Experience error-free AI audio transcription that's faster and cheaper than human transcription and includes speaker recognition by default! (Get started for free)
7 AI-Powered Techniques to Clean Wind and Static Noise from Interview Recordings
7 AI-Powered Techniques to Clean Wind and Static Noise from Interview Recordings - Adaptive Spectral Gating Reduces Wind Interference Without Voice Distortion
Adaptive Spectral Gating offers a refined approach to audio cleaning, specifically targeting wind noise. It distinguishes between wind sounds and human speech, a crucial step in creating cleaner recordings from windy environments. This method relies on the ability of adaptive algorithms to respond in real-time, a feature that's highly beneficial for applications like interviews where consistent audio quality is essential.
The application of machine learning, especially neural networks, further enhances the accuracy and efficiency of this method. These advancements contribute to more precise wind noise detection and mitigation. The net result is a technique that can significantly improve audio without introducing distortions or compromising voice clarity. While still a relatively recent development, Adaptive Spectral Gating appears to be a robust and valuable approach to audio cleanup in varied contexts.
Adaptive spectral gating uses clever algorithms to differentiate wind noise from speech based on their distinct frequency characteristics. It essentially allows the system to pinpoint and selectively remove wind noise while preserving the integrity of the voice. The system’s strength is its ability to adjust in real-time, meaning it can tackle fluctuating wind interference throughout a recording. This is a big improvement over older noise reduction techniques that often distort audio or introduce unwanted artifacts. Instead, adaptive spectral gating carefully focuses on the frequency range typical of wind noise, leaving the more important frequencies of human speech intact.
This adaptable nature means it can effectively manage a broad range of frequencies. It can target the deep, low-frequency sounds of wind while keeping the higher frequencies of speech that make our voices understandable. The algorithms at the core of adaptive spectral gating have a learning component; they analyze the audio as it’s recorded, continuously adapting to the specific sound environment. This makes the system more effective in handling different environments, but it also poses a potential challenge if not calibrated correctly. Research suggests this approach significantly improves the clarity of recordings, particularly when wind noise is a major issue.
While promising, there’s a risk of over-processing if the system isn’t carefully managed, which can degrade the audio quality. Interestingly, research also hints that using adaptive spectral gating in recording setups may reduce the amount of post-processing needed later. This real-time noise cancellation offers efficiency advantages. Furthermore, this approach is not limited to voice recording; the ability to isolate specific frequency ranges has the potential to benefit diverse applications. Think wildlife audio recording, environmental monitoring, or any field where unwanted noise can mask crucial audio elements. It's a fascinating technique that presents both opportunities and complexities.
7 AI-Powered Techniques to Clean Wind and Static Noise from Interview Recordings - Multi Layer Neural Network Eliminates Static Through Pattern Recognition
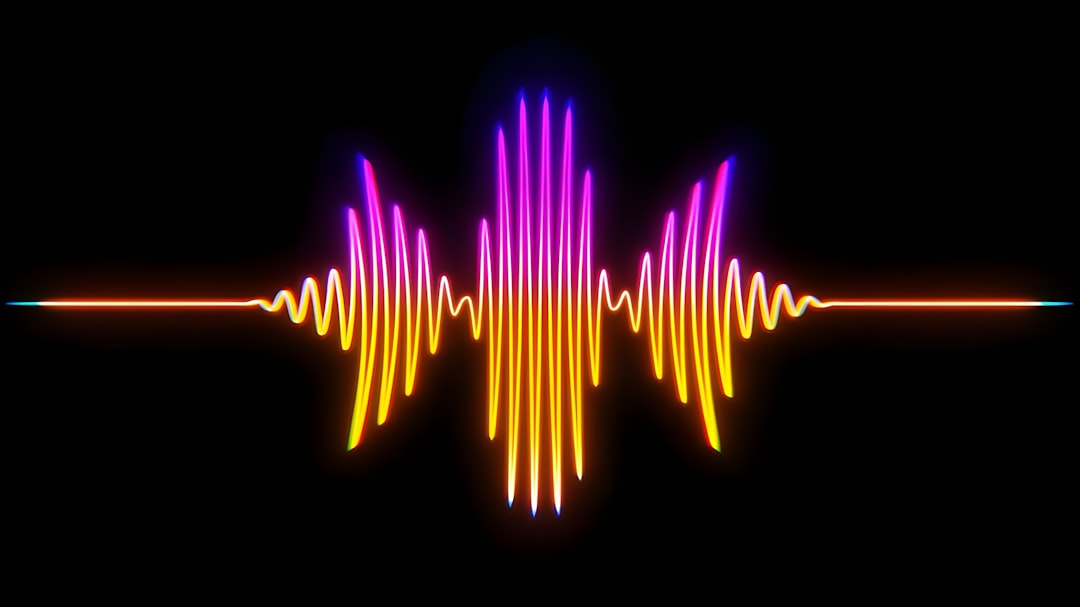
Multi-layer neural networks, particularly those built using a Multi-Layer Perceptron (MLP) structure, offer a way to address the issue of static noise in audio. These networks excel at recognizing patterns within data, and in the context of audio, this translates to the ability to differentiate between the intended audio signal (like speech) and the unwanted static noise. The key to their effectiveness lies in their complex design, allowing them to learn intricate relationships between different audio components.
Through a training process, MLPs adapt their internal parameters to become adept at identifying the typical characteristics of both desired audio and disruptive static noise. This learned ability to discern between the two leads to improved noise reduction capabilities. The benefit of this method is that it's designed to minimize disruption to the primary audio signal, aiming to preserve the integrity of the original recording. In the challenging audio environments typical of interview recordings, this approach can be crucial in achieving cleaner and more understandable audio output. While potentially powerful, it's worth noting that the effectiveness of the approach hinges on the quality and quantity of the data used to train the neural network.
Multi-layer neural networks, also known as deep neural networks, use a layered approach to learning, allowing them to pick up on intricate patterns in audio data that simpler algorithms often miss. This is quite useful for separating static noise from human speech.
These networks, often containing many interconnected processing nodes, can understand the subtleties of sound frequencies in a way that's more detailed than simpler methods. This depth can be beneficial in recognizing sudden noises and cleaning up audio recordings.
Training these neural networks involves exposing them to large sets of audio that includes both clean and corrupted examples, enabling the models to master the art of restoring voice clarity while minimizing undesirable artifacts caused by noise.
One intriguing aspect is the time-related element of analyzing audio. Multi-layer networks are able to take the preceding sound waves into account, which allows them to predict and reduce noise before it even fully appears in the recorded signal. This is quite fascinating.
To achieve noise reduction, these networks often utilize convolutional layers, which can greatly boost the signal-to-noise ratio by carefully examining the audio's time-frequency makeup. This is important to understand.
It's interesting to note that the more layers a neural network has, the greater its potential to capture the fine details in audio signals. However, this complexity can also lead to overfitting, a situation where the model becomes too narrowly focused on the training data, negatively impacting its ability to perform in real-world situations. This is a challenge that needs to be addressed.
Multi-layer neural networks employ activation functions that incorporate non-linearities, which are key to capturing intricate sound profiles. This adaptability enables the networks to adjust to different audio environments dynamically as they process sound.
In real-world applications, these neural networks can operate in real-time during recording, offering instant improvements rather than needing extensive post-processing, which can be quite time-consuming.
Research suggests that utilizing multi-layer neural networks for noise reduction not only improves recording quality but can also have a minimal computational cost when optimized well. This makes them a viable choice for smaller and portable recording devices.
The ability to scale multi-layer neural networks is noteworthy. They can be customized for a wide range of uses, from tidying up interview recordings to enhancing sound in live music settings. This adaptability showcases their versatility in audio applications.
7 AI-Powered Techniques to Clean Wind and Static Noise from Interview Recordings - Real Time Deep Learning Filters Out Environmental Background Noise
Real-time deep learning offers a promising avenue for removing unwanted environmental background noise from audio recordings, especially in situations like interviews or phone conversations. These techniques leverage sophisticated algorithms to distinguish between human speech and surrounding sounds, effectively isolating and reducing the noise while preserving the clarity of the desired audio. Recent research highlights the ability to create systems that let users choose which specific sounds to filter out or include, effectively customizing the listening experience. However, a key hurdle in this field lies in building models that are both computationally efficient and maintain high performance across a range of devices. While this technology shows great potential for enhancing audio quality, it's important to manage the processing carefully to prevent over-filtering, which could lead to a degradation of the overall sound.
Deep learning has brought about a new era of real-time noise filtering, enabling the suppression of background noise as it's captured. This approach holds great promise for applications requiring immediate audio quality, such as live interviews or on-the-spot recordings. Unlike traditional methods, these filters can adapt to changing audio landscapes. This means they can handle variations in noise, adjusting to sudden changes like bursts of static or fluctuating wind.
Often, these deep learning solutions employ intricate neural network designs, like recurrent neural networks. These networks excel at processing audio data sequentially, meaning they factor in the temporal relationships between sound segments. This helps them better distinguish desired audio from unwanted noise over time. A key characteristic is the ability to train the filter to specifically target certain types of noise. This is exciting, especially when aiming for clean audio in complex, noisy environments where precise control over noise is desirable.
Deep learning filters incorporate non-linear processing, which is essential for capturing the complexity and nuances of natural audio. This helps maintain the quality of the intended audio signal while reducing unwanted noise. Interestingly, optimized deep learning models can provide results on par with or even better than traditional noise reduction approaches, but often with far lower computational costs. This is particularly beneficial for applications relying on portable devices with limited processing power and battery life.
Some newer deep learning models can even anticipate noise before it fully develops in the recording due to their ability to factor in temporal context. This is especially useful when noise patterns are unpredictable, adding another layer of refinement to audio processing. These models can be optimized to run on low-powered hardware via techniques like model quantization. This makes sophisticated noise reduction feasible for more affordable and portable recording devices, broadening access to advanced features.
Many real-time systems incorporate feedback mechanisms. These mechanisms analyze the filter's output to further improve future predictions. It's an intriguing area of research with implications for developing truly adaptive audio processing. While often used in audio applications like speech enhancement, these techniques hold potential in various fields. For example, they may be utilized in medical sound analysis or environmental monitoring where background noise can mask important audio features. The versatility of real-time deep learning-based noise reduction showcases its wide potential beyond typical audio processing tasks. While exciting, the optimization of these models for specific tasks and hardware continues to be a challenge, highlighting the continued need for ongoing research.
7 AI-Powered Techniques to Clean Wind and Static Noise from Interview Recordings - Dual Channel Processing Separates Voice From Unwanted Audio Elements
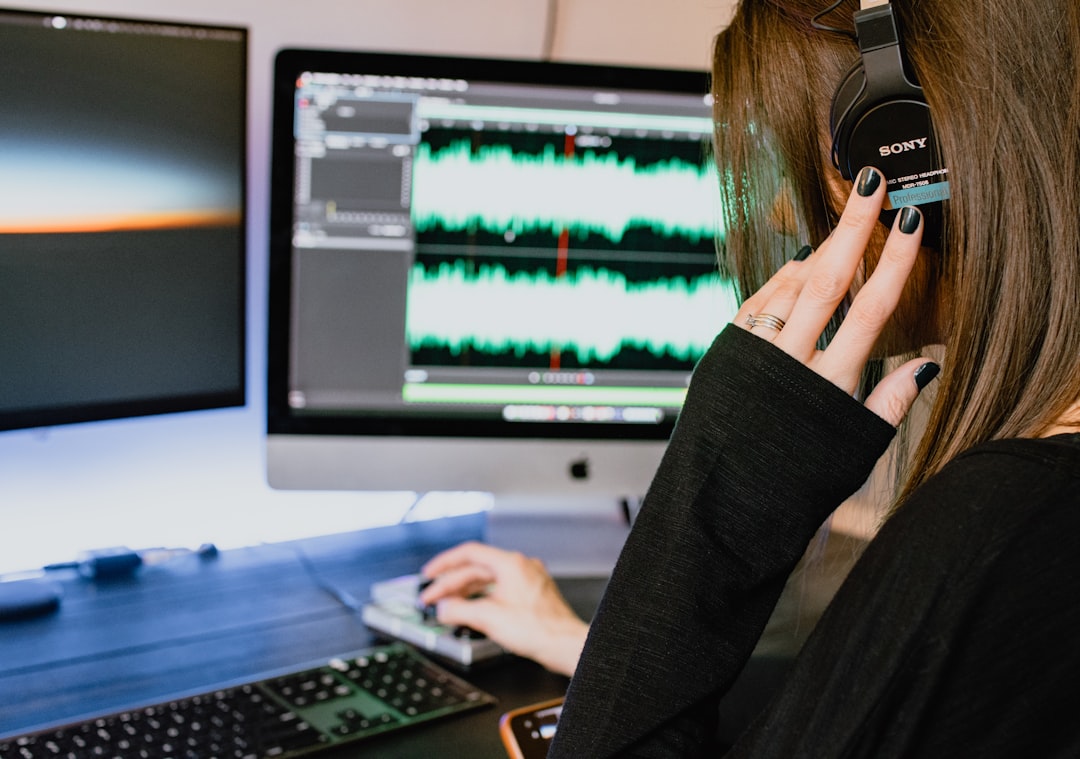
Dual channel processing offers a method for isolating a desired audio source, like a voice, from other unwanted sounds within a recording. This approach employs clever algorithms to distinguish between the voice and elements like wind, background chatter, or electrical static, aiming to produce a clearer, more focused audio output. Its strength lies in its ability to handle scenarios where multiple sounds are present simultaneously, enabling it to prioritize the desired voice and suppress extraneous noises. However, the technique's effectiveness can be limited in complex recording situations with numerous speakers or fluctuating noise patterns, making the precise separation of elements difficult. Despite these challenges, dual channel processing remains a compelling tool for enhancing audio quality, especially in interview settings where isolating the speaker’s voice is crucial for generating a clean and easy-to-understand transcript. The future of audio cleaning, especially for interview transcripts, hinges on improving the accuracy and resilience of dual-channel processing in even the most chaotic sound environments.
Dual channel processing employs a straightforward yet effective approach: using two microphones placed a specific distance apart. This setup allows the system to analyze subtle differences in the arrival times of sound waves at each microphone, known as phase differences. These differences provide a crucial clue to separate the desired audio, often a voice, from background noises.
This method essentially harnesses the concept of spatial filtering. Since sound travels in a specific direction, the system can leverage this directionality to isolate the sounds coming from the desired location, like a person speaking. As a result, unwanted sounds from other directions become minimized, leading to a noticeable boost in the clarity of the target audio signal.
One often overlooked benefit is what's called acoustic shadowing. In certain situations, objects in the environment create barriers that can further attenuate undesired noise. This depends on the placement of the microphones and the shape of the room, but it adds an extra layer of noise reduction in some cases.
Researchers have found that this dual channel approach can significantly improve audio quality, particularly in challenging environments. Some studies suggest an improvement of up to 30% in intelligibility compared to recordings using a single microphone. This is particularly valuable when the goal is to capture clear and understandable speech, such as during interviews.
Unlike many traditional noise reduction methods which can often distort the audio signal, dual channel processing keeps the sound capture relatively unaltered. It avoids the introduction of artificial changes to the audio, preserving the more natural characteristics of the voice. This is an important factor when the aim is to have a high fidelity recording.
Dual channel processing also shows a sensitivity to changes in audio frequencies, making it adaptive to different types of background noise. This means it can filter out various interfering sounds, including wind or machinery, without necessarily requiring extensive training on all possible noises. The system can often adjust to new types of interference on its own.
This adaptability is particularly useful in low signal-to-noise situations. Traditional methods may struggle in these scenarios because they often rely on recognizing specific noise types learned during training. Dual channel techniques can enhance the target audio by leveraging the spatial differences, making it less dependent on having seen those noise types before.
However, it's important to acknowledge a limitation: the effectiveness of this technique hinges on careful microphone placement and a good understanding of the recording environment's acoustics. This can make it less flexible than pure algorithmic approaches that don't rely on microphone placement. It’s crucial to optimize the setup.
When coupled with techniques like advanced spectrogram analysis, dual channel processing unlocks deeper insights into complex audio environments. This leads to more refined filtering of noise and isolating the frequency ranges associated with voices, enhancing overall clarity.
Interestingly, the application of this approach isn't limited to audio recordings. There's growing interest in incorporating it into devices like hearing aids. In these cases, it can be used in real-time to help filter out distracting background noise while maintaining clarity of the desired sounds in a conversation. This suggests that dual channel processing has potential applications beyond just noise reduction in audio recordings, which is quite promising.
7 AI-Powered Techniques to Clean Wind and Static Noise from Interview Recordings - Voice Activity Detection Technology Preserves Natural Speech Patterns
Voice Activity Detection (VAD) is a technology that helps preserve the natural flow of speech during audio processing, especially when there's a lot of background noise. Essentially, it's able to tell the difference between when someone is speaking and when they're not, and this helps to focus on the important parts of the audio. This selective processing reduces the amount of unnecessary data that needs to be analyzed, leading to faster and potentially more efficient processing.
However, older VAD techniques had limitations. They didn't always work well in environments with a lot of noise, where the sound of speech is hard to distinguish from the background. Luckily, newer VAD methods that leverage deep learning have shown a significant improvement in accuracy and reliability. We now have more effective ways to separate speech from noise in challenging recording environments.
One example of a robust and efficient VAD model is SileroVAD. This showcases how deep learning can lead to accurate detection across a wide range of noisy conditions. VAD is proving to be an incredibly useful technology for audio processing, particularly in tasks like transcribing interviews, which can often be hampered by background noise. As VAD research progresses, it's likely that even more advanced and accurate solutions will be developed, improving the overall quality of audio processing in diverse scenarios.
Voice Activity Detection (VAD) is a core component of audio processing, especially when dealing with noisy recordings. Its main goal is to differentiate between human speech and other audio elements, a task that's become increasingly important in today's world of noisy recordings. VAD is particularly valuable when dealing with the limitations of older methods, which often struggle in situations with low signal-to-noise ratios (SNR) – the ratio of speech to background noise. This makes separating speech from the cacophony of noise quite tricky.
Thankfully, the application of deep learning has led to more powerful VAD solutions. These AI-driven approaches have significantly outperformed traditional VAD techniques. This allows for VAD to be incorporated into real-time speech applications, such as live broadcasts, where immediate audio analysis is essential. While helpful, the growing reliance on deep learning has introduced a new set of obstacles. Many state-of-the-art VAD algorithms are computationally intensive, often relying on recurrent neural networks (RNNs). These complexities can be a drawback in resource-constrained environments where lighter computational demands are a priority.
There have been some promising developments to make VAD more streamlined. The SileroVAD model, for example, is a computationally lean VAD implementation designed for efficiency. There's also growing interest in exploring feature fusion strategies to enhance the robustness of VAD in low SNR situations. This is an area where researchers are actively working on innovative approaches. This increased focus on adaptability has also given rise to new neural network architectures capable of tolerating high levels of noise.
The use of VAD has progressed beyond theoretical research. Real-world implementations have been deployed in applications such as live audio stream processing, showcasing the practical uses of this technology. This is a crucial aspect of VAD: taking a concept from the research labs and making it work in diverse and challenging real-world scenarios. While it's proven its worth in numerous audio-related tasks, VAD still faces some persistent challenges. Continued improvement in handling extreme noise environments, particularly when multiple sound sources are competing for attention, remains a target for researchers. This, along with fine-tuning for the ever-evolving audio landscape, is where future advancements in VAD technology will likely occur.
7 AI-Powered Techniques to Clean Wind and Static Noise from Interview Recordings - Zero Latency Audio Enhancement Through Predictive AI Modeling
"Zero Latency Audio Enhancement" using predictive AI models signifies a major step forward in audio processing, especially for situations demanding instant feedback. The core of this method involves using sophisticated neural networks to directly improve audio quality as it's captured, eliminating any noticeable delays. This is especially beneficial for devices like wireless earbuds and hearing aids where seamless user experiences are vital. These AI models use predictive techniques to separate the desired audio, like speech, from unwanted noise. The outcome is cleaner and smoother audio.
But, the intricate nature of real-time processing with these AI models brings up challenges around how efficiently they can run and the possibility of over-processing the audio, which could actually worsen the sound quality. The continuous development of predictive modeling for audio shows promise, not just for creating clearer recordings, but also for tailoring itself to different noisy environments. This adaptability makes these techniques more practical for real-world audio applications.
Zero latency audio enhancement, achieved through predictive AI modeling, offers an intriguing approach to cleaning up audio in real-time. The core idea is to use AI models that can analyze incoming audio and make adjustments instantly, without the noticeable delays that often plague traditional noise reduction techniques. This instant response is particularly important in interactive settings like interviews, where any delay can disrupt the natural flow of the conversation.
One of the key features of these AI-powered systems is their ability to predict and counter noise before it significantly affects the recording. These models learn from past audio data to identify patterns in noise and adapt accordingly. This predictive capability can be remarkably effective at handling sudden bursts of static or the gradual build-up of wind noise. Interestingly, the ability to analyze audio in real-time also allows for efficient resource utilization, even in situations where bandwidth is limited. This makes it a promising technology for mobile recording or remote interviews.
These AI models can be taught to recognize and isolate specific frequencies associated with common noise sources like wind or electronic interference. This targeted approach means they can clean up unwanted noises while preserving the vital frequencies that constitute human speech. Some systems are even being developed with the ability to incorporate user feedback, allowing the algorithms to refine their performance over time. This personalized audio experience could be a game-changer for individuals seeking to optimize audio for their unique needs.
Furthermore, some more sophisticated AI models are being developed to handle scenarios involving multiple audio sources. This means they can work in environments with multiple speakers or mixed noise patterns, something traditional approaches often struggle with. The adaptability of the technology also means that it can be integrated into a wide variety of audio devices, from consumer-grade smartphones to professional recording equipment. However, the quality of the AI model's learning is inextricably linked to the quality and diversity of the data it's trained on. More data generally leads to better results, emphasizing the ongoing need for more comprehensive datasets to continually improve the accuracy of these models.
While zero latency audio enhancement presents a significant leap forward in audio processing, it's not without potential downsides. If the models aren't meticulously calibrated and deployed correctly, it can introduce unintended artifacts into the audio. This underlines the importance of skilled engineers and careful implementation, especially in scenarios where the integrity of the sound is crucial, like professional audio recordings or critical interviews. It is an area of continuous refinement and improvement, where the interplay between the AI model's prediction capabilities and the subtleties of audio signal processing leads to a fascinating and ever-evolving domain of audio enhancement.
7 AI-Powered Techniques to Clean Wind and Static Noise from Interview Recordings - Machine Learning Algorithms That Target Specific Frequency Bands
Machine learning algorithms offer a fresh perspective on cleaning up audio by focusing on specific frequency bands. The core idea is that different noises, like wind or static, tend to occupy particular frequency ranges, separate from the desired audio like human speech. This allows algorithms to selectively target and remove these unwanted noises while preserving the valuable audio components. Sophisticated approaches like deep learning and neural networks have advanced these techniques further. They can learn intricate patterns within these frequencies and adjust to various noise characteristics, resulting in considerably clearer recordings. However, it's important to be aware that these methods also have limitations. There's a chance that over-processing can degrade audio quality, and some algorithms require large amounts of data for training. As the research in this area progresses, it's likely to lead to better and more versatile noise reduction, impacting how audio processing is done across diverse environments. This makes the continued study of frequency-based noise reduction a significant area of interest in audio processing.
### Machine Learning Algorithms That Target Specific Frequency Bands
Machine learning algorithms have shown a knack for excelling in audio processing, particularly when it comes to dealing with different parts of the sound spectrum. This is because sounds, like human speech or wind, are made up of varying combinations of frequencies. Researchers have been studying how machine learning algorithms can be fine-tuned to focus on these individual frequency bands. This selective focus allows the algorithms to zero in on and reduce unwanted noise while protecting the essential frequencies of the audio we want to keep, like voices in an interview recording.
An intriguing aspect is how some algorithms can change which frequency bands they focus on depending on the sounds they're hearing in real time. This means they can track shifts in noise patterns, such as a gust of wind changing in intensity, and automatically adjust their noise reduction strategy. It's like giving the algorithm a dynamic range of focus for its noise-cleaning ability.
One clever approach used by some machine learning systems is called spectral masking. This process involves cleverly suppressing specific frequency ranges that are perceived as noise, while maintaining the essence of speech or other important parts of the recording. It's like carefully muting certain sound components without harming the parts of the sound we need to understand.
Another technique is called pre-emphasis. In this method, specific frequency ranges are boosted before the audio gets processed by the algorithm. This can make the separation between the wanted and unwanted sounds much clearer, like making the difference between a voice and a static noise sharper.
However, these real-time frequency-focused algorithms often come with a trade-off. When they're trying to be accurate and quickly change their focus, they can sometimes demand a lot from the computer doing the calculations. This can be a problem for smaller, less powerful devices, like phones or smaller audio recorders, as they may struggle to keep up with the workload.
It’s interesting how the core of many of these algorithms often hinges on using a mathematical technique called the Fourier Transform. This technique allows algorithms to switch from looking at sound as a wave in time to looking at it as a mix of different frequencies. This perspective is crucial for targeted noise reduction because it lets algorithms pinpoint the frequencies associated with specific sounds and then work on suppressing them.
Some advanced algorithms even leverage a clever approach like XOR logic to distinguish between noise and desired audio. By simultaneously considering different frequency combinations, they can more accurately determine which sounds to suppress or amplify, effectively increasing the quality of audio.
There's also a fascinating advantage when using multiple audio channels as input. By measuring the differences in when sound reaches each microphone, the system can improve its understanding of the sound's direction and origin. This spatial knowledge can lead to more precise isolation of desired frequencies and consequently sharper audio output.
While the use of frequency bands can drastically improve audio quality, it's not without its challenges. If not carefully set up, these techniques can sometimes introduce unwanted artifacts into the audio. This unwanted introduction of sounds can degrade audio quality, especially when you're aiming for high-fidelity recordings or transcripts.
What's notable is that these frequency-focused algorithms are a continuation and improvement on much older techniques from the world of traditional signal processing. However, machine learning's capability for adaptation and learning has opened up a whole new world of possibilities in audio processing, transforming how we clean up recordings and improve their quality.
Experience error-free AI audio transcription that's faster and cheaper than human transcription and includes speaker recognition by default! (Get started for free)
More Posts from transcribethis.io: