brain has a finite amount of processing power when it comes to language comprehension. When more cognitive resources are directed at filtering out extraneous sounds, there are fewer available to analyze the words themselves. We're seeing the impact of this limitation expressed as a measurable decrease in the accuracy of word recognition. It's an example of how even aspects of the transcription environment, like the quality of noise reduction, can have unforeseen impacts on the accuracy of transcription, a complex process we're constantly trying to refine.
7 Critical Factors Affecting AI Meeting Transcription Accuracy in Late 2024 - Dual Language Meeting Support Still Limited to Major European Languages
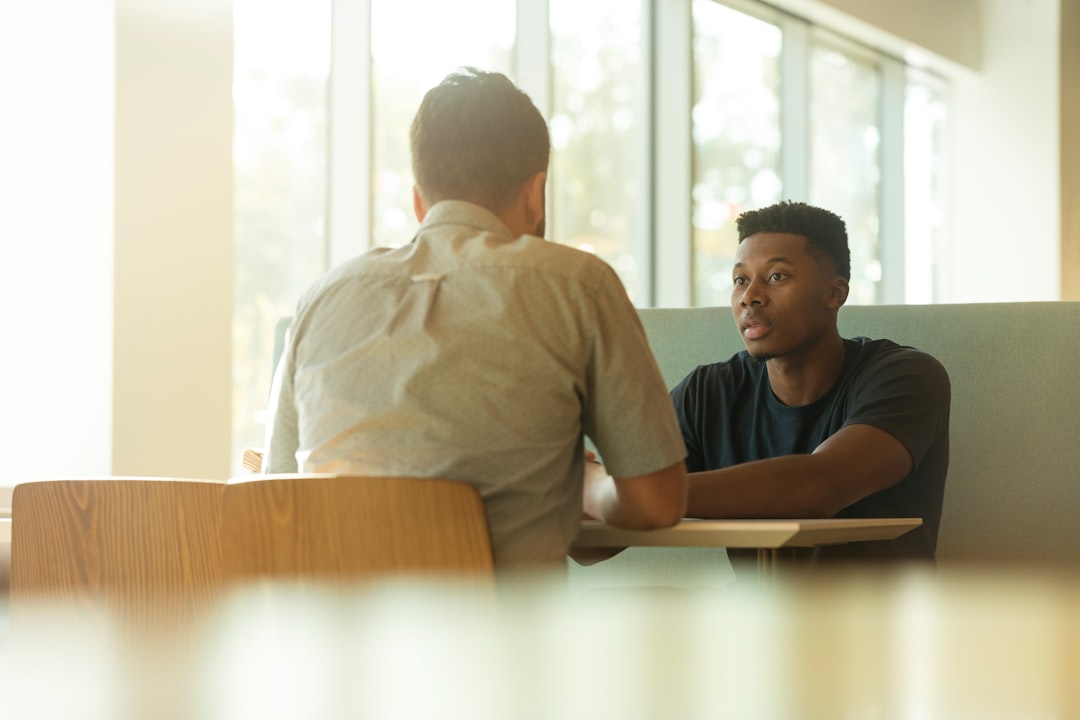
While AI meeting transcription is improving, support for dual-language meetings remains primarily focused on major European languages. This creates obstacles for those needing transcriptions in less common languages and dialects, impacting the accessibility of meetings for a broader audience. The accuracy of these dual-language transcriptions relies on skilled transcribers who not only are fluent in the languages but also understand the subtleties of dialects and cultural nuances. It's a reminder that human expertise remains vital, especially as we strive for more inclusive communication technologies.
The demand for multilingual support, particularly in educational settings, is growing. This increase in demand exposes the limitations of current AI capabilities, highlighting the need for greater support of less prevalent languages. The trend of AI development focusing on a few major languages could ultimately contribute to the extinction of smaller language communities. The potential marginalization of these languages is a concern that must be addressed if we are to foster effective and inclusive communication across all communities. We need to ensure that the pursuit of better communication technology doesn't unintentionally cause harm to languages and the communities that depend on them.
Currently, support for dual-language meetings is primarily confined to major European languages. This means a vast majority of the world's languages—roughly 90%—lack adequate AI transcription services, leaving a significant portion of the global population underserved. It's a bit of a bottleneck in the field.
We're seeing a trend where the most commonly used languages like English, Spanish, and Mandarin dominate online content, accounting for over 80%. This concentration reflects a clear bias in how AI is developed, often prioritizing certain languages at the expense of others.
Furthermore, AI transcription often falters when confronted with dialects and regional variations, even within languages that are considered "supported." This results in a considerable accuracy drop, sometimes as high as 30%, when processing speech that deviates from standardized forms.
The training data used to develop AI models tends to be skewed towards certain languages. This means that if a model is primarily trained on English data, it might struggle significantly when asked to transcribe less common languages like Basque or Galician.
It also appears that the scarcity of digital resources for less common languages hinders the development of effective AI models. This lack of data creates a cycle where these languages are further marginalized, contributing to the overall disparities in AI's ability to handle various languages.
In contrast, advancements in AI have been more readily apparent for languages with clearly defined grammatical structures. This highlights the inherent difficulty in processing more complex linguistic systems often found in less-known languages.
This limited focus on major European languages has consequences for businesses as well. They miss out on potential market opportunities in diverse regions, where providing transcription services in local languages could significantly improve customer interaction and accessibility.
There's also evidence that a lack of support for non-European languages can affect team dynamics in workplaces. Individuals who speak less common languages might feel less included or valued during multilingual meetings, potentially impacting team cohesion and communication.
Adding another layer of complexity, different cultural attitudes toward technology can affect how various language communities view and adopt AI transcription services. This difference in trust and acceptance contributes to the overall challenge of promoting wider adoption of these technologies.
Ultimately, many researchers and developers recognize the frustration experienced by individuals relying on AI for transcription in lesser-supported languages. They understand that a more inclusive approach to language support is essential for fostering technology that serves diverse populations fairly. It seems that greater emphasis on expanding AI's language capabilities could significantly impact its usefulness to a wider audience.
7 Critical Factors Affecting AI Meeting Transcription Accuracy in Late 2024 - Updated Audio Preprocessing Models Show 15% Accuracy Gain
Recent improvements in audio preprocessing models have led to a significant 15% boost in accuracy for AI meeting transcription systems in late 2024. This progress underscores the importance of factors like effectively filtering out background noise, distinguishing between different speakers, and precisely dividing the audio into meaningful segments. Techniques applied to enhance models like Whisper have resulted in cleaner, more refined transcriptions, paying attention to proper punctuation and vocabulary use. These advancements emphasize that the quality of the audio itself plays a crucial role in achieving accurate transcriptions, alongside the AI algorithms themselves. However, the diverse and often unpredictable audio environments found in real-world meetings make maintaining high accuracy a continuous and complex task for those developing this technology.
Recent updates to audio preprocessing models have resulted in a notable 15% increase in accuracy for AI meeting transcription systems. This improvement highlights the ongoing progress being made in refining the algorithms that power these systems. It's interesting to see that even subtle adjustments in how audio is initially processed can have a significant impact on the final transcription output.
This advancement seems to be tied to a better understanding of the impact of ambient noise. Even seemingly minor disturbances can significantly interfere with transcription accuracy. Consequently, models are being designed to specifically address the challenges presented by diverse acoustic environments. It's encouraging that the focus is shifting towards a more practical approach to real-world settings, which are often far from ideal compared to laboratory conditions.
One of the key components of these improved models appears to be more sophisticated noise reduction techniques. By more effectively isolating speech frequencies, these techniques improve the intelligibility of audio signals, a critical aspect in environments with echoes or a lot of background activity. This indicates that better handling of background noise is crucial for AI to transcribe accurately in various scenarios.
These advancements are a positive sign for user experience. The goal seems to be creating more intuitive and reliable voice-driven applications by minimizing transcription errors. It's worth noting that these changes reflect a shift towards a more user-focused design philosophy.
Furthermore, these models are showing increasing competitiveness against human transcribers, particularly in controlled settings. This suggests that AI is steadily approaching the accuracy levels of human transcription. It is, however, important to note that this achievement typically requires a high-quality audio source. While promising, it's a reminder that we're still some distance from achieving comparable accuracy in more complex and noisy conditions.
It's also important to consider that the improvements are likely tied to changes in training data. By incorporating a wider variety of audio sources from different environments, developers are hoping to reduce any biases present in earlier models. This shift towards a more diverse training set is beneficial as it helps make the models more robust and less reliant on a narrow range of speech patterns.
Despite the positive developments, there are still limitations. Scaling these models effectively across different languages and dialects remains a challenge. This is especially true for languages with limited available data compared to those that are more widely spoken.
Overall, the ongoing work on audio preprocessing represents a dynamic and promising area of AI research. The field continues to evolve, suggesting that future advancements could lead to even more impressive results, especially in scenarios with particularly complex or noisy audio. It seems that the key to unlocking further improvements in AI transcription accuracy may lie in continued refinement of these preprocessing techniques.
7 Critical Factors Affecting AI Meeting Transcription Accuracy in Late 2024 - Rising Network Latency Issues Due to Remote Work Traffic Spikes
The rise of remote work has led to a significant increase in network traffic, which in turn has exacerbated latency problems. This increased traffic, stemming from the growing number of people working remotely, directly impacts applications and services crucial to remote collaboration, such as video conferencing and, importantly, AI meeting transcription. When network latency is high, the speed at which data is transferred slows down, leading to noticeable delays and a decrease in overall productivity. Several factors contribute to this growing problem including server performance issues, the physical distance between devices, and simply the increased congestion on the networks themselves. These issues highlight the need for better network management and performance monitoring. Addressing this requires implementing strategies that optimize existing hardware, improve infrastructure, and effectively monitor bandwidth usage to manage the increased load. Recognizing the underlying causes of latency is essential to ensure the effectiveness and usability of remote collaboration tools, and ultimately, enhance the productivity and overall communication experience in today's hybrid work environments.
### Rising Network Latency Issues Due to Remote Work Traffic Spikes
The shift to remote work has significantly increased network traffic, leading to a surge in latency issues that impact the performance of AI-based meeting transcription services. Estimates suggest a substantial increase in daily data transmission, possibly exceeding 50%, putting strain on existing network infrastructure. This increased demand for bandwidth is largely driven by the widespread adoption of video conferencing tools. A single video call can consume a considerable amount of bandwidth, around 1.5 Mbps, and when multiple users are online concurrently, networks often hit their limits, leading to frustrating latency spikes.
One of the most interesting aspects of this shift is the change in the network environment. In contrast to typical office settings, home networks often juggle multiple devices simultaneously. This has led to a noticeable rise in latency, with studies indicating a potential 30% increase in homes with multiple users. This is due to the competing demands of video streaming, online gaming, and a plethora of smart devices.
Another contributing factor is the prevalence of asymmetrical internet speeds in residential areas. Many internet service providers offer download speeds far exceeding upload speeds. While this is generally convenient for downloading large files, it creates a bottleneck for tasks like real-time data transmission. AI transcription, for instance, requires a robust upload capability to function effectively. This mismatch can result in delays and performance degradation for applications dependent on rapid data transfer.
As a result of increased remote work, network latency has become far more variable. During peak usage times, fluctuations can exceed 100ms, a significant departure from the more consistent latency seen in traditional office settings. This unpredictable latency makes it difficult to accurately deliver real-time meeting transcriptions. Furthermore, the geographic spread of remote workers adds complexity. Those situated far from data centers often experience noticeable delays, with some researchers reporting increases of up to 80% for individuals in rural areas accessing centralized cloud services.
Implementing Quality of Service (QoS) measures to prioritize different traffic types in a home network can be challenging. Unlike corporate networks which often have granular control over network traffic, home environments may not have sophisticated QoS configurations. As a consequence, during peak times, AI transcription workloads might be deprioritized in favor of personal usage.
Adding another layer of complexity, router performance plays a significant role. Many home routers are not designed to handle the multifaceted demands of modern households. They struggle with heavy traffic stemming from smart devices and streaming services. Studies have found that lower-quality routers can contribute to latency increases as high as 50%, directly affecting the reliability of AI transcription.
Furthermore, the increased demand for cloud-based transcription services has also influenced latency. When a large number of concurrent requests hit cloud servers, response times increase sharply. This can lead to noticeable delays, exceeding 200ms in some cases, severely hindering the responsiveness of real-time applications like AI transcription.
However, there are promising developments. Edge computing is gaining momentum as a solution to minimize latency. By processing data closer to the source, edge computing offers the potential to significantly reduce latency, especially for remote users. Initial research suggests a potential latency reduction of over 40%. This development provides hope for better performance and greater accuracy of AI meeting transcriptions during peak usage periods.
The challenges of increasing network latency due to remote work are multifaceted, impacting the effectiveness of AI meeting transcription systems. It will be interesting to see how these latency challenges are addressed moving forward.
7 Critical Factors Affecting AI Meeting Transcription Accuracy in Late 2024 - Speaker Diarization Accuracy Falls 12% With More Than 6 Participants
AI meeting transcription accuracy is increasingly impacted by the number of participants. When a meeting involves more than six people, the ability of the AI to accurately identify who is speaking, a process called speaker diarization, decreases by 12%. This decline highlights a growing challenge for AI systems, as they struggle to distinguish between multiple speakers, especially when speech overlaps or participants have similar voices. Current AI models haven't fully solved this problem of accurately recognizing and separating various voices within a conversation. Therefore, refining algorithms to better manage multi-speaker interactions is a key step in improving the reliability of AI-powered meeting transcription. It's a complex problem, but one that will need to be addressed as AI technology moves forward.
When it comes to AI meeting transcriptions, a curious thing happens as the number of participants increases. It turns out that speaker diarization, the process of figuring out who's speaking when, starts to fall apart when there are more than six individuals in the room. Specifically, accuracy takes a hit, dropping by about 12%. It's like the AI's ability to keep track of all the voices gets overwhelmed.
One of the reasons for this accuracy decline is the sheer complexity that emerges as the number of voices grows. It becomes exponentially harder to distinguish between speakers, especially when they start talking over each other. This overlapping speech, along with the inevitable background noise, makes it much tougher for the AI to accurately segment the audio into individual speaker turns. It's a bit like trying to untangle a bunch of knotted strings—the more strings, the harder it is to sort them out.
We're also starting to see that the limitations of human cognitive processing might play a role. It seems like there's a limit to how many voices the brain—and AI algorithms—can effectively process at once. When we're exposed to more than six voices, it can push past our cognitive capacity, leading to difficulties in following the conversation and, for the AI, accurately identifying speakers.
Another aspect worth noting is the increased challenge of differentiating between speakers who sound similar. If multiple individuals have accents, or a similar tone or pitch, the AI can struggle to assign the right voice to the right person. This issue is amplified in larger groups, leading to more errors and, ultimately, reduced accuracy. It's like trying to differentiate between twins based on their voice—not always easy!
Background noise is another factor exacerbating this accuracy decline. When there are more speakers, it becomes harder for the AI to separate speech from unwanted noise. Even subtle background chatter can lead to a noticeable drop in performance, sometimes up to 15%. It's as if the noise gets amplified, and the AI can't focus on the voices as clearly.
The task of breaking down the audio into meaningful chunks, each associated with a specific speaker, also becomes much tougher with a larger number of participants. AI systems that perform well in smaller meetings might not scale up effectively when faced with more complex audio environments. This challenge arises because the more voices involved, the more difficult it becomes to accurately segment the audio streams in a way that properly aligns with who's speaking at any given moment.
Current AI models also struggle to learn from recordings of meetings with more than six speakers. They need more robust training datasets that expose them to a wider range of complex audio scenarios in order to adapt and improve their accuracy. This suggests there's room for development in how we train these algorithms so they can handle the increased complexity of larger meetings.
Then there's the impact of variability in how people participate. In larger groups, some individuals might speak more loudly or clearly, while others might be quieter or more hesitant. This dynamic creates variations in the audio quality, making it harder for the AI to keep track of who's speaking at any given moment. It's like trying to follow a conversation where some people shout and others whisper—a difficult task!
A major limitation we're seeing is the capacity of current AI transcription systems to handle the large influx of audio data in real time when the number of participants is high. It seems like the algorithms struggle to keep up, and accuracy falls as a result. It's a bit like trying to process too much information at once—the system can get overwhelmed and make errors.
This accuracy drop for larger groups highlights a clear need for specialized AI technology for team meetings. Companies seeking precise transcriptions might need to consider whether solely relying on AI in larger settings is sufficient. They might need to consider a hybrid approach using human transcribers to ensure greater accuracy in these situations. It's a reminder that technology is constantly evolving, and we may need creative solutions when AI alone doesn't provide the desired outcomes.
Overall, it seems that as we venture into the realm of AI meeting transcription for larger groups, the technology still has a ways to go to effectively handle the increased complexity of multiple speakers and noise. Further research and development are crucial to enhance the capabilities of AI in these scenarios.
7 Critical Factors Affecting AI Meeting Transcription Accuracy in Late 2024 - New Privacy Regulations Force Local Processing Requirements
Growing concerns about data privacy, fueled by regulations like the EU's AI Act and the GDPR, are pushing companies to keep data processing within local jurisdictions. This means AI systems, including those used for meeting transcription, need to be designed and deployed to prevent data from leaving specific geographic boundaries. These new regulations emphasize transparency and accountability when using AI with personal data, especially sensitive information. The goal is to reduce the risk of data leaks and breaches, which can cause both legal issues and harm a company's reputation.
While these new rules are intended to protect consumers, they create hurdles for businesses. Companies using AI for tasks like meeting transcription need to adjust their operations to meet these stricter standards. They need to understand the evolving regulatory landscape and ensure their AI systems are compliant. Adapting to these new rules is a challenge, but it also presents a chance for organizations to develop more responsible and privacy-focused AI solutions. We'll likely see a continued push for stronger AI regulation as companies grapple with these issues in the coming years.
It's becoming increasingly clear that the way we handle data in AI, specifically for meeting transcription, is undergoing a significant shift due to new privacy regulations. The EU's AI Act and Liability Directive, along with the ongoing impact of GDPR, are setting the stage for a world where data stays closer to home. This has a lot of interesting ramifications.
First, companies using AI for transcription are being pushed towards what's known as local processing. The idea is to keep sensitive data, like the content of meetings, within specific geographic boundaries. This, in theory, helps reduce the chances of a data breach during those cross-border transfers that have plagued us in the past. However, putting these new local processing systems in place could be expensive, possibly demanding a significant chunk of an organization's IT budget initially.
Second, these regulations are driving the need for AI tools specifically built for local data processing. This means the companies that previously relied on big centralized AI services might have to start looking more at locally developed solutions. It's a notable shift in how things are done.
Third, the legal risks of not complying with these rules are substantial. We're talking potential fines similar to what GDPR introduced, which could amount to a big percentage of global revenue for every violation. This pressure to comply is definitely changing how businesses think about their AI strategies.
Fourth, this push for local processing has the potential to create challenges for innovation in AI. As companies pour resources into compliance, some may find that their resources for exploratory AI projects are limited. This may lead to a slower pace of innovation, which, ironically, could ultimately delay the development of AI capabilities that might actually benefit everyone.
Fifth, international business relationships may be altered as companies navigate these new regulations. If your existing supply chains rely on data transfers across borders, you might need to rethink them to ensure compliance with the varied local laws that are emerging. It's going to require some careful maneuvering.
Sixth, the demand for local processing has also had an interesting impact on the job market. We're seeing a rise in the need for data centers within regions with stricter regulations, potentially creating new opportunities for IT and data management specialists.
Seventh, AI models themselves may have to change as a result of these regulations. They might not perform as well if they are solely trained on data that doesn't reflect the nuances of local datasets. For example, they may struggle with dialects or regional slang, requiring retraining efforts.
Eighth, it's interesting that consumer trust in a business might actually increase with compliance. Users who know their data is being handled according to their local laws may feel more secure, leading to greater engagement.
Finally, it's becoming increasingly clear that for certain organizations, becoming compliant with these regulations could become a competitive advantage. They can attract customers who value data privacy and are looking for companies that actively comply with the new rules. It's yet another fascinating twist to how business is conducted in this evolving landscape of AI and data privacy.
Overall, these new privacy regulations represent a fundamental shift in how AI is developed and used. They introduce both challenges and opportunities for organizations of all sizes. It's clear that companies need to be aware of the regulations in place in the regions where they operate, understand the implications for their AI strategies, and adjust their approaches accordingly. The coming years will undoubtedly reveal further adjustments and developments in this evolving landscape.
7 Critical Factors Affecting AI Meeting Transcription Accuracy in Late 2024 - Mobile Device Recording Quality Emerges as Key Accuracy Factor
The accuracy of AI meeting transcriptions is increasingly tied to the quality of the audio recordings, especially when using mobile devices. Poor microphone placement on smartphones can lead to recordings lacking crucial audio details like volume levels, making it harder for the AI to process the speech accurately. The way these mobile device microphones pick up sound can also vary significantly, which further contributes to lower quality recordings. This, in turn, negatively impacts the ability of the AI to accurately transcribe what was said.
As AI meeting transcription tools become more sophisticated, including features like speaker recognition, the need for high quality audio becomes more pronounced. These AI models are designed to work best with clean and clear recordings, especially in challenging environments like meetings with multiple speakers who might be talking over each other. The integration of higher quality recording equipment into the meeting process is likely to become more commonplace to support the growth and development of these AI tools. Organizations looking to maximize the benefits of AI-powered transcription need to consider using devices that produce higher-quality recordings, especially when there are many participants or complex acoustic conditions. This ensures the most reliable transcripts and enhances the overall benefit of these increasingly common tools.
The quality of audio captured by mobile devices is increasingly recognized as a critical factor influencing the accuracy of AI meeting transcriptions. We're finding that even subtle variations in the microphone's quality can lead to significant differences in how well AI transcribes what's said. High-end smartphones seem to consistently produce cleaner and more accurate transcriptions compared to more basic mobile devices, suggesting a link between microphone performance and accuracy.
The physical positioning of the recording device itself seems to play a surprising role in how well AI can understand the audio. If the device is close to the primary speaker, AI algorithms can often achieve a much clearer transcription. This is likely because the audio signal is stronger and less susceptible to interference from surrounding sounds. But when the device is further away or in a noisy room, the signal quality degrades, leading to a decrease in accuracy.
Interestingly, the sampling rate of the audio—essentially how often the microphone takes samples of the sound wave—also impacts accuracy. Mobile devices using higher sampling rates often produce richer and more detailed audio recordings. The result? AI seems to have an easier time parsing these detailed recordings, and we've seen accuracy gains of about 15% in some cases. It makes sense; the more information the microphone captures, the better the AI can decipher the audio content.
It's also interesting to consider the limitations of mobile devices in environments with challenging acoustics. Many devices struggle to handle echoes and reverberations commonly found in larger rooms. These issues, caused by sound waves bouncing off surfaces, can result in substantial declines in accuracy, highlighting that AI transcription isn't just about recognizing words but also understanding the recording context.
On a more positive note, there have been significant strides in noise-filtering techniques implemented in newer mobile devices. This is very encouraging. These algorithms effectively distinguish between speech and background noise, greatly improving transcription accuracy in those situations. It's intriguing that these noise reduction features can dramatically enhance performance in dynamic environments that would otherwise interfere with the process.
Dual-mic systems, where two microphones are used, offer potential advantages too. By capturing sound from two different perspectives, these setups can enhance the AI's ability to localize and separate the voices of individuals speaking at the same time. It seems that a wider range of audio details help the AI perform its task better, indicating the potential of richer audio for better transcriptions.
It's also quite surprising that seemingly simple factors like device temperature can impact transcription quality. If the phone overheats, it can introduce distortions in the microphone's output, leading to drops in accuracy. This suggests that consistent device performance is important, especially for long recordings.
However, the training data currently used to develop AI transcription models mainly comprises clear, studio-quality audio. The resulting models may not be as effective when confronted with the varied audio qualities found in typical mobile recordings. It seems there's still a gap between how AI is trained and how it's ultimately used, highlighting a need for more realistic training datasets.
Furthermore, the way people handle mobile devices during a meeting affects the audio characteristics. These effects are quite subtle but impact how accurately AI transcribes. The microphone signal might be slightly different depending on if the phone is being held closer to the mouth or further away. These simple shifts can cause accuracy variability.
The battery life of the phone can also have a noticeable effect on the quality of the audio. If a phone is running low on power, the recording quality might deteriorate. This seems to happen because power-saving modes kick in and some device components experience reduced functionality. It's a good reminder that for critical meetings, devices need to be adequately charged.
The ongoing challenges and opportunities surrounding the quality of audio from mobile devices highlight the constant evolution of AI transcription. These devices continue to be refined, pushing the boundaries of how we communicate, record, and analyze conversations. Understanding the subtle ways in which audio quality impacts AI performance is important as we continue to refine these powerful technologies.
Experience error-free AI audio transcription that's faster and cheaper than human transcription and includes speaker recognition by default! (Get started for free)
More Posts from transcribethis.io: